University certificate
The world's largest faculty of information technology”
Why study at TECH?
With this MBA in Data Science Management you will be the best possible candidate for the management of any team, bringing with you a unique analytical and technical point of view’’
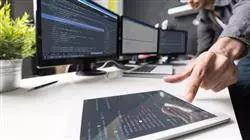
Companies which are up-to-date with the reality of the digital world we live in require multidisciplinary professionals. These teams are generally made up of highly qualified individuals who require an even more specialized level of management which has been adapted according to the level of their expertise. This MBA in Data Science Management covers this niche market, providing students with a unique and useful set of skills with which to lead teams. Using data science and deep analytics, the student will be able to make quick decisions from a global perspective with a comprehensive understanding of the reality that surrounds these complex and changing business environments.
The training material covers all aspects necessary to successfully lead, from an analytical perspective, the management, manipulation and interpretation of collected data; the optimal devices and platforms for data management; data mining, data graphing and data-driven predictive models; and finally, leadership and effective communication with large groups at the workplace. In addition to all of the above, there are other complementary, more technical skills that make this a wide-ranging and comprehensive course.
Moreover, the students will have the total freedom to take on this program at their own pace, since it is a 100% online qualification, without fixed schedules or the obligation to attend in person. The didactic material is accessible at all times and the student can adapt the learning experience to suit their personal and professional obligations.
With the skill set provided by this MBA in Data Science Management ,you will have everything it takes to launch your career to new heights and achieve your goals’’
This MBA in Data Science Management contains the most complete and up-to-date educational program on the market. The most important features include:
- The development of case studies presented by experts in leadership and data analytics
- The graphic, schematic, and eminently practical contents with which they are created, provide scientific and practical information on the disciplines that are essential for professional practice
- Practical exercises where the self-assessment process can be carried out to improve learning
- Special emphasis on innovative methodologies in the field of data science
- Theoretical lessons, questions to the expert, debate forums on controversial topics, and individual reflection work
- Content that is accessible from any fixed or portable device with an Internet connection
Leaders with the greatest skills and knowledge are the ones who can make a difference in a contested and competitive business environment.Make a difference and succeed where others have failed with leadership and data science skills’’
The faculty includes professionals belonging to the field of business management and data science, who bring to this program their vast work experience, as well as recognized specialists from prestigious societies and universities of reference.
The multimedia content, developed with the latest educational technology, will provide the professional with situated and contextual learning, i.e., a simulated environment that will deliver an immersive learning experience, programmed to train in real situations.
This program is designed around Problem-Based Learning, whereby the student must try to solve the different professional practice situations that arise during the course. For this purpose, the professional will be assisted by an innovative interactive video system created by renowned and experienced experts.
This is your moment to reach the zenith of your professional career. Specialize with this MBA in Data Science Management and apply for the jobs you've always dreamed of"
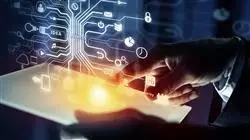
TECH offers you the possibility to study at your own pace. You have a unique opportunity to give your resume a unique touch of class and stand out from the rest of the competition"
Syllabus
The MBA in Data Science Management is composed of 19 modules made up of a variety of different topics and subtopics, all the information is compiled in a precise and clear way so that the students will not have any difficulties when it comes to carrying out their studies. During the course, the students will learn innovative work methodologies, different forms of data management and storage, as well as how to solve and mediate any possible conflicts that may arise in the workplace, alongside additional key information that will also prove useful to their professional career in management.
