University certificate
The world's largest school of business”
Description
A university program with which you will become the most outstanding Clinical Researcher in your environment. You will lead projects that will contribute to the advancement of Medicine!”
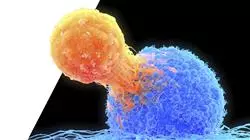
Why study at TECH?
TECH is the world's largest 100% online business school. It is an elite business school, with a model based on the highest academic standards. A world-class centre for intensive managerial skills training.
TECH is a university at the forefront of technology, and puts all its resources at the student's disposal to help them achieve entrepreneurial success"
At TECH Global University
|
Innovation |
The university offers an online learning model that combines the latest educational technology with the most rigorous teaching methods. A unique method with the highest international recognition that will provide students with the keys to develop in a rapidly-evolving world, where innovation must be every entrepreneur’s focus.
"Microsoft Europe Success Story", for integrating the innovative, interactive multi-video system.
|
The Highest Standards |
Admissions criteria at TECH are not economic. Students don't need to make a large investment to study at this university. However, in order to obtain a qualification from TECH, the student's intelligence and ability will be tested to their limits. The institution's academic standards are exceptionally high...
95% of TECH students successfully complete their studies.
|
Networking |
Professionals from countries all over the world attend TECH, allowing students to establish a large network of contacts that may prove useful to them in the future.
100,000+ executives trained each year, 200+ different nationalities.
|
Empowerment |
Students will grow hand in hand with the best companies and highly regarded and influential professionals. TECH has developed strategic partnerships and a valuable network of contacts with major economic players in 7 continents.
500+ collaborative agreements with leading companies.
|
Talent |
This program is a unique initiative to allow students to showcase their talent in the business world. An opportunity that will allow them to voice their concerns and share their business vision.
After completing this program, TECH helps students show the world their talent.
Show the world your talent after completing this program.
|
Multicultural Context |
While studying at TECH, students will enjoy a unique experience. Study in a multicultural context. In a program with a global vision, through which students can learn about the operating methods in different parts of the world, and gather the latest information that best adapts to their business idea.
TECH students represent more than 200 different nationalities.
|
Learn with the best |
In the classroom, TECH’s teaching staff discuss how they have achieved success in their companies, working in a real, lively, and dynamic context. Teachers who are fully committed to offering a quality specialization that will allow students to advance in their career and stand out in the business world.
Teachers representing 20 different nationalities.
TECH strives for excellence and, to this end, boasts a series of characteristics that make this university unique:
|
Analysis |
TECH explores the student’s critical side, their ability to question things, their problem-solving skills, as well as their interpersonal skills.
|
Academic Excellence |
TECH offers students the best online learning methodology. The university combines the Relearning method (a postgraduate learning methodology with the highest international rating) with the Case Study. A complex balance between tradition and state-of-the-art, within the context of the most demanding academic itinerary.
|
Economy of Scale |
TECH is the world’s largest online university. It currently boasts a portfolio of more than 10,000 university postgraduate programs. And in today's new economy, volume + technology = a ground-breaking price. This way, TECH ensures that studying is not as expensive for students as it would be at another university.
At TECH, you will have access to the most rigorous and up-to-date case studies in the academic community”
Syllabus
Through this university specialization, students will have a solid understanding of the principles of Artificial Intelligence and will be able to effectively integrate its tools into their Clinical Research projects. To this end, the syllabus will include topics such as Intelligent Systems, Algorithmics and Machine Learning. This will enable graduates to analyze large amounts of medical data, which will be used to make highly informed decisions. In addition, the program will include disruptive modules that will delve into Neural Networks, Model Personalization or Natural Language Processing.
This university program will allow you to exercise in simulated environments, which provide immersive learning programmed to specialize in front of real situations”
Syllabus
The Advanced master’s degree in MBA in Artificial Intelligence in Clinical Research at TECH Global University is an intensive program that prepares students to face business challenges and decisions internationally. Its content is designed to promote the development of managerial skills that enable more rigorous decision-making in uncertain environments.
Throughout 3,600 hours of study, students will analyze a multitude of practical cases through individual work, achieving high quality learning that can be applied to their daily practice. It is, therefore, an authentic immersion in real business situations.
This program deals in depth with the main areas of Artificial Intelligence and is designed for managers to understand its application in Clinical Research from a strategic, international and innovative perspective.
A plan designed for students, focused on their professional improvement and that prepares them to achieve excellence in the field of Artificial Intelligence in Clinical Research. A program that understands your needs and those of your company through innovative content based on the latest trends, and supported by the best educational methodology and an exceptional faculty, which will provide you with the competencies to solve critical situations in a creative and efficient way.
This program is developed over 2 years and is divided into 30 modules:
Module 1. Leadership, Ethics and Social Responsibility in Companies
Module 2. Strategic Managementand Executive Management
Module 3. People and Talent Management
Module 4. Economic and Financial Management
Module 5. Operations and Logistics Management
Module 6. Information Systems Management
Module 7. Commercial Management, Strategic Marketing and Corporate Communications
Module 8. Market Research, Advertising and Commercial Management
Module 9. Innovation and Project Management
Module 10. Executive Management
Module 11. Fundamentals of Artificial Intelligence
Module 12. Data Types and Life Cycle
Module 13. Data in Artificial Intelligence
Module 14. Data Mining: Selection, Pre-Processing and Transformation
Module 15. Algorithm and Complexity in Artificial Intelligence
Module 16. Intelligent Systems
Module 17. Machine Learning and Data Mining
Module 18. Neural networks, the basis of Deep Learning
Module 19. Deep Neural Networks Training
Module 20. Model Customization and Training with TensorFlow
Module 21. Deep Computer Vision with Convolutional Neural Networks
Module 22. Natural Language Processing (NLP) with Recurrent Neural Networks (RNN) and Attention
Module 23. Autoencoders, GANs, and Diffusion Models
Module 24. Bio-Inspired Computing
Module 25. Artificial Intelligence: Strategies and Applications
Module 26. Artificial Intelligence Methods and Tools for Clinical Research
Module 27. Biomedical Research with AI
Module 28. Practical Application of Artificial Intelligence in Clinical Research
Module 29. Big Data Analytics and Machine Learning in Clinical Research
Module 30. Ethical, Legal and Future Aspects of Artificial Intelligence in Clinical Research
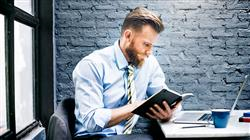
Where, When and How is it Taught?
TECH offers the possibility of developing this MBA in Artificial Intelligence in Clinical Research completely online. During the 2 years of the program, students will be able to access all the contents in this program at any time, which will allow them to manage their own study time
