University certificate
The world's largest faculty of medicine”
Description
Thanks to this 100% online Postgraduate diploma, you will handle the most innovative techniques of Artificial Intelligence to increase the accuracy of imaging findings and optimize clinical diagnoses”
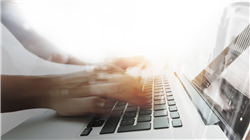
A recent report by the World Health Organization highlights that the use of Artificial Intelligence in the healthcare field has allowed optimizing the early detection rate of Breast Cancer Tumors by 95%. This fact highlights the potential of these emerging technologies for the early detection of a wide range of pathologies. Therefore, it is important that professionals update their knowledge regularly to incorporate the latest advances in techniques such as Machine Learning into their clinical practice. Only in this way will experts be able to increase the accuracy of their clinical diagnoses and design the most appropriate individualized treatments to ensure optimal patient recovery.
In order to facilitate this task, TECH has created a pioneering program in Image Analysis with Artificial Intelligence for Medical Diagnosis. Conceived by references in this field, the academic itinerary will focus on aspects ranging from the use of Deep Learning in Radiology or the development of graphic interfaces for the exploration of 3D images to Natural Language Processing with Nuance PowerScribe 360. In this way, graduates will develop advanced clinical skills to employ algorithms in Biomedical Imaging to detect subtle features. In addition, the course materials will analyze the most effective simulation and computational modeling techniques for planning complex surgical procedures.
In terms of methodology, TECH provides a 100% online environment that adapts to the needs of busy physicians looking to experience a leap in quality in their professional careers. In addition, it employs its disruptive Relearning system, based on the reiteration of key concepts to facilitate the updating of knowledge. In this sense, the only thing graduates will need is an electronic device with an Internet connection to access the Virtual Campus. There they will find a library of different multimedia resources such as explanatory videos, specialized readings or interactive summaries.
This program gives you the opportunity to update your knowledge in a real scenario, with the maximum scientific rigor of an institution at the forefront of technology"
This Postgraduate diploma in Image Analysis with Artificial Intelligence for Medical Diagnosis contains the most complete and up-to-date scientific program on the market. The most important features include:
- Development of practical cases presented by experts in Artificial Intelligence
- The graphic, schematic and eminently practical contents with which it is conceived gather scientific and practical information on those disciplines that are indispensable for professional practice
- Practical exercises where self-assessment can be used to improve learning
- Its special emphasis on innovative methodologies
- Theoretical lessons, questions to the expert, debate forums on controversial topics, and individual reflection assignments
- Content that is accessible from any fixed or portable device with an Internet connection
You will gain profound knowledge in the process of Data Mining with Radiomics, which will allow you to identify risk factors that manifest the probability of developing pathologies such as Diabetes”
The program’s teaching staff includes professionals from the field who contribute their work experience to this educational program, as well as renowned specialists from leading societies and prestigious universities.
The multimedia content, developed with the latest educational technology, will provide the professional with situated and contextual learning, i.e., a simulated environment that will provide immersive education programmed to learn in real situations.
This program is designed around Problem-Based Learning, whereby the professional must try to solve the different professional practice situations that arise during the course. For this purpose, students will be assisted by an innovative interactive video system created by renowned and experienced experts.
Are you looking to incorporate state-of-the-art methods to reduce noise in imaging tests into your daily clinical practice? Achieve it through this university program"
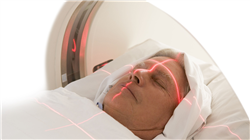
With TECH's revolutionary Relearning methodology, you will integrate all the knowledge in an optimal way without the need to resort to traditional techniques such as memorization"
Syllabus
This program has been designed by references in the field of Image Analysis with Artificial Intelligence for Medical Diagnosis. The academic itinerary will delve into the management of sophisticated tools such as Deep Learning, Convolutional Neural Networks or specialized software in the processing of Biomedical Images. Therefore, graduates will develop advanced competencies to optimize their clinical diagnoses and offer more personalized treatments to patients. In addition, the syllabus will delve into the advantages of Artificial Intelligence to accelerate the vaccine process and reduce the response time to emergencies to ensure the recovery of users.