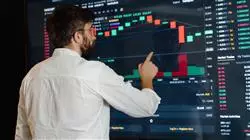
This MBA in Data Science Management gives you the opportunity to acquire the best knowledge in the most concise and precise way possible’’
Module 1. Data Analytics in the Business Organization
1.1. Business Analysis
1.1.1. Business Analysis
1.1.2. Data Structure
1.1.3. Phases and Elements
1.2. Data Analytics in the Company
1.2.1. Scorecards and KPIs by Departments
1.2.2. Operational, Tactical and Strategic Reporting
1.2.3. Data Analytics Applied to Each Department
1.2.3.1. Marketing and Communication
1.2.3.2. Commercial
1.2.3.3. Customer Service
1.2.3.4. Purchasing
1.2.3.5. Administration
1.2.3.6. Human Resources
1.2.3.7. Production
1.2.3.8. IT
1.3. Marketing and Communication
1.3.1. KPIs to be Measured, Applications and Benefits
1.3.2. Marketing Systems and Data Warehouse
1.3.3. Implementation of a Data Analytics Marketing Framework
1.3.4. Marketing and Communication Plan
1.3.5. Strategies, Forecasting and Campaign Management
1.4. Commerce and Sales
1.4.1. Contributions of Data Analytics in the Commercial Area
1.4.2. Sales Department Needs
1.4.3. Market Research
1.5. Customer Service
1.5.1. Loyalty
1.5.2. Personal Coaching and Emotional Intelligence
1.5.3. Customer Satisfaction
1.6. Purchasing
1.6.1. Data Analytics for Market Research
1.6.2. Data Analytics for Competitive Studies
1.6.3. Other Applications
1.7. Administration
1.7.1. Needs in the Administration Department
1.7.2. Data Warehouse and Financial Risk Analysis
1.7.3. Data Warehouse and President of Credit Risk Analysis
1.8. Human Resources
1.8.1. Human Resources and Benefits of Data Analytics
1.8.2. Data Analytics Tools in the PR Department
1.8.3. Data Analytics Application in the PR Department
1.9. Production
1.9.1. Data Analysis in a Production Department
1.9.2. Applications
1.9.3. Benefits
1.10. IT
1.10.1. IT Department
1.10.2. Data Analytics and Digital Transformation
1.10.3. Innovation and Productivity
Module 2. Data Management, Data Manipulation and Data Science Reporting
2.1. Statistics: Variables, Indexes and Ratios
2.1.1. Statistics
2.1.2. Statistical Dimensions
2.1.3. Variables, Indexes and Ratios
2.2. Data Typology
2.2.1. Qualitative
2.2.2. Quantitative
2.2.3. Characterization and Categories
2.3. Knowledge of Data Places from Measurement
2.3.1. Centralization Measures
2.3.2. Measures of Dispersion
2.3.3. Correlation
2.4. Knowledge of Data Places from Graph
2.4.1. Display According to Data Type
2.4.2. Interpretation of Graphic Information
2.4.3. Customization of Graphics with R
2.5. Probability
2.5.1. Probability
2.5.2. Probability Function
2.5.3. Distribution
2.6. Data Collection
2.6.1. Methodology of Data Collection
2.6.2. Data Collection Tools
2.6.3. Data Collection Channels
2.7. Data Cleaning
2.7.1. Phases of Data Cleansing
2.7.2. Data Quality
2.7.3. Data Manipulation (with R)
2.8. Data Analysis, Interpretation and Evaluation of Results
2.8.1. Statistical Measures
2.8.2. Relationship Indices
2.8.3. Data Mining
2.9. Data Warehouse
2.9.1. Components
2.9.2. Design
2.10. Data Availability
2.10.1. Access
2.10.2. Uses
2.10.3. Security/Safety
Module 3. IoT Devices and Platforms as a Foundation for Data Science
3.1. Internet of Things
3.1.1. Internet of the Future, Internet of Things
3.1.2. The Industrial Internet Consortium
3.2. Architecture of Reference
3.2.1. The Architecture of Reference
3.2.2. Layers
3.2.3. Components
3.3. Sensors and IoT Devices
3.3.1. Main Components
3.3.2. Sensors and Actuators
3.4. Communications and Protocols
3.4.1. Protocols. OSI Model
3.4.2. Communication Technologies
3.5. Cloud Platforms for IoT and IIoT
3.5.1. General Purpose Platforms
3.5.2. Industrial Platforms
3.5.3. Open Code Platforms
3.6. Data Management on IoT Platforms
3.6.1. Data Management Mechanisms. Open Data
3.6.2. Data Exchange and Visualization
3.7. IoT Security
3.7.1. Requirements and Safety Areas
3.7.2. IIoT Security Strategies
3.8. IoT Applications
3.8.1. Intelligent Cities
3.8.2. Health and Fitness
3.8.3. Smart Home
3.8.4. Other Applications
3.9. IIoT Applications
3.9.1. Fabrication
3.9.2. Transport
3.9.3. Energy
3.9.4. Agriculture and Livestock
3.9.5. Other Sectors
3.10. Industry 4.0
3.10.1. IoRT (Internet of Robotics Things)
3.10.2. 3D Additive Manufacturing
3.10.3. Big Data Analytics
Module 4. Graphical Representation for Data Analysis
4.1. Exploratory Analysis
4.1.1. Representation for Information Analysis