Module 1. Leadership, Ethics and Social Responsibility in Companies
1.1. Globalization and Governance
1.1.1. Governance and Corporate Governance
1.1.2. The Fundamentals of Corporate Governance in Companies
1.1.3. The Role of the Board of Directors in the Corporate Governance Framework
1.2. Leadership
1.2.1. Leadership A Conceptual Approach
1.2.2. Leadership in Companies
1.2.3. The Importance of Leaders in Business Management
1.3. Cross Cultural Management
1.3.1. Cross Cultural Management Concept
1.3.2. Contributions to Knowledge of National Cultures
1.3.3. Diversity Management
1.4. Management and Leadership Development
1.4.1. Concept of Management Development
1.4.2. Concept of Leadership
1.4.3. Leadership Theories
1.4.4. Leadership Styles
1.4.5. Intelligence in Leadership
1.4.6. The Challenges of Today's Leader
1.5. Business Ethics
1.5.1. Ethics and Morality
1.5.2. Business Ethics
1.5.3. Leadership and Ethics in Companies
1.6. Sustainability
1.6.1. Sustainability and Sustainable Development
1.6.2. The 2030 Agenda
1.6.3. Sustainable Companies
1.7. Corporate Social Responsibility
1.7.1. International Dimensions of Corporate Social Responsibility
1.7.2. Implementing Corporate Social Responsibility
1.7.3. The Impact and Measurement of Corporate Social Responsibility
1.8. Responsible Management Systems and Tools
1.8.1. CSR: Corporate Social Responsibility
1.8.2. Essential Aspects for Implementing a Responsible Management Strategy
1.8.3. Steps for the Implementation of a Corporate Social Responsibility Management System
1.8.4. CSR Tools and Standards
1.9. Multinationals and Human Rights
1.9.1. Globalization, Multinational Companies and Human Rights
1.9.2. Multinational Companies vs. International Law
1.9.3. Legal Instruments for Multinationals in the Area of Human Rights
1.10. Legal Environment and Corporate Governance
1.10.1. International Rules on Importation and Exportation
1.10.2. Intellectual and Industrial Property
1.10.3. International Labor Law
Module 2. Strategic Management and Executive Management
2.1. Organizational Analysis and Design
2.1.1. Conceptual Framework
2.1.2. Key Elements in Organizational Design
2.1.3. Basic Organizational Models
2.1.4. Organizational Design: Typologies
2.2. Corporate Strategy
2.2.1. Competitive Corporate Strategy
2.2.2. Types of Growth Strategies
2.2.3. Conceptual Framework
2.3. Strategic Planning and Strategy Formulation
2.3.1. Conceptual Framework
2.3.2. Elements of Strategic Planning
2.3.3. Strategy Formulation: Strategic Planning Process
2.4. Strategic Thinking
2.4.1. The Company as a System
2.4.2. Organization Concept
2.5. Financial Diagnosis
2.5.1. Concept of Financial Diagnosis
2.5.2. Stages of Financial Diagnosis
2.5.3. Assessment Methods for Financial Diagnosis
2.6. Planning and Strategy
2.6.1. The Plan from a Strategy
2.6.2. Strategic Positioning
2.6.3. Strategy in Companies
2.7. Strategy Models and Patterns
2.7.1. Conceptual Framework
2.7.2. Strategic Models
2.7.3. Strategic Patterns: The Five P’s of Strategy
2.8. Competitive Strategy
2.8.1. The Competitive Advantage
2.8.2. Choosing a Competitive Strategy
2.8.3. Strategies Based on the Strategic Clock Model
2.8.4. Types of Strategies According to the Industrial Sector Life Cycle
2.9. Strategic Management
2.9.1. The Concept of Strategy
2.9.2. The Process of Strategic Management
2.9.3. Approaches in Strategic Management
2.10. Strategy Implementation
2.10.1. Indicator Systems and Process Approach
2.10.2. Strategic Map
2.10.3. Strategic Alignment
2.11. Executive Management
2.11.1. Conceptual Framework of Executive Management
2.11.2. Executive Management The Role of the Board of Directors and Corporate Management Tools
2.12. Strategic Communication
2.12.1. Interpersonal Communication
2.12.2. Communication Skills and Influence
2.12.3. Internal Communication
2.12.4. Barriers to Business Communication
Module 3. People and Talent Management
3.1. Organizational Behavior
3.1.1. Organizational Behavior Conceptual Framework
3.1.2. Main Factors of Organizational Behavior
3.2. People in Organizations
3.2.1. Quality of Work Life and Psychological Well-Being
3.2.2. Work Teams and Meeting Management
3.2.3. Coaching and Team Management
3.2.4. Managing Equality and Diversity
3.3. Strategic People Management
3.3.1. Strategic Human Resources Management
3.3.2. Strategic People Management
3.4. Evolution of Resources An Integrated Vision
3.4.1. The Importance of HR
3.4.2. A New Environment for People Management and Leadership
3.4.3. Strategic HR Management
3.5. Selection, Group Dynamics and HR Recruitment
3.5.1. Approach to Recruitment and Selection
3.5.2. Recruitment.
3.5.3. The Selection Process
3.6. Human Resources Management by Competencies
3.6.1. Analysis of the Potential
3.6.2. Remuneration Policy
3.6.3. Career/Succession Planning
3.7. Performance Evaluation and Compliance Management
3.7.1. Performance Management
3.7.2. Performance Management: Objectives and Process
3.8. Training Management
3.8.1. Learning Theories
3.8.2. Talent Detection and Retention
3.8.3. Gamification and Talent Management
3.8.4. Training and Professional Obsolescence
3.9. Talent Management
3.9.1. Keys for Positive Management
3.9.2. Conceptual Origin of Talent and its Implication in the Company
3.9.3. Map of Talent in the Organization
3.9.4. Cost and Added Value
3.10. Innovation in Talent and People Management
3.10.1. Strategic Talent Management Models
3.10.2. Identification, Training and Development of Talent
3.10.3. Loyalty and Retention
3.10.4. Proactivity and Innovation
3.11. Motivation
3.11.1. The Nature of Motivation
3.11.2. Expectations Theory
3.11.3. Needs Theory
3.11.4. Motivation and Financial Compensation
3.12. Employer Branding
3.12.1. Employer Branding in HR
3.12.2. Personal Branding for HR Professionals
3.13. Developing High Performance Teams
3.13.1. High-Performance Teams: Self-Managed Teams
3.13.2. Methodologies for the Management of High Performance Self-Managed Teams
3.14. Management Skills Development
3.14.1. What are Manager Competencies?
3.14.2. Elements of Competencies
3.14.3. Knowledge
3.14.4. Management Skills
3.14.5. Attitudes and Values in Managers
3.14.6. Managerial Skills
3.15. Time Management
3.15.1. Benefits
3.15.2. What Can be the Causes of Poor Time Management?
3.15.3. Time
3.15.4. Time Illusions
3.15.5. Attention and Memory
3.15.6. State of Mind
3.15.7. Time Management
3.15.8. Being Proactive
3.15.9. Be Clear About the Objective
3.15.10. Order
3.15.11. Planning
3.16. Change Management
3.16.1. Change Management
3.16.2. Type of Change Management Processes
3.16.3. Stages or Phases in the Change Management Process
3.17. Negotiation and Conflict Management
3.17.1 Negotiation
3.17.2 Conflicts Management
3.17.3 Crisis Management
3.18. Executive Communication
3.18.1. Internal and External Communication in the Corporate Environment
3.18.2. Communication Departments
3.18.3. The Person in Charge of Communication of the Company. The Profile of the Dircom
3.19. Human Resources Management and PRL Teams
3.19.1. Management of Human Resources and Teams
3.19.2. Prevention of Occupational Hazards
3.20. Productivity, Attraction, Retention and Activation of Talent
3.20.1. Productivity
3.20.2. Talent Attraction and Retention Levers
3.21. Monetary Compensation Vs. Non-Cash
3.21.1. Monetary Compensation Vs. Non-Cash
3.21.2. Wage Band Models
3.21.3. Non-cash Compensation Models
3.21.4. Working Model
3.21.5. Corporate Community
3.21.6. Company Image
3.21.7. Emotional Salary
3.22. Innovation in Talent and People Management II
3.22.1. Innovation in Organizations
3.22.2. New Challenges in the Human Resources Department
3.22.3. Innovation Management
3.22.4. Tools for Innovation
3.23. Knowledge and Talent Management
3.23.1. Knowledge and Talent Management
3.23.2. Knowledge Management Implementation
3.24. Transforming Human Resources in the Digital Era
3.24.1. The Socioeconomic Context
3.24.2. New Forms of Corporate Organization
3.24.3. New Methodologies
Module 4. Economic and Financial Management
4.1. Economic Environment
4.1.1. Macroeconomic Environment and the National Financial System
4.1.2. Financial Institutions
4.1.3. Financial Markets
4.1.4. Financial Assets
4.1.5. Other Financial Sector Entities
4.2. Company Financing
4.2.1. Sources of Financing
4.2.2. Types of Financing Costs
4.3. Executive Accounting
4.3.1. Basic Concepts
4.3.2. The Company's Assets
4.3.3. The Company's Liabilities
4.3.4. The Company's Net Worth
4.3.5. The Income Statement
4.4. From General Accounting to Cost Accounting
4.4.1. Elements of Cost Calculation
4.4.2. Expenses in General Accounting and Cost Accounting
4.4.3. Costs Classification
4.5. Information Systems and Business Intelligence
4.5.1. Fundamentals and Classification
4.5.2. Cost Allocation Phases and Methods
4.5.3. Choice of Cost Center and Impact
4.6. Budget and Management Control
4.6.1. The Budget Model
4.6.2. The Capital Budget
4.6.3. The Operating Budget
4.6.4. Treasury Budget
4.6.5. Budget Monitoring
4.7. Treasury Management
4.7.1. Accounting Working Capital and Necessary Working Capital
4.7.2. Calculation of Operating Requirementsof Funds
4.7.3. Credit Management
4.8. Corporate Tax Responsibility
4.8.1. Basic Tax Concepts
4.8.2. Corporate Income Tax
4.8.3. Value Added Tax
4.8.4. Other Taxes Related to Commercial with the Mercantile Activity
4.8.5. The Company as a Facilitator of the Work of the of the State
4.9. Systems of Controlof Enterprises
4.9.1. Analysis of Financial Statements
4.9.2. The Company's Balance Sheet
4.9.3. The Profit and Loss Statement
4.9.4. The Statement of Cash Flows
4.9.5. Ratio Analysis
4.10. Financial Management
4.10.1. The Company's Financial Decisions
4.10.2. Financial Department
4.10.3. Cash Surpluses
4.10.4. Risks Associated with Financial Management
4.10.5. Financial Administration Risk Management
4.11. Financial Planning
4.11.1. Definition of Financial Planning
4.11.2. Actions to be Taken in Financial Planning
4.11.3. Creation and Establishment of the Business Strategy
4.11.4. The Cash Flow Table
4.11.5. The Working Capital Table
4.12. Corporate Financial Strategy
4.12.1. Corporate Strategy and Sources of Financing
4.12.2. Financial Products for Corporate Financing
4.13. Macroeconomic Context
4.13.1. Macroeconomic Context
4.13.2. Relevant Economic Indicators
4.13.3. Mechanisms for Monitoring of Macroeconomic Magnitudes
4.13.4. Economic Cycles
4.14. Strategic Financing
4.14.1. Self-Financing