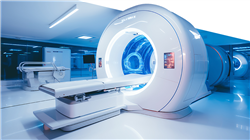
You will make the earliest and most accurate clinical diagnoses thanks to the predictive capabilities of Artificial Intelligence”
Module 1. Artificial Intelligence Innovations in Diagnostic Imaging
1.1. Artificial Intelligence Technologies and Tools in Diagnostic Imaging with IBM Watson Imaging Clinical Review
1.1.1. Leading Software Platforms for Medical Image Analysis
1.1.2. Radiology-Specific Deep Learning Tools
1.1.3. Innovations in Hardware to Accelerate Image Processing
1.1.4. Integration of Artificial Intelligence Systems in Existing Hospital Infrastructures
1.2. Statistical Methods and Algorithms for Medical Image Interpretation with DeepMind AI for Breast Cancer Analysis
1.2.1. Image Segmentation Algorithms
1.2.2. Classification and Detection Techniques in Medical Images
1.2.3. Use of Convolutional Neural Networks in Radiology
1.2.4. Noise Reduction and Image Quality Improvement Methods
1.3. Design of Experiments and Analysis of Results in Diagnostic Imaging with Google Cloud Healthcare API
1.3.1. Design of Validation Protocols for Artificial Intelligence Algorithms
1.3.2. Statistical Methods for Comparing the Performance of Artificial Intelligence and Radiologists
1.3.3. Setting Up Multicenter Studies for Artificial Intelligence Testing
1.3.4. Interpretation and Presentation of Performance Test Results
1.4. Detection of Subtle Patterns in Low-Resolution Images
1.4.1. Artificial Intelligence for Early Diagnosis of Neurodegenerative Diseases
1.4.2. Artificial Intelligence Applications in Interventional Cardiology
1.4.3. Use of Artificial Intelligence for the Optimization of Imaging Protocols
1.5. Biomedical Image Analysis and Processing
1.5.1. Pre-Processing Techniques to Improve Automatic Interpretation
1.5.2. Texture and Pattern Analysis in Histological Images
1.5.3. Extraction of Clinical Features from Ultrasound Images
1.5.4. Methods for Longitudinal Analysis of Images in Clinical Studies
1.6. Advanced Data Visualization in Diagnostic Imaging with OsiriX MD
1.6.1. Development of Graphical Interfaces for 3D Image Exploration
1.6.2. Tools for Visualization of Temporal Changes in Medical Images
1.6.3. Augmented Reality Techniques for the Teaching of Anatomy
1.6.4. Real-Time Visualization Systems for Surgical Procedures
1.7. Natural Language Processing in Medical Image Documentation and Reporting with Nuance PowerScribe 360
1.7.1. Automatic Generation of Radiological Reports
1.7.2. Extraction of Relevant Information from Electronic Medical Records
1.7.3. Semantic Analysis for the Correlation of Imaging and Clinical Findings
1.7.4. Image Search and Retrieval Tools Based on Textual Descriptions
1.8. Integration and Processing of Heterogeneous Data in Medical Imaging
1.8.1. Fusion of Imaging Modalities for Complete Diagnostics
1.8.2. Integration of Laboratory and Genetic Data in the Image Analysis
1.8.3. Systems for Handling Large Volumes of Imaging Data
1.8.4. Strategies for Normalization of Datasets from Multiple Sources
1.9. Applications of Neural Networks in Medical Image Interpretation with Zebra Medical Vision
1.9.1. Use of Generative Networks for the Creation of Synthetic Medical Images
1.9.2. Neural Networks for Automatic Tumor Classification
1.9.3. Deep Learning for the Analysis of Time Series in Functional Imaging
1.9.4. Fitting of Pre-Trained Models on Specific Medical Image Datasets
1.10. Predictive Modeling and its Impact on Diagnostic Imaging with IBM Watson Oncology
1.10.1. Predictive Models for Risk Assessment in Oncology Patients
1.10.2. Predictive Tools for Chronic Disease Follow-Up
1.10.3. Survival Analysis Using Medical Imaging Data
1.10.4. Prediction of Disease Progression using Machine Learning Techniques
Module 2. Advanced AI Applications in Medical Imaging Studies and Analysis
2.1. Design and Execution of Observational Studies using Artificial Intelligence in Medical Imaging with Flatiron Health
2.1.1. Criteria for the Selection of Populations in Artificial Intelligence Observational Studies
2.1.2. Methods for Controlling Confounding Variables in Imaging Studies
2.1.3. Strategies for Long-Term Follow-Up in Observational Studies
2.1.4. Analysis of Results and Validation of Artificial Intelligence Models in Real Clinical Settings
2.2. Validation and Calibration of AI Models in Image Interpretation with Arterys Cardio AI
2.2.1. Cross-Validation Techniques Applied to Diagnostic Imaging Models
2.2.2. Methods for Probability Calibration in AI Predictions
2.2.3. Performance Standards and Accuracy Metrics for AI Evaluation
2.2.4. Implementation of Robustness Testing in Different Populations and Conditions
2.3. Methods of Integrating Imaging Data with other Biomedical Sources
2.3.1. Data Fusion Techniques to Improve Image Interpretation
2.3.2. Joint Analysis of Images and Genomic Data for Accurate Diagnoses