4.1.2. The Value of Graphical Representation
4.1.3. New Paradigms of Graphical Representation
4.2. Optimization for Data Science
4.2.1. Color Range and Design
4.2.2. Gestalt in Graphic Representation
4.2.3. Mistakes to Avoid and Tips
4.3. Sources of Basic Data
4.3.1. For Quality Representation
4.3.2. For Amount Representation
4.3.3. For Time Representation
4.4. Sources of Complexity Data
4.4.1. Files, Listings and Data Bases
4.4.2. Open Data
4.4.3. Continuous Generation Data
4.5. Types of Graphs
4.5.1. Basic Representations
4.5.2. Block Representation
4.5.3. Presentation for Dispersion Analysis
4.5.4. Circular Representations
4.5.5. Bubble Representations
4.5.6. Geographical Representations
4.6. Types of Display
4.6.1. Comparative and Relational
4.6.2. Distribution
4.6.3. Hierarchical
4.7. Report Design with Graphical Representation
4.7.1. Application of Graphs in Marketing Reports
4.7.2. Application of Graphs in Scorecards and KPIs
4.7.3. Application of Graphics in Strategic Plans
4.7.4. Other Uses: Science, Health, Business
4.8. Graphic Narration
4.8.1. Graphic Narration
4.8.2. Evolution
4.8.3. Uses
4.9. Visualization-Oriented Tools
4.9.1. Advanced Tools
4.9.2. Online Software
4.9.3. Open Source
4.10. New Technologies in Data Visualization
4.10.1. Systems for Virtualization of Reality
4.10.2. Reality Enhancement and Augmentation Systems
4.10.3. Intelligent Systems
Module 5. Data Science Tools
5.1. Data Science
5.1.1. Data Vizualization
5.1.2. Advanced Tools for the Data Scientist
5.2. Data, Information and Knowledge
5.2.1. Data, Information and Knowledge
5.2.2. Types of Data
5.2.3. Sources of Data
5.3. From Data to Information
5.3.1. Data Analysis
5.3.2. Types of Analysis
5.3.3. Extraction of Information from a Dataset
5.4. Extraction of Information by Visualization
5.4.1. Visualization as an Analysis Tool
5.4.2. Visualization Methods
5.4.3. Visualization of a Data Set
5.5. Quality of Data
5.5.1. Quality Data
5.5.2. Data Cleansing
5.5.3. Data Pre-Basic Processing
5.6. Dataset
5.6.1. Dataset Enrichment
5.6.2. The Curse of Dimensionality
5.6.3. Modification of a Data Set
5.7. Imbalance
5.7.1. Class Imbalance
5.7.2. Imbalance Mitigation Techniques
5.7.3. Balancing a Dataset
5.8. Unsupervised Models
5.8.1. Unsupervised Models
5.8.2. Methods
5.8.3. Classification with Unsupervised Models
5.9. Supervised Models
5.9.1. Supervised Models
5.9.2. Methods
5.9.3. Classification with Unsupervised Models
5.10. Tools and Best Practices
5.10.1. Good Practices for the Data Scientist
5.10.2. The Best Model
5.10.3. Useful Tools
Module 6. Data Mining Selection, Pre-Processing and Transformation
6.1. Statistical Inference
6.1.1. Descriptive Statistics vs. Statistical Inference
6.1.2. Parametric Procedures
6.1.3. Non-Parametric Procedures
6.2. Exploratory Analysis
6.2.1. Descriptive Analysis
6.2.2. Visualization
6.2.3. Data Preparation
6.3. Data Preparation
6.3.1. Data Integration and Data Cleansing
6.3.2. Data Normalization
6.3.3. Transforming Attributes
6.4. Lost Values
6.4.1. Treatment of Missing Values
6.4.2. Maximum Likelihood Imputation Methods
6.4.3. Missing Value Imputation Using Machine Learning
6.5. Noise in the Data
6.5.1. Noise Classes and Attributes
6.5.2. Noise Filtering
6.5.3. The Effect of Noise
6.6. The Curse of Dimensionality
6.6.1. Oversampling
6.6.2. Undersampling
6.6.3. Multidimensional Data Reduction
6.7. From Continuous to Discrete Attributes
6.7.1. Continuous Data vs. Discreet Data
6.7.2. Discretization Process
6.8. The Data
6.8.1. Data Selection
6.8.2. Prospects and Selection Criteria
6.8.3. Selection Methods
6.9. Instance Selection
6.9.1. Methods for Instance Selection
6.9.2. Prototype Selection
6.9.3. Advanced Methods for Instance Selection
6.10. Data Pre-Processing in Big Data Environments
6.10.1. Big Data
6.10.2. Classical vs. Massive Pre-Processing
6.10.3. Smart Data
Module 7. Predictability and Analysis of Stochastic Phenomena
7.1. Time Series
7.1.1. Time Series
7.1.2. Utility and Applicability
7.1.3. Related Case Studies
7.2. The Time Series
7.2.1. ST Seasonality Trend
7.2.2. Typical Variations
7.2.3. Residue Analysis
7.3. Typology
7.3.1. Stationary
7.3.2. Non-Stationary
7.3.3. Transformations and Adjustments
7.4. Schemes for Time Series
7.4.1. Additive Scheme (Model)
7.4.2. Multiplied Scheme (Model)
7.4.3. Procedures for Determining the Type of Model
7.5. Basic Forecasting Methods