4.14.2. Increase in Equity
4.14.3. Hybrid Resources
4.14.4. Financing Through Intermediaries
4.15. Money and Capital Markets
4.15.1. The Money Market
4.15.2. The Fixed Income Market
4.15.3. The Equity Market
4.15.4. The Foreign Exchange Market
4.15.5. The Derivatives Market
4.16. Financial Analysis and Planning
4.16.1. Analysis of the Balance Sheet
4.16.2. Analysis of the Income Statement
4.16.3. Profitability Analysis
4.17. Analysis and Resolution of Cases/Problems
4.17.1. Financial Information on Industria de Diseño y Textil, S.A. (INDITEX)
Module 5. Operations and Logistics Management
5.1. Operations Direction and Management
5.1.1. The Role of Operations
5.1.2. The Impact of Operations on the Management of Companies.
5.1.3. Introduction to Operations Strategy
5.1.4. Operations Management
5.2. Industrial Organization and Logistics
5.2.1. Industrial Organization Department
5.2.2. Logistics Department
5.3. Structure and Types of Production (MTS, MTO, ATO, ETO, etc)
5.3.1. Production System
5.3.2. Production Strategy
5.3.3. Inventory Management System
5.3.4. Production Indicators
5.4. Structure and Types of Procurement
5.4.1. Function of Procurement
5.4.2. Procurement Management
5.4.3. Types of Purchases
5.4.4. Efficient Purchasing Management of a Company
5.4.5. Stages of the Purchase Decision Process
5.5. Economic Control of Purchasing
5.5.1. Economic Influence of Purchases
5.5.2. Cost Centers
5.5.3. Budget
5.5.4. Budgeting vs. Actual Expenditure
5.5.5. Budgetary Control Tools
5.6. Warehouse Operations Control
5.6.1. Inventory Control
5.6.2. Location Systems
5.6.3. Stock Management Techniques
5.6.4. Storage Systems
5.7. Strategic Purchasing Management
5.7.1. Business Strategy
5.7.2. Strategic Planning
5.7.3. Purchasing Strategies
5.8. Typologies of the Supply Chain (SCM)
5.8.1. Supply Chain
5.8.2. Benefits of Supply Chain Management
5.8.3. Logistical Management in the Supply Chain
5.9. Supply Chain Management
5.9.1. The Concept of Management of the Supply Chain (SCM)
5.9.2. Supply Chain Costs and Efficiency
5.9.3. Demand Patterns
5.9.4. Operations Strategy and Change
5.10. Interactions Between the SCM and All Other Departments
5.10.1. Interaction of the Supply Chain
5.10.2. Interaction of the Supply Chain. Integration by Parts
5.10.3. Supply Chain Integration Problems
5.10.4. Supply Chain
5.11. Logistics Costs
5.11.1. Logistics Costs
5.11.2. Problems with Logistics Costs
5.11.3. Optimizing Logistic Costs
5.12. Profitability and Efficiency of Logistics Chains: KPIS
5.12.1. Logistics Chain
5.12.2. Profitability and Efficiency of the Logistics Chain
5.12.3. Indicators of Profitability and Efficiency of the Supply Chain
5.13. Process Management
5.13.1. Process Management
5.13.2. Process-Based Approach: Process Mapping
5.13.3. Improvements in Process Management
5.14. Distribution and Transportation and Logistics
5.14.1. Distribution in the Supply Chain
5.14.2. Transportation Logistics
5.14.3. Geographic Information Systems as a Support to Logistics
5.15. Logistics and Customers
5.15.1. Demand Analysis
5.15.2. Demand and Sales Forecast
5.15.3. Sales and Operations Planning
5.15.4. Participatory Planning, Forecasting and and Replenishment Planning (CPFR)
5.16. International Logistics
5.16.1. Export and Import Processes
5.16.2. Customs
5.16.3. Methods and Means of International Payment
5.16.4. International Logistics Platforms
5.17. Outsourcing of Operations
5.17.1. Operations Management and Outsourcing
5.17.2. Outsourcing Implementation in Logistics Environments
5.18. Competitiveness in Operations
5.18.1. Operations Management
5.18.2. Operational Competitiveness
5.18.3. Operations Strategy and Competitive Advantages
5.19. Quality Management
5.19.1. Internal and External Customers
5.19.2. Quality Costs
5.19.3. Ongoing Improvement and the Deming Philosophy
Module 6. Information Systems Management
6.1. Technological Environment
6.1.1. Technology and Globalization
6.1.2. Economic Environment and Technology
6.1.3. Technological Environment and its Impact on Companies
6.2. Information Systems and Technologies in the Enterprise
6.2.1. The Evolution of the IT Model
6.2.2. Organization and IT Departments
6.2.3. Information Technology and Economic Environment
6.3. Corporate Strategy and Technology Strategy
6.3.1. Creating Value for Customers and Shareholders
6.3.2. Strategic IS/IT Decisions
6.3.3. Corporate Strategy Vs. Technology and Digital Strategy
6.4. Information Systems Management
6.4.1. Corporate Governance of Technology and Information Systems
6.4.2. Management of Information Systems in Companies
6.4.3. Expert Managers in Information Systems: Roles and Functions
6.5. Information Technology Strategic Planning
6.5.1. Information Systems and Corporate Strategy
6.5.2. Strategic Planning of Information Systems
6.5.3. Phases of Information Systems Strategic Planning
6.6. Information Systems for Decision-Making
6.6.1. Business Intelligence
6.6.2. Data Warehouse
6.6.3. BSC or Balanced Scorecard
6.7. Exploring the Information
6.7.1. SQL: Relational Databases Basic Concepts
6.7.2. Networks and Communications
6.7.3. Operational System: Standardized Data Models
6.7.4. Strategic System: OLAP, Multidimensional Model and Graphical Dashboards
6. 7.5. Strategic DB Analysis and Report Composition
6.8. Enterprise Business Intelligence
6.8.1. The World of Data
6.8.2. Relevant Concepts
6.8.3. Main Characteristics
6.8.4. Solutions in Today's Market
6.8.5. Overall Architecture of a BI Solution
6.8.6. Cybersecurity in BI and Data Science
6.9. New Business Concept
6.9.1. Why BI
6.9.2. Obtaining Information
6.9.3. BI in the Different Departments of the Company
6.9.4. Reasons to Invest in BI
6.10. BI Tools and Solutions
6.10.1. How to Choose the Best Tool?
6.10.2. Microsoft Power BI, MicroStrategy y Tableau
6.10.3. SAP BI, SAS BI and Qlikview
6.10.4. Prometheus
6.11. BI Project Planning and Management
6.11.1. First Steps to Define a BI Project
6.11.2. BI Solution for the Company
6.11.3. Requirements and Objectives
6.12. Corporate Management Applications
6.12.1. Information Systems and Corporate Management
6.12.2. Applications for Corporate Management
6.12.3. Enterprise Resource Planning or ERP Systems
6.13. Digital Transformation
6.13.1. Conceptual Framework of Digital Transformation
6.13.2. Digital Transformation; Key Elements, Benefits and Drawbacks
6.13.3. Digital Transformation in Companies
6.14. Technology and Trends
6.14.1. Main Trends in the Field of Technology that are Changing Business Models
6.14.2. Analysis of the Main Emerging Technologies
6.15. IT Outsourcing
6.15.1. Conceptual Framework of Outsourcing
6.15.2. IT Outsourcing and its Impact on the Business
6.15.3. Keys to Implement Corporate IT Outsourcing Projects
Module 7. Commercial Management, Strategic Marketing and Corporate Communication
7.1. Commercial Management
7.1.1. Conceptual Framework of Commercial Management
7.1.2. Business Strategy and Planning
7.1.3. The Role of Sales Managers
7.2. Marketing
7.2.1. The Concept of Marketing
7.2.2. Basic Elements of Marketing
7.2.3. Marketing Activities of the Company
7.3. Strategic Marketing Management
7.3.1. The Concept of Strategic Marketing
7.3.2. Concept of Strategic Marketing Planning
7.3.3. Stages in the Process of Strategic Marketing Planning
7.4. Digital Marketing and E-Commerce
7.4.1. Digital Marketing and E-Commerce Objectives
7.4.2. Digital Marketing and Media Used
7.4.3. E-Commerce General Context
7.4.4. Categories of E-Commerce
7.4.5. Advantages and Disadvantages of E-Commerce Versus Traditional Commerce.
7.5. Managing Digital Business
7.5.1. Competitive Strategy in the Face of the Growing Digitalization of the Media
7.5.2. Design and Creation of a Digital Marketing Plan
7.5.3. ROI Analysis in a Digital Marketing Plan
7.6. Digital Marketing to Reinforce the Brand
7.6.1. Online Strategies to Improve Your Brand's Reputation
7.6.2. Branded Content and Storytelling
7.7. Digital Marketing Strategy
7.7.1. Defining the Digital Marketing Strategy
7.7.2. Digital Marketing Strategy Tools
7.8. Digital Marketing to Attract and Retain Customers
7.8.1. Loyalty and Engagement Strategies Through the Internet
7.8.2. Visitor Relationship Management
7.8.3. Hypersegmentation
7.9. Managing Digital Campaigns
7.9.1. What is a Digital Advertising Campaign?
7.9.2. Steps to Launch an Online Marketing Campaign
7.9.3. Mistakes in Digital Advertising Campaigns
7.10. Online Marketing Plan
7.10.1. What is an Online Marketing Plan?
7.10.2. Steps to Create an Online Marketing Plan
7.10.3. Advantages of Having an Online Marketing Plan
7.11. Blended Marketing
7.11.1. What is Blended Marketing?
7.11.2. Differences Between Online and Offline Marketing
7.11.3. Aspects to be Taken into Account in the Blended Marketing Strategy
7.11.4. Characteristics of a Blended Marketing Strategy
7.11.5. Recommendations in Blended Marketing
7.11.6. Benefits of Blended Marketing
7.12. Sales Strategy
7.12.1. Sales Strategy
7.12.2. Sales Methods
7.13. Corporate Communication
7.13.1. Concept
7.13.2. The Importance of Communication in the Organization
7.13.3. Type of Communication in the Organization
7.13.4. Functions of Communication in the Organization
7.13.5. Elements of Communication
7.13.6. Communication Problems
7.13.7. Communication Scenarios
7.14. Corporate Communication Strategy
7.14.1. Motivational Programs, Social Action, Participation and Training with HR
7.14.2. Internal Communication Tools and Supports
7.14.3. Internal Communication Plan
7.15. Digital Communication and Reputation
7.15.1. Online Reputation
7.15.2. How to Measure Digital Reputation?
7.15.3. Online Reputation Tools
7.15.4. Online Reputation Report
7.15.5. Online Branding
Module 8. Market Research, Advertising and Commercial Management
8.1. Market Research
8.1.1. Marketing Research: Historical Origin
8.1.2. Analysis and Evolution of the Conceptual Framework of Marketing Research
8.1.3. Key Elements and Value Contribution of Market Research
8.2. Quantitative Research Methods and Techniques
8.2.1. Sample Size
8.2.2. Sampling
8.2.3. Types of Quantitative Techniques
8.3. Qualitative Research Methods and Techniques
8.3.1. Types of Qualitative Research
8.3.2. Qualitative Research Techniques
8.4. Market Segmentation
8.4.1. Market Segmentation Concept
8.4.2. Utility and Segmentation Requirements
8.4.3. Consumer Market Segmentation
8.4.4. Industrial Market Segmentation
8.4.5. Segmentation Strategies
