University certificate
The world's largest faculty of information technology”
Why study at TECH?
Protecting data is key in the face of constant threats. You could be the guardian of that valuable information”
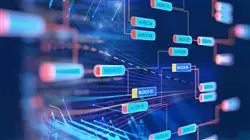
Every second, thousands of pieces of data are generated, shared and stored in the digital environment. From making online payments and accessing educational services to coordinating business activities or protecting digital identities, technology has become an essential pillar that continually transforms the way we live and work. These interactions generate and transfer massive amounts of data at every instant, from personal information to sensitive files related to companies and institutions. This constant flow of data highlights the need for proper handling to ensure its security and privacy.
Managing and protecting this data is no simple task, as it requires the combination of highly specialized expertise in areas such as cybersecurity and information management. These disciplines, although distinct, must be integrated to address the complex challenges of today's digital environment. In this context, the Advanced master’s degree in Secure Information Management represents a unique opportunity for engineers and IT professionals interested in acquiring a comprehensive vision that will enable them to master both areas and position themselves as leaders in a constantly growing sector.
Many companies and institutions face the need to protect critical and highly sensitive data, but lack experts who can ensure effective management, preservation and surveillance of their digital information. To respond to this demand, TECH has designed a program that combines the best content with a teaching team of recognized professional experience. This approach ensures that students acquire the tools and knowledge necessary to stand out in the job market and access strategic positions in organizations seeking to strengthen their information security.
Acquire the skills needed to secure and effectively manage data in a competitive digital environment”
This Advanced master’s degree in Secure Information Management contains the most complete and up-to-date educational program on the market. The most important features include:
- The development of practical cases presented by experts in Secure Information Management
- The graphic, schematic, and practical contents with which they are created, provide scientific and practical information on the disciplines that are essential for professional practice
- Practical exercises where self-assessment can be used to improve learning
- Special emphasis on innovative methodologies in Secure Information Management
- Theoretical lessons, questions to the expert, debate forums on controversial topics, and individual reflection assignments
- Content that is accessible from any fixed or portable device with an Internet connection
Consolidate your theoretical knowledge with the numerous practical resources included in this Advanced master’s degree in Secure Information Management”
The teaching staff includes professionals belonging to the field of Finance, who bring to this program the experience of their work, as well as recognized specialists from leading companies and prestigious universities.
The multimedia content, developed with the latest educational technology, will provide the professional with situated and contextual learning, i.e., a simulated environment that will provide an immersive learning experience designed to prepare for real-life situations.
This program is designed around Problem-Based Learning, whereby the student must try to solve the different professional practice situations that arise throughout the program. For this purpose, the professional will be assisted by an innovative interactive video system created by renowned and experienced experts.
Discover the most innovative educational methodology designed by TECH to guarantee immersive and contextualized learning"
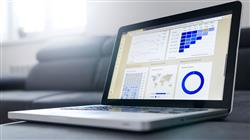
Access a 100% online program that allows you to study at your own pace, at any time and from anywhere in the world"