2.3.3. Integration of Clinical and Laboratory Information in Artificial Intelligence Systems
2.3.4. Development of User Interfaces for Integrated Visualization of Multidisciplinary Data
2.4. Use of Medical Imaging Data in Multidisciplinary Research with Enlitic Curie
2.4.1. Interdisciplinary Collaboration for Advanced Image Analysis
2.4.2. Application of Artificial Intelligence Techniques from other Fields in Diagnostic Imaging
2.4.3. Challenges and Solutions in the Management of Large and Heterogeneous Data
2.4.4. Case Studies of Successful Multidisciplinary Applications
2.5. Specific Deep Learning Algorithms for Medical Imaging with Aidoc
2.5.1. Development of Image-Specific Neural Network Architectures
2.5.2. Optimization of Hyperparameters for Medical Imaging Models
2.5.3. Transfer of Learning and its Applicability in Radiology
2.6. Challenges in the Interpretation and Visualization of Features Learned by Deep Models
2.6.1. Optimization of the Interpretation of Medical Images by Automation with Viz.ai
2.6.2. Automation of Diagnostic Routines for Operational Efficiency
2.6.3. Early Warning Systems for Anomaly Detection
2.6.4. Reduction of Radiologists' Workload through Artificial Intelligence Tools
2.6.5. Impact of Automation on the Accuracy and Speed of Diagnostics
2.7. Simulation and Computational Modeling in Diagnostic Imaging
2.7.1. Simulations for Training and Validation of Artificial Intelligence Algorithms
2.7.2. Modeling of Diseases and their Representation in Synthetic Images
2.7.3. Use of Simulations for Treatment and Surgery Planning
2.7.4. Advances in Computational Techniques for Real-Time Image Processing
2.8. Virtual and Augmented Reality in Medical Image Visualization and Analysis
2.8.1. Virtual Reality Applications for Diagnostic Imaging Education
2.8.2. Use of Augmented Reality in Image-Guided Surgical Procedures
2.8.3. Advanced Visualization Tools for Therapeutic Planning
2.8.4. Development of Immersive Interfaces for the Review of Radiological Studies
2.9. Data Mining Tools Applied to Diagnostic Imaging with Radiomics
2.9.1. Techniques for Data Mining of Large Medical Image Repositories
2.9.2. Pattern Analysis Applications for Image Data Collections
2.9.3. Biomarker Identification through Image Data Mining
2.9.4. Integration of Data Mining and Machine Learning for Clinical Discovery
2.10. Development and Validation of Biomarkers using Image Analysis with Oncimmune
2.10.1. Strategies to Identify Imaging Biomarkers in Various Diseases
2.10.2. Clinical Validation of Imaging Biomarkers for Diagnostic Use
2.10.3. Impact of Imaging Biomarkers on Treatment Personalization
2.10.4. Emerging Technologies in the Detection and Analysis of Biomarkers through Artificial Intelligence
Module 3. Personalization and Automation in Medical Diagnosis through Artificial Intelligence
3.1. Application of Artificial Intelligence in Genomic Sequencing and Correlation with Imaging Findings using Fabric Genomics
3.1.1. Artificial Intelligence Techniques for the Integration of Genomic and Imaging Data
3.1.2. Predictive Models to Correlate Genetic Variants with Pathologies Visible in Images
3.1.3. Development of Algorithms for the Automatic Analysis of Sequences and their Representation in Images
3.1.4. Case Studies on the Clinical Impact of Genomics-Imaging Fusion
3.2. Advances in Artificial Intelligence for the Detailed Analysis of Biomedical Images with PathAI
3.2.1. Innovations in Image Processing and Analysis Techniques at the Cellular Level
3.2.2. Application of Artificial Intelligence for Resolution Enhancement in Microscopy Images
3.2.3. Deep Learning Algorithms Specialized in the Detection of Submicroscopic Patterns
3.2.4. Impact of Advances in Artificial Intelligence on Biomedical Research and Clinical Diagnosis
3.3. Automation in Medical Image Acquisition and Processing with Butterfly Network
3.3.1. Automated Systems for the Optimization of Image Acquisition Parameters
3.3.2. Artificial Intelligence in the Management and Maintenance of Imaging Equipment
3.3.3. Algorithms for Real-Time Processing of Images during Medical Procedures
3.3.4. Successful Cases in the Implementation of Automated Systems in Hospitals and Clinics
3.4. Personalization of Diagnoses using Artificial Intelligence and Precision Medicine with Tempus AI
3.4.1. Artificial Intelligence Models for Personalized Diagnostics Based on Genetic and Imaging Profiles
3.4.2. Strategies for the Integration of Clinical and Imaging Data in Therapeutic Planning
3.4.3. Impact of Precision Medicine on Clinical Outcomes Via AI
3.4.4. Ethical and Practical Challenges in Implementing Personalized Medicine
3.5. Innovations in AI-Assisted Diagnostics with Caption Health
3.5.1. Development of New Artificial Intelligence Tools for the Early Detection of Diseases