8.4.6. Segmentation Based on Marketing - Mix Criteria
8.4.7. Market Segmentation Methodology
8.5. Research Project Management
8.5.1. Market Research as a Process
8.5.2. Planning Stages in Market Research
8.5.3. Stages of Market Research Implementation
8.5.4. Managing a Research Project
8.6. International Market Research
8.6.1. International Market Research
8.6.2. International Market Research Process
8.6.3. The Importance of Secondary Sources in International Market Research
8.7. Feasibility Studies
8.7.1. Concept and Usefulness
8.7.2. Outline of a Feasibility Study
8.7.3. Development of a Feasibility Study
8.8. Publicity
8.8.1. Historical Background of Advertising
8.8.2. Conceptual Framework of Advertising; Principles, Concept of Briefing and Positioning
8.8.3. Advertising Agencies, Media Agencies and Advertising Professionals
8.8.4. Importance of Advertising in Business
8.8.5. Advertising Trends and Challenges
8.9. Developing the Marketing Plan
8.9.1. Marketing Plan Concept
8.9.2. Situation Analysis and Diagnosis
8.9.3. Strategic Marketing Decisions
8.9.4. Operational Marketing Decisions
8.10. Promotion and Merchandising Strategies
8.10.1. Integrated Marketing Communication
8.10.2. Advertising Communication Plan
8.10.3. Merchandising as a Communication Technique
8.11. Media Planning
8.11.1. Origin and Evolution of Media Planning
8.11.2. Media
8.11.3. Media Plan
8.12. Fundamentals of Commercial Management
8.12.1. The Role of Commercial Management
8.12.2. Systems of Analysis of the Company/Market Commercial Competitive Situation
8.12.3. Commercial Planning Systems of the Company
8.12.4. Main Competitive Strategies
8.13. Commercial Negotiation
8.13.1. Commercial Negotiation
8.13.2. Psychological Issues in Negotiation
8.13.3. Main Negotiation Methods
8.13.4. The Negotiation Process
8.14. Decision-Making in Commercial Management
8.14.1. Commercial Strategy and Competitive Strategy
8.14.2. Decision Making Models
8.14.3. Decision-Making Analytics and Tools
8.14.4. Human Behavior in Decision Making
8.15. Leadership and Management of the Sales Network
8.15.1. Sales Management Sales Management
8.15.2. Networks Serving Commercial Activity
8.15.3. Salesperson Recruitment and Training Policies
8.15.4. Remuneration Systems for Own and External Commercial Networks
8.15.5. Management of the Commercial Process Control and Assistance to the Work of the Sales Representatives Based on the Information
8.16. Implementing the Commercial Function
8.16.1. Recruitment of Own Sales Representatives and Sales Agents
8.16.2. Controlling Commercial Activity
8.16.3. The Code of Ethics of Sales Personnel
8.16.4. Compliance with Legislation
8.16.5. Generally Accepted Standards of Business Conduct
8.17. Key Account Management
8.17.1. Concept of Key Account Management
8.17.2. The Key Account Manager
8.17.3. Key Account Management Strategy
8.18. Financial and Budgetary Management
8.18.1. The Break-Even Point
8.18.2. The Sales Budget Control of Management and of the Annual Sales Plan
8.18.3. Financial Impact of Strategic Sales Decisions
8.18.4. Cycle Management, Turnover, Profitability and Liquidity
8.18.5. Income Statement
Module 9. Innovation and Project Management
9.1. Innovation
9.1.1. Introduction to Innovation
9.1.2. Innovation in the Entrepreneurial Ecosystem
9.1.3. Instruments and Tools for the Business Innovation Process
9.2. Innovation Strategy
9.2.1. Strategic Intelligence and Innovation
9.2.2. Innovation from Strategy
9.3. Project Management for Startups
9.3.1. Startup Concept
9.3.2. Lean Startup Philosophy
9.3.3. Stages of Startup Development
9.3.4. The Role of a Project Manager in a Startup
9.4. Business Model Design and Validation
9.4.1. Conceptual Framework of a Business Model
9.4.2. Business Model Design and Validation
9.5. Project Management
9.5.1. Project Management: Identification of Opportunities to Develop Corporate Innovation Projects
9.5.2. Main stages or Phases in the Direction and Management of Innovation Projects
9.6. Project Change Management: Training Management
9.6.1. Concept of Change Management
9.6.2. The Change Management Process
9.6.3. Change Implementation
9.7. Project Communication Management
9.7.1. Project Communications Management
9.7.2. Key Concepts for Project Communications Management
9.7.3. Emerging Trends
9.7.4. Adaptations to Equipment
9.7.5. Planning Communications Management
9.7.6. Manage Communications
9.7.7. Monitoring Communications
9.8. Traditional and Innovative Methodologies
9.8.1. Innovative Methodologies
9.8.2. Basic Principles of Scrum
9.8.3. Differences between the Main Aspects of Scrum and Traditional Methodologies
9.9. Creation of a Startup
9.9.1. Creation of a Startup
9.9.2. Organization and Culture
9.9.3. Top Ten Reasons Why Startups Fail
9.9.4. Legal Aspects
9.10. Project Risk Management Planning
9.10.1. Risk Planning
9.10.2. Elements for Creating a Risk Management Plan
9.10.3. Tools for Creating a Risk Management Plan
9.10.4. Content of the Risk Management Plan
Module 10. Executive Management
10.1. General Management
10.1.1. The Concept of General Management
10.1.2. The General Manager's Action
10.1.3. The CEO and their Responsibilities
10.1.4. Transforming the Work of Management
10.2. Manager Functions: Organizational Culture and Approaches
10.2.1. Manager Functions: Organizational Culture and Approaches
10.3. Operations Management
10.3.1. The Importance of Management
10.3.2. Value Chain
10.3.3. Quality Management
10.4. Public Speaking and Spokesperson Education
10.4.1. Interpersonal Communication
10.4.2. Communication Skills and Influence
10.4.3. Communication Barriers
10.5. Personal and Organizational Communications Tools
10.5.1. Interpersonal Communication
10.5.2. Interpersonal Communication Tools
10.5.3. Communication in the Organization
10.5.4. Tools in the Organization
10.6. Communication in Crisis Situations
10.6.1. Crisis
10.6.2. Phases of the Crisis
10.6.3. Messages: Contents and Moments
10.7. Preparation of a Crisis Plan
10.7.1. Analysis of Possible Problems
10.7.2. Planning
10.7.3. Adequacy of Personnel
10.8. Emotional Intelligence
10.8.1. Emotional Intelligence and Communication
10.8.2. Assertiveness, Empathy, and Active Listening
10.8.3. Self-Esteem and Emotional Communication
10.9. Personal Branding
10.9.1. Strategies to Develop Personal Branding
10.9.2. Personal Branding Laws
10.9.3. Tools for Creating Personal Brands
10.10. Leadership and Team Management
10.10.1. Leadership and Leadership Styles
10.10.2. Leader Capabilities and Challenges
10.10.3. Managing Change Processes
10.10.4. Managing Multicultural Teams
Module 11. Fundamentals of Artificial Intelligence
11.1. History of Artificial Intelligence
11.1.1. When Do We Start Talking About Artificial Intelligence?
11.1.2. References in Film
11.1.3. Importance of Artificial Intelligence
11.1.4. Technologies that Enable and Support Artificial Intelligence
11.2. Artificial Intelligence in Games
11.2.1. Game Theory
11.2.2. Minimax and Alpha-Beta Pruning
11.2.3. Simulation: Monte Carlo
11.3. Neural Networks
11.3.1. Biological Fundamentals
11.3.2. Computational Model
11.3.3. Supervised and Unsupervised Neural Networks
11.3.4. Simple Perceptron
11.3.5. Multilayer Perceptron
11.4. Genetic Algorithms
11.4.1. History
11.4.2. Biological Basis
11.4.3. Problem Coding
11.4.4. Generation of the Initial Population
11.4.5. Main Algorithm and Genetic Operators
11.4.6. Evaluation of Individuals: Fitness
11.5. Thesauri, Vocabularies, Taxonomies
11.5.1. Vocabulary
11.5.2. Taxonomy
11.5.3. Thesauri
11.5.4. Ontologies
11.5.5. Knowledge Representation Semantic Web
11.6. Semantic Web
11.6.1. Specifications RDF, RDFS and OWL
11.6.2. Inference/ Reasoning
11.6.3. Linked Data
11.7. Expert Systems and DSS
11.7.1. Expert Systems
11.7.2. Decision Support Systems
11.8. Chatbots and Virtual Assistants
11.8.1. Types of Assistants: Voice and Text Assistants
11.8.2. Fundamental Parts for the Development of an Assistant: Intents, Entities and Dialogue Flow
11.8.3. Integrations: Web, Slack, WhatsApp, Facebook
11.8.4. Assistant Development Tools: Dialog Flow, Watson Assistant
11.9. AI Implementation Strategy
11.10. Future of Artificial Intelligence
11.10.1. Understand How to Detect Emotions Using Algorithms
11.10.2. Creating a Personality: Language, Expressions and Content
11.10.3. Trends of Artificial Intelligence
11.10.4. Reflections
Module 12. Data Types and Life Cycle
12.1. Statistics
12.1.1. Statistics: Descriptive Statistics, Statistical Inferences
12.1.2. Population, Sample, Individual
12.1.3. Variables: Definition, Measurement Scales
12.2. Types of Data Statistics
12.2.1. According to Type
12.2.1.1. Quantitative: Continuous Data and Discrete Data
12.2.1.2. Qualitative. Binomial Data, Nominal Data and Ordinal Data
12.2.2. According to their Shape
12.2.2.1. Numeric
12.2.2.2. Text:
12.2.2.3. Logical
12.2.3. According to its Source
12.2.3.1. Primary
12.2.3.2. Secondary
12.3. Life Cycle of Data
12.3.1. Stages of the Cycle
12.3.2. Milestones of the Cycle
12.3.3. FAIR Principles
12.4. Initial Stages of the Cycle
12.4.1. Definition of Goals
12.4.2. Determination of Resource Requirements
12.4.3. Gantt Chart
12.4.4. Data Structure
12.5. Data Collection
12.5.1. Methodology of Data Collection
12.5.2. Data Collection Tools
12.5.3. Data Collection Channels
12.6. Data Cleaning
12.6.1. Phases of Data Cleansing
12.6.2. Data Quality
12.6.3. Data Manipulation (with R)
12.7. Data Analysis, Interpretation and Result Evaluation
12.7.1. Statistical Measures
12.7.2. Relationship Indexes
12.7.3. Data Mining
12.8. Datawarehouse
12.8.1. Elements that Comprise it
12.8.2. Design
12.8.3. Aspects to Consider
12.9. Data Availability
12.9.1. Access
12.9.2. Uses
12.9.3. Security
12.10. Regulatory Framework
12.10.1. Data Protection Law
12.10.2. Good Practices
12.10.3. Other Regulatory Aspects
Module 13. Data in Artificial Intelligence
13.1. Data Science
13.1.1. Data Science
13.1.2. Advanced Tools for the Data Scientist
13.2. Data, Information and Knowledge
13.2.1. Data, Information and Knowledge
13.2.2. Types of Data
13.2.3. Data Sources
13.3. From Data to Information
13.3.1. Data Analysis
13.3.2. Types of Analysis
13.3.3. Extraction of Information from a Dataset
13.4. Extraction of Information Through Visualization
13.4.1. Visualization as an Analysis Tool
13.4.2. Visualization Methods
13.4.3. Visualization of a Data Set
13.5. Data Quality
13.5.1. Quality Data
13.5.2. Data Cleaning
13.5.3. Basic Data Pre-Processing