7.5.1. Stockings
7.5.2. Naïve
7.5.3. Seasonal Naivety
7.5.4. Comparison of Methods
7.6. Residue Analysis
7.6.1. Autocorrelation
7.6.2. Waste ACF
7.6.3. Correlation Test
7.7. Regression in the Context of Time Series
7.7.1. ANOVA
7.7.2. Fundamentals
7.7.3. Practical Applications
7.8. Predictive Time Series Models
7.8.1. ARIMA
7.8.2. Exponential Smoothing
7.9. Manipulation and Analysis of Time Series with R
7.9.1. Data Preparation
7.9.2. Pattern Identification
7.9.3. Model Analysis
7.9.4. Prediction
7.10. Combined Graphical Analysis with R
7.10.1. Typical Situations
7.10.2. Practical Application for Simple Problem Solving
7.10.3. Practical Application for Advanced Problem Solving
Module 8. Design and Development of Intelligent Systems
8.1. Data Pre-Processing
8.1.1. Data Pre-Processing
8.1.2. Data Transformation
8.1.3. Data Mining
8.2. Automatic Learning
8.2.1. Supervised and Unsupervised Learning
8.2.2. Reinforcement Learning
8.2.3. Other Learning paradigms
8.3. Classification Algorithms
8.3.1. A Utomatic Learning
8.3.2. SVM & KNN
8.3.3. Metrics and Scores for Ranking
8.4. Regression Algorithms
8.4.1. Linear Regression, Logistic Regression and Nonlinear Models
8.4.2. The Time Series
8.4.3. Metrics and Scores for Regression
8.5. Clustering Algorithms
8.5.1. Hierarchical Grouping Techniques
8.5.2. Hierarchical Grouping Techniques
8.5.3. Metrics and Scores for Clustering
8.6. Association Rules Techniques
8.6.1. Methods for Rules Extraction
8.6.2. Metrics and Scores for Association Rule Algorithms
8.7. Advanced Classification Techniques. Multiclassifiers
8.7.1. Bagging Algorithms
8.7.2. Random Forests Sorter
8.7.3. Boosting for Decision Trees
8.8. Probabilistic Graphical Models
8.8.1. Probability Models
8.8.2. Bayesian Networks Properties, Representation and Parameterization
8.8.3. Other Probabilistic Graphical Models
8.9. Neural Networks
8.9.1. Machine Learning with Neural Networks Artificial
8.9.2. Feedforward Networks
8.10. Deep Learning
8.10.1. Deep Feedforward Networks
8.10.2. Convolutional Neural Networks and Sequence Models
8.10.3. Tools for Implementing Deep Neural Networks
Module 9. Tools for Implementing Deep Neural Networks
9.1. Non-Functional Requirements. Pillars of Big Data Applications
9.1.1. Reliability
9.1.2. Adaptability
9.1.3. Maintainability
9.2. Data Models
9.2.1. Relational Model
9.2.2. Documentary Model
9.2.3. Network Data Model
9.3. Databases Data Storage and Retrieval Engines
9.3.1. Hash Indexes
9.3.2. Structured Log Storage
9.3.3. Trees B
9.4. Data Encoding Formats
9.4.1. Language-Specific Formats
9.4.2. Standardized Formats
9.4.3. Binary Encoding Formats
9.4.4. Data Flow between Processes
9.5. Replication
9.5.1. Replication Objectives
9.5.2. Replication Models
9.5.3. Problems with Replication
9.6. Distributed Transactions
9.6.1. Transaction
9.6.2. Protocols for Distributed Transactions
9.6.3. Serializable Transactions
9.7. Partitions
9.7.1. Forms of Partitioning
9.7.2. Secondary Index Interaction and Partitioning
9.7.3. Partition Rebalancing
9.8. Offline Data Processing
9.8.1. Batch Processing
9.8.2. Distributed File Systems
9.8.3. MapReduce
9.9. Real-Time Data Processing
9.9.1. Types of Message Brokers
9.9.2. Representation of Databases as Data Flows
9.9.3. Data Stream Processing
9.10. Practical Applications in the Company
9.10.1. Consistency in Readings
9.10.2. Holistic Approach to Data
9.10.3. Scaling of a Distributed Service
Module 10. Practical Application of Data Science in Sectors of Business Activity
10.1. Health Sector
10.1.1. Implications of AI and Data Analytics in the Healthcare Sector
10.1.2. Opportunities and Challenges
10.2. Risks and Trends in the Healthcare Sector
10.2.1. Use in the Healthcare Sector
10.2.2. Potential Risks Related to the Use of AI
10.3. Financial Services
10.3.1. Implications of AI and Data Analytics in the Financial Services Industry
10.3.2. Use in Financial Services
10.3.3. Potential Risks Related to the Use of AI
10.4. Retail
10.4.1. Implications of AI and Data Analytics in the Retail Sector
10.4.2. Use in Retail
10.4.3. Potential Risks Related to the Use of AI
10.5. Industry 4.0
10.5.1. Implications of AI and Data Analytics in 4.0 Industry
10.5.2. Use in 4.0 Industry
10.6. Risks and Trends in 4.0 Industry
10.6.1. Potential Risks Related to the Use of AI
10.7. Public Administration
10.7.1. Implications of AI and Data Analytics in Public Administration