3.5.2. Advances in Artificial Intelligence Algorithms for the Interpretation of Complex Pathologies
3.5.3. Integration of AI-Assisted Diagnostics in Routine Clinical Practice
3.5.4. Evaluation of the Effectiveness and Acceptance of Diagnostic Artificial Intelligence by Healthcare Professionals
3.6. Applications of Artificial Intelligence in Microbiome Image Analysis with DayTwo AI
3.6.1. Artificial Intelligence Techniques for Image Analysis in Microbiome Studies
3.6.2. Correlation of Microbiome Imaging Data with Health Indicators
3.6.3. Impact of Microbiome Findings on Therapeutic Decisions
3.6.4. Challenges in the Standardization and Validation of Microbiome Imaging
3.7. Use of Wearables to Improve the Interpretation of Diagnostic Images with AliveCor
3.7.1. Integration of Wearable Data with Medical Images for Complete Diagnostics
3.7.2. AI Algorithms for the Analysis of Continuous Data and its Representation in Images
3.7.3. Technological Innovations in Wearable Devices for Health Monitoring
3.7.4. Case Studies on Improving Quality of Life Through Wearables and Imaging Diagnostics
3.8. Management of Diagnostic Imaging Data in Clinical Trials using Artificial Intelligence
3.8.1. AI Tools for the Efficient Management of Large Volumes of Image Data
3.8.2. Strategies to Ensure the Quality and Integrity of Data in Multicenter Studies
3.8.3. Artificial Intelligence Applications for Predictive Analytics in Clinical Trials
3.8.4. Challenges and Opportunities in the Standardization of Imaging Protocols in Global Trials
3.9. Development of Treatments and Vaccines Assisted by Advanced AI Diagnostics
3.9.1. Use of Artificial Intelligence to Design Personalized Treatments Based on Imaging and Clinical Data
3.9.2. Artificial Intelligence Models in the Accelerated Development of Vaccines Supported by Diagnostic Imaging
3.9.3. Evaluation of the Effectiveness of Treatments by Means of Image Monitoring
3.9.4. Impact of Artificial Intelligence in the Reduction of Time and Costs in the Development of New Therapies
3.10. AI Applications in Immunology and Immune Response Studies with ImmunoMind
3.10.1. AI Models for the Interpretation of Images Related to the Immune Response
3.10.2. Integration of Imaging Data and Immunological Analysis for Accurate Diagnosis
3.10.3. Development of Imaging Biomarkers for Autoimmune Diseases
3.10.4. Advances in the Personalization of Immunological Treatments through the Use of Artificial Intelligence
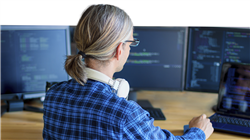
This academic itinerary is exclusive to TECH and you will be able to develop it at your own pace thanks to its 100% online Relearning methodology"
Postgraduate Diploma in Image Analysis with Artificial Intelligence for Medical Diagnosis
Artificial intelligence (AI) is transforming the world of medicine, especially in the field of diagnostic imaging. Advanced AI techniques make it possible to analyze medical images with a precision and speed that surpasses human capabilities, revolutionizing the early detection of diseases and improving patient outcomes. To prepare healthcare professionals in this area, TECH Global University offers this Postgraduate Diploma in Image Analysis with Artificial Intelligence for Medical Diagnosis. A 100% online program that will equip you with the necessary skills to make the most of AI capabilities in the clinical setting. The study plan covers from the fundamentals of medical image analysis, to the most advanced applications of AI algorithms in the diagnosis of pathologies. Through this experience, you will learn how to handle machine learning and deep learning tools applied to images, acquiring key skills to interpret automated results and improve clinical decision making. In addition, you will learn how artificial intelligence is being used to optimize radiology workflows, improving efficiency and reducing margins of error in the detection of anomalies.
Develop key competencies in the use of AI for medical diagnosis
The demand for professionals capable of interpreting and applying AI-based tools in X-ray, MRI and CT scans has grown exponentially. TECH offers you this fully online course, which will give you the opportunity to update and improve your skills without abandoning your job responsibilities. As you advance, you will address essential topics such as the integration of machine learning and deep learning algorithms for image analysis, allowing you to learn how to specialize and apply predictive models to identify patterns associated with various pathologies. In addition, you will study the processes of segmentation and classification of medical images, which are essential for accurate detection of diseases such as cancer, cardiovascular diseases and neurological pathologies. Take advantage of this opportunity to advance your career and make a difference in modern medical diagnosis - enroll now!