13.6. Dataset
13.6.1. Dataset Enrichment
13.6.2. The Curse of Dimensionality
13.6.3. Modification of Our Data Set
13.7. Unbalance
13.7.1. Classes of Unbalance
13.7.2. Unbalance Mitigation Techniques
13.7.3. Balancing a Dataset
13.8. Unsupervised Models
13.8.1. Unsupervised Model
13.8.2. Methods
13.8.3. Classification with Unsupervised Models
13.9. Supervised Models
13.9.1. Supervised Model
13.9.2. Methods
13.9.3. Classification with Supervised Models
13.10. Tools and Good Practices
13.10.1. Good Practices for Data Scientists
13.10.2. The Best Model
13.10.3. Useful Tools
Module 14. Data Mining Selection, Pre-Processing and Transformation
14.1. Statistical Inference
14.1.1. Descriptive Statistics vs. Statistical Inference
14.1.2. Parametric Procedures
14.1.3. Non-Parametric Procedures
14.2. Exploratory Analysis
14.2.1. Descriptive Analysis
14.2.2. Visualization
14.2.3. Data Preparation
14.3. Data Preparation
14.3.1. Integration and Data Cleaning
14.3.2. Normalization of Data
14.3.3. Transforming Attributes
14.4. Missing Values
14.4.1. Treatment of Missing Values
14.4.2. Maximum Likelihood Imputation Methods
14.4.3. Missing Value Imputation Using Machine Learning
14.5. Noise in the Data
14.5.1. Noise Classes and Attributes
14.5.2. Noise Filtering
14.5.3. The Effect of Noise
14.6. The Curse of Dimensionality
14.6.1. Oversampling
14.6.2. Undersampling
14.6.3. Multidimensional Data Reduction
14.7. From Continuous to Discrete Attributes
14.7.1. Continuous Data Vs. Discreet Data
14.7.2. Discretization Process
14.8. The Data
14.8.1. Data Selection
14.8.2. Prospects and Selection Criteria
14.8.3. Selection Methods
14.9. Instance Selection
14.9.1. Methods for Instance Selection
14.9.2. Prototype Selection
14.9.3. Advanced Methods for Instance Selection
14.10. Data Pre-Processing in Big Data Environments
Module 15. Algorithm and Complexity in Artificial Intelligence
15.1. Introduction to Algorithm Design Strategies
15.1.1. Recursion
15.1.2. Divide and Conquer
15.1.3. Other Strategies
15.2. Efficiency and Analysis of Algorithms
15.2.1. Efficiency Measures
15.2.2. Measuring the Size of the Input
15.2.3. Measuring Execution Time
15.2.4. Worst, Best and Average Case
15.2.5. Asymptotic Notation
15.2.6. Criteria for Mathematical Analysis of Non-Recursive Algorithms
15.2.7. Mathematical Analysis of Recursive Algorithms
15.2.8. Empirical Analysis of Algorithms
15.3. Sorting Algorithms
15.3.1. Concept of Sorting
15.3.2. Bubble Sorting
15.3.3. Sorting by Selection
15.3.4. Sorting by Insertion
15.3.5. Merge Sort
15.3.6. Quick Sort
15.4. Algorithms with Trees
15.4.1. Tree Concept
15.4.2. Binary Trees
15.4.3. Tree Paths
15.4.4. Representing Expressions
15.4.5. Ordered Binary Trees
15.4.6. Balanced Binary Trees
15.5. Algorithms Using Heaps
15.5.1. Heaps
15.5.2. The Heapsort Algorithm
15.5.3. Priority Queues
15.6. Graph Algorithms
15.6.1. Representation
15.6.2. Traversal in Width
15.6.3. Depth Travel
15.6.4. Topological Sorting
15.7. Greedy Algorithms
15.7.1. Greedy Strategy
15.7.2. Elements of the Greedy Strategy
15.7.3. Currency Exchange
15.7.4. Traveler’s Problem
15.7.5. Backpack Problem
15.8. Minimal Path Finding
15.8.1. The Minimum Path Problem
15.8.2. Negative Arcs and Cycles
15.8.3. Dijkstra's Algorithm
15.9. Greedy Algorithms on Graphs
15.9.1. The Minimum Covering Tree
15.9.2. Prim's Algorithm
15.9.3. Kruskal’s Algorithm
15.9.4. Complexity Analysis
15.10. Backtracking
15.10.1. Backtracking
15.10.2. Alternative Techniques
Module 16. Intelligent Systems
16.1. Agent Theory
16.1.1. Concept History
16.1.2. Agent Definition
16.1.3. Agents in Artificial Intelligence
16.1.4. Agents in Software Engineering
16.2. Agent Architectures
16.2.1. The Reasoning Process of an Agent
16.2.2. Reactive Agents
16.2.3. Deductive Agents
16.2.4. Hybrid Agents
16.2.5. Comparison
16.3. Information and Knowledge
16.3.1. Difference between Data, Information and Knowledge
16.3.2. Data Quality Assessment
16.3.3. Data Collection Methods
16.3.4. Information Acquisition Methods
16.3.5. Knowledge Acquisition Methods
16.4. Knowledge Representation
16.4.1. The Importance of Knowledge Representation
16.4.2. Definition of Knowledge Representation According to Roles
16.4.3. Knowledge Representation Features
16.5. Ontologies
16.5.1. Introduction to Metadata
16.5.2. Philosophical Concept of Ontology
16.5.3. Computing Concept of Ontology
16.5.4. Domain Ontologies and Higher-Level Ontologies
16.5.5. How to Build an Ontology?
16.6. Ontology Languages and Ontology Creation Software
16.6.1. Triple RDF, Turtle and N
16.6.2. RDF Schema
16.6.3. OWL
16.6.4. SPARQL
16.6.5. Introduction to Ontology Creation Tools
16.6.6. Installing and Using Protégé
16.7. Semantic Web
16.7.1. Current and Future Status of the Semantic Web
16.7.2. Semantic Web Applications
16.8. Other Knowledge Representation Models
16.8.1. Vocabulary
16.8.2. Global Vision
16.8.3. Taxonomy
16.8.4. Thesauri
16.8.5. Folksonomy
16.8.6. Comparison
16.8.7. Mind Maps
16.9. Knowledge Representation Assessment and Integration
16.9.1. Zero-Order Logic
16.9.2. First-Order Logic
16.9.3. Descriptive Logic
16.9.4. Relationship between Different Types of Logic
16.9.5. Prolog: Programming Based on First-Order Logic
16.10. Semantic Reasoners, Knowledge-Based Systems and Expert Systems
16.10.1. Concept of Reasoner
16.10.2. Reasoner Applications
16.10.3. Knowledge-Based Systems
16.10.4. MYCIN: History of Expert Systems
16.10.5. Expert Systems Elements and Architecture
16.10.6. Creating Expert Systems
Module 17. Machine Learning and Data Mining
17.1. Introduction to Knowledge Discovery Processes and Basic Concepts of Machine Learning
17.1.1. Key Concepts of Knowledge Discovery Processes
17.1.2. Historical Perspective of Knowledge Discovery Processes
17.1.3. Stages of the Knowledge Discovery Processes
17.1.4. Techniques Used in Knowledge Discovery Processes
17.1.5. Characteristics of Good Machine Learning Models
17.1.6. Types of Machine Learning Information
17.1.7. Basic Learning Concepts
17.1.8. Basic Concepts of Unsupervised Learning
17.2. Data Exploration and Pre-Processing
17.2.1. Data Processing
17.2.2. Data Processing in the Data Analysis Flow
17.2.3. Types of Data
17.2.4. Data Transformations
17.2.5. Visualization and Exploration of Continuous Variables
17.2.6. Visualization and Exploration of Categorical Variables
17.2.7. Correlation Measures
17.2.8. Most Common Graphic Representations
17.2.9. Introduction to Multivariate Analysis and Dimensionality Reduction
17.3. Decision Trees
17.3.1. ID Algorithm
17.3.2. Algorithm C
17.3.3. Overtraining and Pruning
17.3.4. Result Analysis
17.4. Evaluation of Classifiers
17.4.1. Confusion Matrixes
17.4.2. Numerical Evaluation Matrixes
17.4.3. Kappa Statistic
17.4.4. ROC Curves
17.5. Classification Rules
17.5.1. Rule Evaluation Measures
17.5.2. Introduction to Graphic Representation
17.5.3. Sequential Overlay Algorithm
17.6. Neural Networks
17.6.1. Basic Concepts
17.6.2. Simple Neural Networks
17.6.3. Backpropagation Algorithm
17.6.4. Introduction to Recurrent Neural Networks
17.7. Bayesian Methods
17.7.1. Basic Probability Concepts
17.7.2. Bayes' Theorem
17.7.3. Naive Bayes
17.7.4. Introduction to Bayesian Networks
17.8. Regression and Continuous Response Models
17.8.1. Simple Linear Regression
17.8.2. Multiple Linear Regression
17.8.3. Logistic Regression
17.8.4. Regression Trees
17.8.5. Introduction to Support Vector Machines (SVM)
17.8.6. Goodness-of-Fit Measures
17.9. Clustering
17.9.1. Basic Concepts
17.9.2. Hierarchical Clustering
17.9.3. Probabilistic Methods
17.9.4. EM Algorithm
17.9.5. B-Cubed Method
17.9.6. Implicit Methods
17.10. Text Mining and Natural Language Processing (NLP)
17.10.1. Basic Concepts
17.10.2. Corpus Creation
17.10.3. Descriptive Analysis
17.10.4. Introduction to Feelings Analysis
Module 18. Neural Networks, the Basis of Deep Learning
18.1. Deep Learning
18.1.1. Types of Deep Learning
18.1.2. Applications of Deep Learning
18.1.3. Advantages and Disadvantages of Deep Learning
18.2. Surgery
18.2.1. Sum
18.2.2. Product
18.2.3. Transfer
18.3. Layers
18.3.1. Input Layer
18.3.2. Cloak
18.3.3. Output Layer
18.4. Layer Bonding and Operations
18.4.1. Architecture Design
18.4.2. Connection between Layers
18.4.3. Forward Propagation
18.5. Construction of the First Neural Network
18.5.1. Network Design
18.5.2. Establish the Weights
18.5.3. Network Training
18.6. Trainer and Optimizer
18.6.1. Optimizer Selection
18.6.2. Establishment of a Loss Function
18.6.3. Establishing a Metric
18.7. Application of the Principles of Neural Networks
18.7.1. Activation Functions
18.7.2. Backward Propagation
18.7.3. Parameter Adjustment
18.8. From Biological to Artificial Neurons
18.8.1. Functioning of a Biological Neuron
18.8.2. Transfer of Knowledge to Artificial Neurons
18.8.3. Establish Relations Between the Two
18.9. Implementation of MLP (Multilayer Perceptron) with Keras
18.9.1. Definition of the Network Structure
18.9.2. Model Compilation
18.9.3. Model Training
18.10. Fine Tuning Hyperparameters of Neural Networks
18.10.1. Selection of the Activation Function
18.10.2. Set the Learning Rate
18.10.3. Adjustment of Weights
Module 19. Deep Neural Networks Training
19.1. Gradient Problems
19.1.1. Gradient Optimization Techniques
19.1.2. Stochastic Gradients
19.1.3. Weight Initialization Techniques
19.2. Reuse of Pre-Trained Layers
19.2.1. Learning Transfer Training
19.2.2. Feature Extraction
19.2.3. Deep Learning
19.3. Optimizers
19.3.1. Stochastic Gradient Descent Optimizers
19.3.2. Optimizers Adam and RMSprop
19.3.3. Moment Optimizers
19.4. Programming of the Learning Rate
19.4.1. Automatic Learning Rate Control
19.4.2. Learning Cycles
19.4.3. Smoothing Terms
19.5. Overfitting
19.5.1. Cross Validation
19.5.2. Regularization
19.5.3. Evaluation Metrics
19.6. Practical Guidelines
19.6.1. Model Design
19.6.2. Selection of Metrics and Evaluation Parameters
19.6.3. Hypothesis Testing
19.7. Transfer Learning
19.7.1. Learning Transfer Training
19.7.2. Feature Extraction
19.7.3. Deep Learning
19.8. Data Augmentation
19.8.1. Image Transformations
19.8.2. Synthetic Data Generation
19.8.3. Text Transformation
19.9. Practical Application of Transfer Learning
19.9.1. Learning Transfer Training
19.9.2. Feature Extraction
19.9.3. Deep Learning
19.10. Regularization
19.10.1. L and L
19.10.2. Regularization by Maximum Entropy
19.10.3. Dropout
Module 20. Model Customization and Training with TensorFlow