10.7.2. Use in Public Administration
10.7.3. Potential Risks Related to the Use of AI
10.8. Educational
10.8.1. Implications of AI and Data Analytics in Educational
10.8.2. Potential Risks Related to the Use of AI
10.9. Forestry and Agriculture
10.9.1. Implications of AI and Data Analytics in Forestry and Agriculture
10.9.2. Use in Forestry and Agriculture
10.9.3. Potential Risks Related to the Use of AI
10.10. Human Resources
10.10.1. Implications of AI and Data Analytics in Human Resource Management
10.10.2. Practical Applications in the Business World
10.10.3. Potential Risks Related to the Use of AI
Module 11. Main Information Management Systems
11.1. ERP and CRM
11.1.1. ERP
11.1.2. CRM
11.1.3. Differences between ERP and CRM Selling Point
11.1.4. Business Success
11.2. ERP
11.2.1. ERP
11.2.2. Types of ERP
11.2.3. Development of an ERP Implementation Project
11.2.4. ERP Resource Optimizer
11.2.5. Architecture of an ERP System
11.3. Information Provided by the ERP
11.3.1. Information Provided by the ERP
11.3.2. Advantages and Disadvantages
11.3.3. The Information
11.4. ERP Systems
11.4.1. Current ERP Systems and Tools
11.4.2. Decision-Making
11.4.3. Day-to-Day with ERP
11.5. CRM: The Implementation Project
11.5.1. The CRM The Implementation Project
11.5.2. The CRM as a Commercial Tool
11.5.3. Strategies for the Information System
11.6. CRM: Customer Loyalty
11.6.1. Starting Point
11.6.2. Sell or Loyalty
11.6.3. Factors for Success in our Loyalty System
11.6.4. Multi-Channel Strategies
11.6.5. Design of Loyalty Actions
11.6.6. E-Loyalty
11.7. CRM: Communication Campaigns
11.7.1. Communication Actions and Plans
11.7.2. Importance of the Informed Customer
11.7.3. Listening to the Client
11.8. CRM: Preventing Dissatisfaction
11.8.1. Customer Cancellations
11.8.2. Detecting Errors in Time
11.8.3. Improvement Processes
11.8.4. Recovery of the Dissatisfied Customer
11.9. CRM: Special Communication Actions
11.9.1. Objectives and Planning of a Company Event
11.9.2. Design and Realization of the Event
11.9.3. Actions from the Department
11.9.4. Analysis of Results
11.10. Relational Marketing
11.10.1. Implantation. Errors
11.10.2. Methodology, Segmentation and Processes
11.10.3. Performance, According to the Department
11.10.4. CRM Tools
Module 12. Data Types and Data Life Cycle
12.1. Statistics
12.1.1. Statistics: Descriptive Statistics, Statistical Inferences
12.1.2. Population, Sample, Individual
12.1.3. Variables: Definition, Measurement Scales
12.2. Types of Data Statistics
12.2.1. According to Type
12.2.1.1. Quantitative: Continuous Data and Discrete Data
12.2.1.2. Qualitative: Binomial Data, Nominal Data and Ordinal Data
12.2.2. According to their Shape
12.2.2.1. Numeric
12.2.2.2. Text
12.2.2.3. Logical
12.2.3. According to its Source
12.2.3.1. Primary
12.2.3.2. Secondary
12.3. Life Cycle of Data
12.3.1. Stages of the Cycle
12.3.2. Milestones of the Cycle
12.3.3. FAIR Principles
12.4. Initial Stages of the Cycle
12.4.1. Definition of Goals
12.4.2. Determination of Resource Requirements
12.4.3. Gantt Chart
12.4.4. Data Structure
12.5. Data Collection
12.5.1. Methodology of Data Collection
12.5.2. Data Collection Tools
12.5.3. Data Collection Channels
12.6. Data Cleaning
12.6.1. Phases of Data Cleansing
12.6.2. Data Quality
12.6.3. Data Manipulation (with R)
12.7. Data Analysis, Interpretation and Evaluation of Results
12.7.1. Statistical Measures
12.7.2. Relationship Indices
12.7.3. Data Mining
12.8. Data Warehouse
12.8.1. Elements that Comprise It
12.8.2. Design
12.8.3. Aspects to Consider
12.9. Data Availability
12.9.1. Access
12.9.2. Uses
12.9.3. Security/Safety
12.10. Regulatory Aspects
12.10.1. Data Protection Law
12.10.2. Good Practices
12.10.3. Other Normative Aspects
Module 13. Number - Machine Learning
13.1. Knowledge in Databases
13.1.1. Data Pre-Processing
13.1.2. Analysis
13.1.3. Interpretation and Evaluation of the Results
13.2. Machine Learning
13.2.1. Supervised and Unsupervised Learning
13.2.2. Reinforcement Learning
13.2.3. Semi-Supervised Learning. Other Learning Models
13.3. Classification
13.3.1. Decision Trees and Rule-Based Learning
13.3.2. Support Vector Machines (SVM) and K-Nearest Neighbour (KNN) Algorithms
13.3.3. Metrics for Sorting Algorithms
13.4. Regression
13.4.1. Linear and Logistic Regression
13.4.2. Non-Linear Regression Models
13.4.3. Time Series Analysis