20.1. TensorFlow
20.1.1. Use of the TensorFlow Library
20.1.2. Model Training with TensorFlow
20.1.3. Operations with Graphs in TensorFlow
20.2. TensorFlow and NumPy
20.2.1. NumPy Computing Environment for TensorFlow
20.2.2. Using NumPy Arrays with TensorFlow
20.2.3. NumPy Operations for TensorFlow Graphs
20.3. Model Customization and Training Algorithms
20.3.1. Building Custom Models with TensorFlow
20.3.2. Management of Training Parameters
20.3.3. Use of Optimization Techniques for Training
20.4. TensorFlow Features and Graphs
20.4.1. Functions with TensorFlow
20.4.2. Use of Graphs for Model Training
20.4.3. Grap Optimization with TensorFlow Operations
20.5. Loading and Preprocessing Data with TensorFlow
20.5.1. Loading Data Sets with TensorFlow
20.5.2. Preprocessing Data with TensorFlow
20.5.3. Using TensorFlow Tools for Data Manipulation
20.6. The Tf.data API
20.6.1. Using the Tf.data API for Data Processing
20.6.2. Construction of Data Streams with Tf.data
20.6.3. Using the Tf.data API for Model Training
20.7. The TFRecord Format
20.7.1. Using the TFRecord API for Data Serialization
20.7.2. TFRecord File Upload with TensorFlow
20.7.3. Using TFRecord Files for Model Training
20.8. Keras Preprocessing Layers
20.8.1. Using the Keras Preprocessing API
20.8.2. Preprocessing Pipelined Construction with Keras
20.8.3. Using the Keras Preprocessing API for Model Training
20.9. The TensorFlow Datasets Project
20.9.1. Using TensorFlow Datasets for Data Loading
20.9.2. Preprocessing Data with TensorFlow Datasets
20.9.3. Using TensorFlow Datasets for Model Training
20.10. Building a Deep Learning App with TensorFlow
20.10.1. Practical Applications
20.10.2. Building a Deep Learning App with TensorFlow
20.10.3. Model Training with TensorFlow
20.10.4. Use of the Application for the Prediction of Results
Module 21. Deep Computer Vision with Convolutional Neural Networks
21.1. The Visual Cortex Architecture
21.1.1. Functions of the Visual Cortex
21.1.2. Theories of Computational Vision
21.1.3. Models of Image Processing
21.2. Convolutional Layers
21.2.1. Reuse of Weights in Convolution
21.2.2. Convolution D
21.2.3. Activation Functions
21.3. Grouping Layers and Implementation of Grouping Layers with Keras
21.3.1. Pooling and Striding
21.3.2. Flattening
21.3.3. Types of Pooling
21.4. CNN Architecture
21.4.1. VGG Architecture
21.4.2. AlexNet Architecture
21.4.3. ResNet Architecture
21.5. Implementing a CNN ResNet using Keras
21.5.1. Weight Initialization
21.5.2. Input Layer Definition
21.5.3. Output Definition
21.6. Use of Pre-Trained Keras Models
21.6.1. Characteristics of Pre-Trained Models
21.6.2. Uses of Pre-Trained Models
21.6.3. Advantages of Pre-Trained Models
21.7. Pre-Trained Models for Transfer Learning
21.7.1. Learning by Transfer
21.7.2. Transfer Learning Process
21.7.3. Advantages of Transfer Learning
21.8. Deep Computer Vision Classification and Localization
21.8.1. Image Classification
21.8.2. Localization of Objects in Images
21.8.3. Object Detection
21.9. Object Detection and Object Tracking
21.9.1. Object Detection Methods
21.9.2. Object Tracking Algorithms
21.9.3. Tracking and Localization Techniques
21.10. Semantic Segmentation
21.10.1. Deep Learning for Semantic Segmentation
21.10.1. Edge Detection
21.10.1. Rule-Based Segmentation Methods
Module 22. Natural Language Processing (NLP) with Recurrent Neural Networks (RNN) and Attention
22.1. Text Generation using RNN
22.1.1. Training an RNN for Text Generation
22.1.2. Natural Language Generation with RNN
22.1.3. Text Generation Applications with RNN
22.2. Training Data Set Creation
22.2.1. Preparation of the Data for Training an RNN
22.2.2. Storage of the Training Dataset
22.2.3. Data Cleaning and Transformation
22.2.4. Sentiment Analysis
22.3. Classification of Opinions with RNN
22.3.1. Detection of Themes in Comments
22.3.2. Sentiment Analysis with Deep Learning Algorithms
22.4. Encoder-Decoder Network for Neural Machine Translation
22.4.1. Training an RNN for Machine Translation
22.4.2. Use of an Encoder-Decoder Network for Machine Translation
22.4.3. Improving the Accuracy of Machine Translation with RNNs
22.5. Attention Mechanisms
22.5.1. Application of Care Mechanisms in RNN
22.5.2. Use of Care Mechanisms to Improve the Accuracy of the Models
22.5.3. Advantages of Attention Mechanisms in Neural Networks
22.6. Transformer Models
22.6.1. Using Transformers Models for Natural Language Processing
22.6.2. Application of Transformers Models for Vision
22.6.3. Advantages of Transformers Models
22.7. Transformers for Vision
22.7.1. Use of Transformers Models for Vision
22.7.2. Image Data Preprocessing
22.7.3. Training a Transformers Model for Vision
22.8. Hugging Face Transformer Library
22.8.1. Using the Hugging Face's Transformers Library
22.8.2. Hugging Face´s Transformers Library Application
22.8.3. Advantages of Hugging Face´s Transformers Library
22.9. Other Transformers Libraries Comparison
22.9.1. Comparison Between Different Transformers Libraries
22.9.2. Use of the Other Transformers Libraries
22.9.3. Advantages of the Other Transformers Libraries
22.10. Development of an NLP Application with RNN and Attention Practical Applications
22.10.1. Development of a Natural Language Processing Application with RNN and Attention.
22.10.2. Use of RNN, Attention Mechanisms and Transformers Models in the Application
22.10.3. Evaluation of the Practical Application
Module 23. Autoencoders, GANs and Diffusion Models
23.1. Representation of Efficient Data
23.1.1. Dimensionality Reduction
23.1.2. Deep Learning
23.1.3. Compact Representations
23.2. PCA Realization with an Incomplete Linear Automatic Encoder
23.2.1. Training Process
23.2.2. Implementation in Python
23.2.3. Use of Test Data
23.3. Stacked Automatic Encoders
23.3.1. Deep Neural Networks
23.3.2. Construction of Coding Architectures
23.3.3. Use of Regularization
23.4. Convolutional Autoencoders
23.4.1. Design of Convolutional Models
23.4.2. Convolutional Model Training
23.4.3. Results Evaluation
23.5. Noise Suppression of Automatic Encoders
23.5.1. Filter Application
23.5.2. Design of Coding Models
23.5.3. Use of Regularization Techniques
23.6. Sparse Automatic Encoders
23.6.1. Increasing Coding Efficiency
23.6.2. Minimizing the Number of Parameters
23.6.3. Using Regularization Techniques
23.7. Variational Automatic Encoders
23.7.1. Use of Variational Optimization
23.7.2. Unsupervised Deep Learning
23.7.3. Deep Latent Representations
23.8. Generation of Fashion MNIST Images
23.8.1. Pattern Recognition
23.8.2. Image Generation
23.8.3. Deep Neural Networks Training
23.9. Generative Adversarial Networks and Diffusion Models
23.9.1. Content Generation from Images
23.9.2. Modeling of Data Distributions
23.9.3. Use of Adversarial Networks
23.10. Implementation of the Models
23.10.1. Practical Application
23.10.2. Implementation of the Models
23.10.3. Use of Real Data
23.10.4. Results Evaluation
Module 24. Natural Language Processing (NLP) with Recurrent Neural Networks (RNN) and Attention
24.1. Introduction to Bio-Inspired Computing
24.1.1. Introduction to Bio-Inspired Computing
24.2. Social Adaptation Algorithms
24.2.1. Bio-Inspired Computation Based on Ant Colonies
24.2.2. Variants of Ant Colony Algorithms
24.2.3. Particle Cloud Computing
24.3. Genetic Algorithms
24.3.1. General Structure
24.3.2. Implementations of the Major Operators
24.4. Space Exploration-Exploitation Strategies for Genetic Algorithms
24.4.1. CHC Algorithm
24.4.2. Multimodal Problems
24.5. Evolutionary Computing Models (I)
24.5.1. Evolutionary Strategies
24.5.2. Evolutionary Programming
24.5.3. Algorithms Based on Differential Evolution
24.6. Evolutionary Computation Models (II)
24.6.1. Evolutionary Models Based on Estimation of Distributions (EDA)
24.6.2. Genetic Programming
24.7. Evolutionary Programming Applied to Learning Problems
24.7.1. Rules-Based Learning
24.7.2. Evolutionary Methods in Instance Selection Problems
24.8. Multi-Objective Problems
24.8.1. Concept of Dominance
24.8.2. Application of Evolutionary Algorithms to Multi-Objective Problems
24.9. Neural Networks (I)
24.9.1. Introduction to Neural Networks
24.9.2. Practical Example with Neural Networks
24.10. Neural Networks (II)
24.10.1. Use Cases of Neural Networks in Medical Research
24.10.2. Use Cases of Neural Networks in Economics
24.10.3. Use Cases of Neural Networks in Artificial Vision
Module 25. Artificial Intelligence: Strategies and Applications
25.1. Financial Services
25.1.1. The Implications of Artificial Intelligence (AI) in Financial Services Opportunities and Challenges
25.1.2. Case Uses
25.1.3. Potential Risks Related to the Use of AI
25.1.4. Potential Future Developments/Uses of AI
25.2. Implications of Artificial Intelligence in the Healthcare Service
25.2.1. Implications of AI in the Healthcare Sector Opportunities and Challenges
25.2.2. Case Uses
25.3. Risks Related to the Use of AI in the Health Service
25.3.1. Potential Risks Related to the Use of AI
25.3.2. Potential Future Developments/Uses of AI
25.4. Retail
25.4.1. Implications of AI in Retail Opportunities and Challenges
25.4.2. Case Uses
25.4.3. Potential Risks Related to the Use of AI
25.4.4. Potential Future Developments/Uses of AI
25.5. Industry
25.5.1. Implications of AI in Industry Opportunities and Challenges
25.5.2. Case Uses
25.6. Potential Risks Related to the Use of AI in Industry
25.6.1. Case Uses
25.6.2. Potential Risks Related to the Use of AI
25.6.3. Potential Future Developments/Uses of AI
25.7. Public Administration
25.7.1. AI Implications for Public Administration Opportunities and Challenges
25.7.2. Case Uses
25.7.3. Potential Risks Related to the Use of AI
25.7.4. Potential Future Developments/Uses of AI
25.8. Educational
25.8.1. AI Implications for Education Opportunities and Challenges
25.8.2. Case Uses
25.8.3. Potential Risks Related to the Use of AI
25.8.4. Potential Future Developments/Uses of AI
25.9. Forestry and Agriculture
25.9.1. Implications of AI in Forestry and Agriculture Opportunities and Challenges
25.9.2. Case Uses
25.9.3. Potential Risks Related to the Use of AI
25.9.4. Potential Future Developments/Uses of AI
25.10 Human Resources
25.10.1. Implications of AI for Human Resources Opportunities and Challenges
25.10.2. Case Uses
25.10.3. Potential Risks Related to the Use of AI
25.10.4. Potential Future Developments/Uses of AI
Module 26. Artificial Intelligence Methods and Tools for Clinical Research
26.1. AI Technologies and Tools in Clinical Research
26.1.1. Using Machine Learning to Identify Patterns in Clinical Data
26.1.2. Development of Predictive Algorithms for Clinical Trials
26.1.3. Implementation of AI Systems to Improve Patient Recruitment
26.1.4. AI Tools for Real-Time Analysis of Research Data with Tableau
26.2. Statistical Methods and Algorithms in Clinical Trials
26.2.1. Application of Advanced Statistical Techniques for Clinical Data Analysis
26.2.2. Use of Algorithms for the Validation and Verification of Trial Results
26.2.3. Implementation of Regression and Classification Models in Clinical Studies
26.2.4. Analysis of Large Data Sets using Computational Statistical Methods
26.3. Design of Experiments and Analysis of Results
26.3.1. Strategies for the Efficient Design of Clinical Trials Using IA, with IBM Watson Health
26.3.2. AI Techniques for Analysis and Interpretation of Experimental Data
26.3.3. Optimization of Research Protocols Using AI Simulations
26.3.4. Evaluation of Efficacy and Safety of Treatments Using AI Models
26.4. Interpretation of Medical Images in Research Using AI through Aidoc
26.4.1. Development of AI Systems for the Automatic Detection of Pathologies in Images
26.4.2. Use of Deep Learning for Classification and Segmentation in Medical Images
26.4.3. AI Tools to Improve Accuracy in Image Diagnostics
26.4.4. Analysis of Radiological and Magnetic Resonance Imaging Using AI
26.5. Clinical Analysis and Biomedical Data Analysis
26.5.1. AI in Genomics and Proteomics Data Processing and Analysis DeepGenomics
26.5.2. Tools for the Integrated Analysis of Clinical and Biomedical Data
26.5.3. Use of AI to Identify Biomarkers in Clinical Research
26.5.4. Predictive Analysis of Clinical Outcomes Based on Biomedical Data
26.6. Advanced Data Visualization in Clinical Research
26.6.1. Development of Interactive Visualization Tools for Clinical Data
26.6.2. Use of AI in the Creation of Graphical Representations of Complex Data Microsoft Power BI
26.6.3. Visualization Techniques for Easy Interpretation of Research Results
26.6.4. Augmented and Virtual Reality Tools for Visualization of Biomedical Data
26.7. Natural Language Processing in Scientific and Clinical Documentation
26.7.1. Application of NLP for the Analysis of Scientific Literature and Clinical Records with Linguamatics
26.7.2. AI Tools for the Extraction of Relevant Information from Medical Texts
26.7.3. AI Systems for Summarizing and Categorizing Scientific Publications
26.7.4. Use of NLP to Identify Trends and Patterns in Clinical Documentation
26.8. Heterogeneous Data Processing in Clinical Research with Google Cloud Healthcare API and IBM Watson Health
26.8.1. AI Techniques for Integrating and Analyzing Data from Diverse Clinical Sources
26.8.2. Tools for the Management of Unstructured Clinical Data
26.8.3. AI Systems for Correlating Clinical and Demographic Data
26.8.4. Analysis of Multidimensional Data for Clinical Insights
26.9. Applications of Neural Networks in Biomedical Research
26.9.1. Use of Neural Networks for Disease Modeling and Treatment Prediction
26.9.2. Implementation of Neural Networks in Genetic Disease Classification
26.9.3. Development of Diagnostic Systems Based on Neural Networks
26.9.4. Application of Neural Networks in the Personalization of Medical Treatments
26.10. Predictive Modeling and its Impact on Clinical Research
26.10.1. Development of Predictive Models for the Anticipation of Clinical Outcomes
26.10.2. Use of AI in the Prediction of Side Effects and Adverse Reactions
26.10.3. Implementation of Predictive Models in the Optimization of Clinical Trials
26.10.4. Risk Analysis in Medical Treatments Using Predictive Modeling
Module 27. Biomedical Research with AI
27.1. Design and Implementation of Observational Studies with AI
27.1.1. Implementation of AI for the Selection and Segmentation of Populations in Studies
27.1.2. Use of Algorithms for Real-Time Monitoring of Observational Study Data
27.1.3. AI Tools for Identifying Patterns and Correlations in Observational Studies with Flatiron Health
27.1.4. Automation of the Data Collection and Analysis Process in Observational Studies
27.2. Validation and Calibration of Models in Clinical Research
27.2.1. AI Techniques to Ensure the Accuracy and Reliability of Clinical Models
27.2.2. Use of AI in the Calibration of Predictive Models in Clinical Research
27.2.3. Cross-Validation Methods Applied to Clinical Models Using IA, with KINME Analytics Platform
27.2.4. AI Tools for the Evaluation of Generalization of Clinical Models
27.3. Methods for Integration of Heterogeneous Data in Clinical Research
27.3.1. AI Techniques for Combining Clinical, Genomic and Environmental Data with DeepGenomics
27.3.2. Use of Algorithms to Manage and Analyze Unstructured Clinical Data
27.3.3. AI Tools for Clinical Data Standardization and Normalization with Informatica's Healthcare Data Management
27.3.4. AI Systems for Correlation of Different Types of Data in Research
27.4. Multidisciplinary Biomedical Data Integration using Flatiron Health’s OncologyCloud and AutoML
27.4.1. AI Systems to Combine Data from Different Biomedical Disciplines
27.4.2. Algorithms for Integrated Analysis of Laboratory and Clinical Data
27.4.3. AI Tools for Visualization of Complex Biomedical Data
27.4.4. Use of AI in the Creation of Holistic Health Models from Multidisciplinary Data
27.5. Deep Learning Algorithms in Biomedical Data Analysis
27.5.1. Implementation of Neural Networks in the Analysis of Genetic and Proteomic Data
27.5.2. Use of Deep Learning for Pattern Identification in Biomedical Data
27.5.3. Development of Predictive Models in Precision Medicine with Deep Learning
27.5.4. Application of AI in Advanced Biomedical Image Analysis Using Aidoc
27.6. Optimization of Research Processes with Automation
27.6.1. Automation of Laboratory Routines by Means of AI Systems with Beckman Coulter
27.6.2. Use of AI for Efficient Management of Resources and Time in Research
27.6.3. AI Tools for Optimization of Workflows in Clinical Research
27.6.4. Automated Systems for Tracking and Reporting Progress in Research
27.7. Simulation and Computational Modeling in Medicine with AI
27.7.1. Development of Computational Models to Simulate Clinical Scenarios
27.7.2. Use of AI for the Simulation of Molecular and Cellular Interactions with Schrödinger
27.7.3. AI Tools in the Creation of Predictive Disease Models with GNS Healthcare
27.7.4. Application of AI in the Simulation of Drug and Treatment Effects
27.8. Use of Virtual and Augmented Reality in Clinical Studies with Surgical Theater
27.8.1. Implementation of Virtual Reality for Training and Simulation in Medicine
27.8.2. Use of Augmented Reality in Surgical and Diagnostic Procedures
27.8.3. Virtual Reality Tools for Behavioral and Psychological Studies
27.8.4. Application of Immersive Technologies in Rehabilitation and Therapy
27.9. Data Mining Tools Applied to Biomedical Research
27.9.1. Use of Data Mining Techniques to Extract Knowledge from Biomedical Databases
27.9.2. Implementation of AI Algorithms to Discover Patterns in Clinical Data
27.9.3. AI Tools for Trend Identification in Large Data Sets with Tableau
27.9.4. Application of Data Mining in the Generation of Research Hypotheses
27.10. Development and Validation of Biomarkers with Artificial Intelligence
27.10.1. Use of AI for the Identification and Characterization of Innovative Biomarkers
27.10.2. Implementation of AI Models for the Validation of Biomarkers in Clinical Studies
27.10.3. AI tools in Correlating Biomarkers with Clinical Outcomes with Oncimmune
27.10.4. Application of AI in Biomarker Analysis for Personalized Medicine
Module 28. Practical Application of Artificial Intelligence in Clinical Research
28.1. Genomic Sequencing Technologies and Data Analysis with AI with DeepGenomics
28.1.1. Use of AI for Rapid and Accurate Analysis of Genetic Sequences
28.1.2. Implementation of Machine Learning Algorithms in the Interpretation of Genomic Data
28.1.3. AI Tools for Identification of Genetic Variants and Mutations
28.1.4. Development of AI Systems for Anomaly Detection in Medical Images
28.2. AI in Biomedical Images Analysis with Aidoc
28.2.1. Development of AI Systems for the Detection of Anomalies in Medical Images
28.2.2. Use of Deep Learning in the Interpretation of X-rays, MRI and CT Scans
28.2.3. AI Tools to Improve Accuracy in Diagnostic Imaging
28.2.4. Implementation of AI in Biomedical Image Classification and Segmentation
28.3. Robotics and Automation in Clinical Laboratories
28.3.1. Use of Robots for the Automation of Tests and Processes in Laboratories
28.3.2. Implementation of Automatic Systems for the Management of Biological Samples
28.3.3. Development of Robotic Technologies to Improve Efficiency and Accuracy in Clinical Analysis
28.3.4. AI Application in Optimization of Workflows in Laboratories with Optum
28.4. AI in the Personalization of Therapies and Precision Medicine
28.4.1. Development of AI Models for the Personalization of Medical Treatments
28.4.2. Use of Predictive Algorithms in the Selection of Therapies based on Genetic Profiling
28.4.3. AI Tools in the Adaptation of Drug Doses and Combinations with PharmGKB
28.4.4. Application of AI in the Identification of Effective Treatments for Specific Groups
28.5. Innovations in AI-Assisted Diagnostics using ChatGPT and Amazon Comprehend Medical
28.5.1. Implementation of AI Systems for Rapid and Accurate Diagnostics
28.5.2. Use of AI in Early Identification of Diseases through Data Analysis
28.5.3. Development of AI Tools for Clinical Test Interpretation
28.5.4. Application of AI in Combining Clinical and Biomedical Data for Comprehensive Diagnostics
28.6. AI Applications in Microbiome and Microbiology Studies with Metabiomics
28.6.1. Use of AI in the Analysis and Mapping of the Human Microbiome
28.6.2. Implementation of Algorithms to Study the Relationship between Microbiome and Diseases
28.6.3. AI Tools in the Identification of Patterns in Microbiological Studies
28.6.4. Application of AI in Microbiome-Based Therapeutics Research
28.7. Wearables and Remote Monitoring in Clinical Trials
28.7.1. Development of Wearable Devices with AI for Continuous Health Monitoring with FitBit
28.7.2. Use of AI in the Interpretation of Data Collected by Wearables
28.7.3. Implementation of Remote Monitoring Systems in Clinical Trials