13.4.4. Metrics for Regression Algorithms
13.5. Clustering
13.5.1. Hierarchical Grouping
13.5.2. Partitional Grouping
13.5.3. Metrics for Clustering Algorithms
13.6. Association Rules
13.6.1. Measures of Interest
13.6.2. Rule Extraction Methods
13.6.3. Metrics for Association Rule Algorithms
13.7. Multiclassifiers
13.7.1. Bootstrap Aggregation or Bagging
13.7.2. "Random Forests" Algorithm
13.7.3. “Boosting” Algorithm
13.8. Probabilistic Reasoning Models
13.8.1. Probabilistic Reasoning
13.8.2. Bayesian Networks or Belief Networks
13.8.3. Hidden Markov Models
13.9. Multilayer Perceptron
13.9.1. Neural Network
13.9.2. Machine Learning with Neural Networks
13.9.3. Gradient Descent, Backpropagation and Activation Functions
13.9.4. Implementation of an Artificial Neural Network
13.10. Deep Learning
13.10.1. Deep Neural Networks. Introduction
13.10.2. Convolutional Networks
13.10.3. Sequence Modelling
13.10.4. TensorFlow and Pytorch
Module 14. Web Analytics
14.1. Web Analytics
14.1.1. Introduction
14.1.2. Evolution of Web Analytics
14.1.3. Process of Analysis
14.2. Google Analytics
14.2.1. Google Analytics
14.2.2. Use
14.2.3. Objectives
14.3. Hits. Interactions with the Website
14.3.1. Basic Metrics
14.3.2. KPI (Key Performance Indicators)
14.3.3. Adequate Conversion Rates
14.4. Frequent Dimensions
14.4.1. Source
14.4.2. Medium
14.4.3. Keyword
14.4.4. Campaign
14.4.5. Personalized Labelling
14.5. Setting up Google Analytics
14.5.1. Installation. Creating the Account
14.5.2. Versions of the Tool: UA/GA4
14.5.3. Tracking Label
14.5.4. Conversion Objectives
14.6. Organization of Google Analytics
14.6.1. Account
14.6.2. Property
14.6.3. View
14.7. Google Analytics Reports
14.7.1. In Real Time
14.7.2. Audience
14.7.3. Acquisition
14.7.4. Behaviour
14.7.5. Conversions
14.7.6. e-Commerce
14.8. Google Analytics Advanced Reports
14.8.1. Personalized Reports
14.8.2. Panels
14.8.3. APIs
14.9. Filters and Segments
14.9.1. Filter
14.9.2. Segment
14.9.3. Types of Segments: Predefined / Customized
14.9.4. Remarketing Lists
14.10. Digital Analytics Plan
14.10.1. Measurement
14.10.2. Implementation in the Technological Environment
14.10.3. Conclusions
Module 15. Data Management Regulations
15.1. Regulatory Framework
15.1.1. Normative Framework and Definitions
15.1.2. Controllers, Joint Controllers and Processors
15.1.3. Forthcoming Regulatory Framework for Artificial Intelligence
15.2. Principles Relating to the Processing of Personal Data
15.2.1. Lawfulness, Fairness and Transparency and Purpose Limitation
15.2.2. Data Minimization, Accuracy and Limitation of Retention Period
15.2.3. Integrity and Confidentiality
15.2.4. Proactive Responsibility
15.3. Legitimation and Authorization for Processing
15.3.1. Basis of Legitimacy
15.3.2. Authorizations for the Processing of Special Categories of Data
15.3.3. Data Communications
15.4. Individuals Rights
15.4.1. Transparency and Information
15.4.2. Access
15.4.3. Rectification and Deletion (Right to be Forgotten), Limitation and Portability
15.4.4. Opposition and Automated Individual Decisions
15.4.5. Limits to Rights
15.5. Risk Analysis and Management
15.5.1. Identification of Risks and Threats to the Rights and Freedoms of Individuals
15.5.2. Risk Assessment
15.5.3. Risk Management Plan
15.6. Proactive Accountability Measures
15.6.1. Identifying Techniques to Ensure and Accredit Compliance
15.6.2. Organizational Measures
15.6.3. Technical Measures
15.6.4. Management of Personal Data Security Breaches
15.6.5. The Register of Processing Activities
15.7. The Data Protection Impact Assessment (DPA or DPIA)
15.7.1. Activities Requiring PCIA
15.7.2. Evaluation Methodology
15.7.3. Identification of Risks, Threats and Consultation with the Control Authority
15.8. Contractual Regulation: Persons Responsible, Persons in Charge and Other Subjects
15.8.1. Data Protection Contracts
15.8.2. Attribution of Responsibilities
15.8.3. Contracts between Both Responsible Parties
15.9. International Data Transfers
15.9.1. Definition and Safeguards to Be Adopted
15.9.2. Standard Contractual Clauses
15.9.3. Other Instruments to Regulate Transfers
15.10. Violations and Penalties
15.10.1. Violations and Penalties
15.10.2. Graduation Criteria for Penalties
15.10.3. The Data Protection Officer
15.10.4. Functions of the Supervisory Authorities
Module 16. Scalable and Reliable Mass Data Usage Systems
16.1. Scalability, Reliability and Maintainability
16.1.1. Scales
16.1.2. Reliability
16.1.3. Maintainability
16.2. Data Models
16.2.1. Evolution of Data Models
16.2.2. Comparison of Relational Model with Document-Based NoSQL Model
16.2.3. Network Model
16.3. Data Storage and Retrieval Engines
16.3.1. Structured Log Storage
16.3.2. Storage in Segment Tables
16.3.3. Trees B
16.4. Services, Message Passing and Data Encoding Formats
16.4.1. Data Flow in REST Services
16.4.2. Data Flow in Message Passing
16.4.3. Message Sending Formats
16.5. Replication
16.5.1. CAP Theorem
16.5.2. Consistency Models
16.5.3. Models of Replication Based on Leader and Follower Concepts
16.6. Distributed Transactions
16.6.1. Atomic Operations
16.6.2. Distributed Transactions from Different Approaches Calvin, Spanner
16.6.3. Serializability
16.7. Partitions
16.7.1. Types of Partitions
16.7.2. Indexes in Partitions
16.7.3. Partition Rebalancing
16.8. Batch Processing
16.8.1. Batch Processing
16.8.2. MapReduce
16.8.3. Post-MapReduce Approaches
16.9. Data Stream Processing
16.9.1. Messaging Systems
16.9.2. Persistence of Data Flows
16.9.3. Uses and Operations with Data Flows
16.10. Case Uses. Twitter, Facebook, Uber
16.10.1. Twitter: The Use of Caches
16.10.2. Facebook: Non-Relational Models
16.10.3. Uber: Different Models for Different Purposes
Module 17. System Administration for Distributed Deployments
17.1. Classic Administration. The Monolithic Model
17.1.1. Classical Applications. The Monolithic Model
17.1.2. System Requirements for Monolithic Applications
17.1.3. The Administration of Monolithic Systems
17.1.4. Automization
17.2. Distributed Applications. The Microservice
17.2.1. Distributed Computing Paradigm
17.2.2. Microservices-Based Models
17.2.3. System Requirements for Distributed Models
17.2.4. Monolithic vs. Distributed Applications
17.3. Tools for Resource Exploitation
17.3.1. “Iron” Management
17.3.2. Virtualization
17.3.3. Emulation
17.3.4. Paravirtualization
17.4. IaaS, PaaS and SaaS Models
17.4.1. LaaS Model
17.4.2. PaaS Model
17.4.3. SaaS Model
17.4.4. Design Patterns
17.5. Containerization
17.5.1. Virtualization with Cogroups
17.5.2. Containers
17.5.3. From Application to Container
17.5.4. Container Orchestration
17.6. Clustering
17.6.1. High Performance and High Availability
17.6.2. High Availability Models
17.6.3. Cluster as SaaS Platform
17.6.4. Cluster Securitization
17.7. Cloud Computing
17.7.1. Clusters vs Clouds
17.7.2. Types of Clouds
17.7.3. Clouds Service Models
17.7.4. Oversubscription
17.8. Monitoring and Testing
17.8.1. Types of Monitoring
17.8.2. Visualization
17.8.3. Infrastructure Tests
17.8.4. Chaos Engineering
17.9. Study Case: Kubernetes
17.9.1. Structure
17.9.2. Administration
17.9.3. Deployment of Services
17.9.4. Development of Services for K8S
17.10. Study Case: OpenStack
17.10.1. Structure
17.10.2. Administration
17.10.3. Deployment
17.10.4. Development of Services for OpenStack
Module 18. Project Management and Agile Methodologies
18.1. Project Management
18.1.1. The Project
18.1.2. Phases of a Project
18.1.3. Project Management
18.2. PMI Methodology for Project Management
18.2.1. PMI (Project Management Institute)
18.2.2. PMBOK
18.2.3. Difference between Project, Program and Project Portfolio
18.2.4. Evolution of Organizations Working with Projects
18.2.5. Process Assets in Organizations
18.3. PMI Methodology for Project Management: Processes
18.3.1. Groups of Processes
18.3.2. Knowledge Areas
18.3.3. Process Matrix
18.4. Agile Methodologies for Project Management
18.4.1. VUCA context (Volatility, Uncertainty, Complexity and Ambiguity)
18.4.2. Agile Values
18.4.3. Principles of the Agile Manifesto
18.5. Agile Scrum Framework for Project Management
18.5.1. Scrum
18.5.2. The Pillars of the Scrum Methodology
18.5.3. The Values in Scrum
18.6. Agile Scrum Framework for Project Management. Process
18.6.1. The Scrum Process
18.6.2. Typified Roles in a Scrum Process
18.6.3. The Ceremonies of Scrum
18.7. Agile Scrum Framework for Project Management. Artefacts
18.7.1. Artefacts in the Scrum Process
18.7.2. The Scrum Team
18.7.3. Metrics for Evaluating the Performance of a Scrum Team
18.8. Agile Scrum Framework for Project Management. Kanban Method
18.8.1. Kanban
18.8.2. Benefits of Kanban
18.8.3. Kanban Method Components
18.9. Agile Scrum Framework for Project Management. Kanban Method Practices