28.7.4. Application of AI in the Prediction of Clinical Events through Wearable Data
28.8. AI in Clinical Trial Management with Oracle Health Sciences
28.8.1. Use of AI Systems for Optimization of Clinical Trial Management
28.8.2. Implementation of AI in the Selection and Monitoring of Participants
28.8.3. AI Tools for Analysis of Clinical Trial Data and Results
28.8.4. Application of AI to Improve Trial Efficiency and Reduce Trial Costs
28.9. Development of AI-Assisted Vaccines and Treatments with Benevolent AI
28.9.1. Use of AI to Accelerate Vaccine Development
28.9.2. Implementation of Predictive Models in the Identification of Potential Treatments
28.9.3. AI Tools to Simulate Responses to Vaccines and Drugs
28.9.4. Application of AI in the Personalization of Vaccines and Therapies
28.10. AI Applications in Immunology and Immune Response Studies
28.10.1. Development of AI Models to Understand Immunological Mechanisms with Immuneering
28.10.2. Use of AI in the Identification of Patterns in Immune Responses
28.10.3. Implementation of AI in Autoimmune Disorders Research
28.10.4. Application of AI in the Design of Personalized Immunotherapies
Module 29. Big Data Analytics and Machine Learning in Clinical Research
29.1. Big Data in Clinical Research: Concepts and Tools
29.1.1. The Explosion of Data in the Field of Clinical Research
29.1.2. Concept of Big Data and Main Tools
29.1.3. Applications of Big Data in Clinical Research
29.2. Data Mining in Clinical and biomedical Records with KNIME and Python
29.2.1. Main Methodologies for Data Mining
29.2.2. Data Integration of Clinical and Biomedical Registry Data
29.2.3. Detection of Patterns and Anomalies in Clinical and Biomedical Records
29.3. Machine Learning Algorithms in Biomedical Research with KNIME and Python
29.3.1. Classification Techniques in Biomedical Research
29.3.2. Regression Techniques in Biomedical Research
29.3.3. Unsupervised Techniques in Biomedical Research
29.4. Predictive Analytics Techniques in Clinical Research with KNIME and Python
29.4.1. Classification Techniques in Clinical Research
29.4.2. Regression Techniques in Clinical Research
29.4.3. Deep Learning in Clinical Research
29.5. AI Models in Epidemiology and Public Health with KNIME and Python
29.5.1. Classification Techniques for Epidemiology and Public Health
29.5.2. Regression Techniques for Epidemiology and Public Health
29.5.3. Unsupervised Techniques for Epidemiology and Public Health
29.6. Analysis of Biological Networks and Disease Patterns with KNIME and Python
29.6.1. Exploration of Interactions in Biological Networks for the Identification of Disease Patterns
29.6.2. Integration of Omics Data in Network Analysis to Characterize Biological Complexities
29.6.3. Application of Machine Learning Algorithms for the Discovery of Disease Patterns
29.7. Development of Tools for Clinical Prognosis with Workflow and Python Platforms
29.7.1. Creation of Innovative Clinical Prognostic Tools based on Multidimensional Data
29.7.2. Integration of Clinical and Molecular Variables in the Development of Prognostic Tools
29.7.3. Evaluating the Effectiveness of Prognostic Tools in Diverse Clinical Contexts
29.8. Advanced Visualization and Communication of Complex Data with Tools such as PowerBI and Python
29.8.1. Use of Advanced Visualization Techniques to Represent Complex Biomedical Data
29.8.2. Development of Effective Communication Strategies to Present Results of Complex Analyses
29.8.3. Implementation of Interactivity Tools in Visualizations to Enhance Understanding
29.9. Data Security and Challenges in Big Data Management
29.9.1. Addressing Data Security Challenges in the Context of Biomedical Big Data
29.9.1. Strategies for Privacy Protection in the Management of Large Biomedical Datasets
29.9.3. Implementation of Security Measures to Mitigate Risks in the Handling of Sensitive Data
29.10. Practical Applications and Case Studies on Biomedical Big Data
29.10.1. Exploration of Successful Cases in the Implementation of Biomedical Big Data in Clinical Research
29.10.2. Development of Practical Strategies for the Application of Big Data in Clinical Decision-Making
29.10.3. Evaluation of Impact and Lessons Learned through Case Studies in the Biomedical Field
Module 30. Ethical, Legal and Future Aspects of Artificial Intelligence in Clinical Research
30.1. Ethics in the Application of AI in Clinical Research
30.1.1. Ethical Analysis of AI-Assisted Decision Making in Clinical Research Settings
30.1.2. Ethics in the Use of AI Algorithms for Participant Selection in Clinical Trials
30.1.3. Ethical Considerations in the Interpretation of Results Generated by AI Systems in Clinical Research
30.2. Legal and Regulatory Considerations in Biomedical AI
30.2.1. Analysis of Legal Regulations in the Development and Application of AI Technologies in the Biomedical Field
30.2.2. Assessment of Compliance with Specific Regulations to Ensure the Safety and Efficacy of AI-Based Solutions
30.2.3. Addressing Emerging Regulatory Challenges Associated with the Use of AI in Biomedical Research
30.3. Informed Consent and Ethical Aspects in the Use of Clinical Data
30.3.1. Development of Strategies to Ensure Effective Informed Consent in AI Projects
30.3.2. Ethics in the Collection and Use of Sensitive Clinical Data in the Context of AI-Driven Research
30.3.3. Addressing Ethical Issues Related to Ownership and Access to Clinical Data in Research Projects
30.4. AI and Liability in Clinical Research
30.4.1. Evaluation of Ethical and Legal Accountability in the Implementation of AI Systems in Clinical Research Protocols
30.4.2. Development of Strategies to Address Potential Adverse Consequences of the Application of AI in Biomedical Research
30.4.3. Ethical Considerations in the Active Participation of AI in Clinical Research Decision Making
30.5. Impact of AI on Equity and Access to Health Care
30.5.1. Evaluation of the Impact of AI Solutions on Equity in Clinical Trial Participation
30.5.2. Development of Strategies to Improve Access to AI Technologies in Diverse Clinical Settings
30.5.3. Ethics in the Distribution of Benefits and Risks Associated with the Application of AI in Health Care
30.6. Privacy and Data Protection in Research Projects
30.6.1. Ensuring the Privacy of Participants in Research Projects Involving the Use of AI
30.6.2. Development of Policies and Practices for Data Protection in Biomedical Research
30.6.3. Addressing Specific Privacy and Security Challenges in the Handling of Sensitive Data in the Clinical Environment
30.7. AI and Sustainability in Biomedical Research
30.7.1. Assessment of the Environmental Impact and Resources Associated with the Implementation of AI in Biomedical Research
30.7.2. Development of Sustainable Practices in the Integration of AI Technologies into Clinical Research Projects
30.7.3. Ethics in Resource Management and Sustainability in the Adoption of AI in Biomedical Research
30.8. Auditing and Explainability of AI Models in the Clinical Setting
30.8.1. Development of Audit Protocols for Assessing the Reliability and Accuracy of AI Models in Clinical Research
30.8.2. Ethics in Explainability of Algorithms to Ensure Understanding of Decisions Made by AI Systems in Clinical Contexts
30.8.3. Addressing Ethical Challenges in the Interpretation of AI Model Results in Biomedical Research
30.9. Innovation and Entrepreneurship in the Field of Clinical AI
30.9.1. Responsible Innovation Ethics in Developing AI Solutions for Clinical Applications
30.9.2. Development of Ethical Business Strategies in the Field of Clinical AI
30.9.3. Ethical Considerations in the Commercialization and Adoption of AI Solutions in the Clinical Sector
30.10. Ethical Considerations in International Collaboration in Clinical Research
30.10.1. Development of Ethical and Legal Arrangements for International Collaboration in AI-Driven Research Projects
30.10.2. Ethics in Multi-Institutional and Multi-Country Involvement in Clinical Research using AI Technologies
30.10.3. Addressing Emerging Ethical Challenges Associated with Global Collaboration in Biomedical Research
A reference teaching team will introduce you to the latest advances in Deep Computer Vision with Convolutional Neural Networks”
Advanced Master's Degree MBA in Artificial Intelligence in Clinical Research
Advance the field of clinical research and medicine with the unique Advanced Master's Degree MBA created by TECH Global University of Technology. This pioneering program, delivered in an online mode, combines fundamental knowledge of clinical research with advanced AI skills, preparing you to lead in the innovation and advancement of healthcare. Through the study plan, you'll explore the fundamental principles of clinical research, including study design, data collection, statistical analysis and interpretation of results. You will learn how clinical trials are conducted and what regulatory requirements must be met. In addition, you'll discover how AI is transforming clinical research and medical practice. You will explore applications such as medical image analysis, patient outcome prediction and treatment personalization, enabling you to apply machine learning algorithms to improve the accuracy and efficiency of research processes.
Earn a Advanced Master's Degree MBA in Artificial Intelligence in Clinical Research
With a unique combination of theoretical knowledge and practical skills, we'll equip you to address the most pressing challenges in medical research and improve the health and well-being of people around the world. As you advance through the program, you'll explore the ethical and regulatory aspects of clinical research, including informed consent, protection of patient privacy, and compliance with FDA and other regulatory agencies. You will learn how to design studies that meet the highest ethical and regulatory standards. From this, you will develop skills in data analysis and interpretation of clinical research results, enabling you to use statistical tools and data analysis software to extract meaningful information from clinical data sets and communicate findings clearly and effectively. Finally, you will develop competencies in clinical trial design and management, learning how to plan, execute and supervise research studies in the clinical setting. Join us and make a difference in clinical research today!