18.9.1. The Values of Kanban
18.9.2. Principles of the Kanban Method
18.9.3. General Practices of the Kanban Method
18.9.4. Metrics for Kanban Performance Evaluation
18.10. Comparison: PMI, Scrum and Kanban
18.10.1. PMI- SCRUM
18.10.2. PMI- KANBAN
18.10.3. SCRUM - KANBAN
Module 19. Communication, Leadership and Team Management
19.1. Organizational Development in Business
19.1.1. Climate, Culture and Organizational Development in the Company
19.1.2. Human Capital Management
19.2. Direction Models Decision Making
19.2.1. Paradigm Shift in Management Models
19.2.2. Management Process of the Technology Company
19.2.3. Decision Making Planning Instruments
19.3. Leadership. Delegation and Empowerment
19.3.1. Leadership
19.3.2. Delegation and Empowerment
19.3.3. Performance Evaluation
19.4. Leadership. Knowledge and Talent Management
19.4.1. Talent Management in the Company
19.4.2. Engagement Management in the Company
19.4.3. Improving Communication in the Company
19.5. Coaching Applied to Business
19.5.1. Executive Coaching
19.5.2. Team Coaching
19.6. Mentoring Applied to Business
19.6.1. Mentor Profile
19.6.2. The 4 Processes of a Mentoring Program
19.6.3. Tools and Techniques in a Mentoring Process
19.6.4. Benefits of Mentoring in the Business Environment
19.7. Team Management I. Interpersonal Relations
19.7.1. Interpersonal Relationships
19.7.2. Relational Styles: Approach
19.7.3. Effective Meetings and Agreements in Difficult Situations
19.8. Team Management II. The Conflicts
19.8.1. The Conflicts
19.8.2. Preventing, Addressing and Resolving Conflict
19.8.2.1. Strategies to Prevent Conflict
19.8.2.2. Conflict Management. Basic Principles
19.8.3. Conflict Resolution Strategies
19.8.4. Stress and Work Motivation
19.9. Team Management III. Negotiation
19.9.1. Negotiation at the Managerial Level in Technology Companies
19.9.2. Styles of Negotiation
19.9.3. Negotiation Phases
19.9.3.1. Barriers to Overcome in Negotiations
19.10. Team Management IV. Negotiation Techniques
19.10.1. Negotiation Techniques and Strategies
19.10.1.1. Strategies and Main Types of Negotiation
19.10.1.2. Negotiation Tactics and Practical Issues
19.10.2. The Figure of the Negotiating Subject
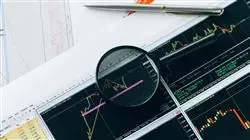
Do not miss the opportunity to mark a before and after in your professional career and enroll now in this MBA in Data Science Management ''
Grand Professional Master's Degree MBA in Data Science Management
.
In a context in which technologies and programs are advancing rapidly to respond adequately to the new digital reality, the emergence of cross-cutting disciplines such as data science is essential. Likewise, the assistance of specialists in the area with a high level of qualification is indispensable as the integration of these technologies becomes an increasing necessity for companies. At TECH Global University we have developed the Advanced Master's Degree in MBA in Data Science Management, a program that will allow you to update your knowledge in order to skillfully approach the management of information with the objective of turning it into a decisive advantage for the companies in which you work. In this way, you will reach a new level of knowledge to progress in your professional goals.
Specialize in the largest Faculty of Computer Science in the world
.
If you are interested in complementing your skills with knowledge in programming and computer science to adapt digital systems and work methodologies to the data storage, processing and analysis needs of modern companies, this program is for you. With the curriculum you will delve into the analytics, management and manipulation of information for data science in the business organization; in the design and development of intelligent systems taking into account the types and life cycle of statistical figures, and in the regulatory framework of data protection and its relationship with the future regulation of systems based on artificial intelligence, among others. This Advanced Master's Degree is a unique opportunity to become an analytical, computer and technological expert capable of assuming great responsibilities in multiple work environments. At the world's largest Faculty of Computer Science, you will be able to take a definitive step towards honing your technical skills and effectively stand out in a highly competitive sector.