Syllabus
You will contribute to the protection of sensitive data and the creation of secure systems that guarantee the operational continuity of companies and institutions”
Module 1. Data Analysis in a Business Organization
1.1. Business Analysis
1.1.1. Business Analysis
1.1.2. Data Structure
1.1.3. Phases and Elements
1.2. Data Analysis in the Business
1.2.1. Departmental Scorecards and KPIs
1.2.2. Operational, Tactical and Strategic Reports
1.2.3. Data Analytics Applied to Each Department
1.2.3.1. Marketing and Communication
1.2.3.2. Commercial
1.2.3.3. Customer Service
1.2.3.4. Purchasing
1.2.3.5. Administration
1.2.3.6. HR
1.2.3.7. Production
1.2.3.8. IT
1.3. Marketing and Communication
1.3.1. KPIs for Measurement, Applications and Benefits
1.3.2. Marketing Systems and Data Warehouse
1.3.3. Implementation of a Data Analytics Framework in Marketing
1.3.4. Marketing and Communication Plan
1.3.5. Strategies, Prediction and Campaign Management
1.4. Commerce and Sales
1.4.1. Contributions of Data Analytics in the Commercial Area
1.4.2. Sales Department Needs
1.4.3. Market Research
1.5. Customer Service
1.5.1. Loyalty
1.5.2. Personal Coaching and Emotional Intelligence
1.5.3. Customer Satisfaction
1.6. Purchasing
1.6.1. Data Analysis for Market Research
1.6.2. Data Analysis for Competency Research
1.6.3. Other Applications
1.7. Administration
1.7.1. Needs of the Administration Department
1.7.2. Data Warehouse and Financial Risk Analysis
1.7.3. Data Warehouse and Credit Risk Analysis
1.8. Human Resources
1.8.1. HR and the Benefits of Data Analysis
1.8.2. Data Analytics Tools for the HR Department
1.8.3. Data Analytics Applications for the HR Department
1.9. Production
1.9.1. Data Analysis in a Production Department
1.9.2. Applications
1.9.3. Benefits
1.10. IT
1.10.1. IT Department
1.10.2. Data Analysis and Digital Transformation
1.10.3. Innovation and Productivity
Module 2. Data Management, Data Manipulation and Information Management for Data Science
2.1. Statistics. Variables, Indices and Ratios
2.1.1. Statistics
2.1.2. Statistical Dimensions
2.1.3. Variables, Indices and Ratios
2.2. Type of Data
2.2.1. Qualitative
2.2.2. Quantitative
2.2.3. Characterization and Categories
2.3. Data Knowledge from the Measurements
2.3.1. Centralization Measurements
2.3.2. Measures of Dispersion
2.3.3. Correlation
2.4. Data Knowledge from the Graphs
2.4.1. Visualization According to Type of Data
2.4.2. Interpretation of Graphic Information
2.4.3. Customization of Graphics with
2.5. Probability
2.5.1. Probability
2.5.2. Function of Probability
2.5.3. Distributions
2.6. Data Collection
2.6.1. Methodology of Data Collection
2.6.2. Data Collection Tools
2.6.3. Data Collection Channels
2.7. Data Cleaning
2.7.1. Phases of Data Cleansing
2.7.2. Data Quality
2.7.3. Data Manipulation (with R)
2.8. Data Analysis, Interpretation and Evaluation of Results
2.8.1. Statistical Measures
2.8.2. Relationship Indexes
2.8.3. Data Mining
2.9. Datawarehouse
2.9.1. Components
2.9.2. Design
2.10. Data Availability
2.10.1. Access
2.10.2. Uses
2.10.3. Security
Module 3. Devices and IoT Platforms as a Base for Data Science
3.1. Internet of Things
3.1.1. Internet of the Future, Internet of Things
3.1.2. The Industrial Internet Consortium
3.2. Architecture of Reference
3.2.1. The Architecture of Reference
3.2.2. Layers
3.2.3. Components
3.3. Sensors and IoT Devices
3.3.1. Principal Components
3.3.2. Sensors and Actuators
3.4. Communications and Protocols
3.4.1. Protocols. OSI Model
3.4.2. Communication Technologies
3.5. Cloud Platforms for LoT and LIoT
3.5.1. General Purpose Platforms
3.5.2. Industrial Platforms
3.5.3. Open Code Platforms
3.6. Data Management on IoT Platforms
3.6.1. Data Management Mechanisms. Open Data
3.6.2. Data Exchange and Visualization
3.7. IoT Security
3.7.1. Requirements and Security Areas
3.7.2. Security Strategies in IIoT
3.8. Applications of IoT
3.8.1. Intelligent Cities
3.8.2. Health and Fitness
3.8.3. Smart Home
3.8.4. Other Applications
3.9. Applications of IIoT
3.9.1. Fabrication
3.9.2. Transport
3.9.3. Energy
3.9.4. Agriculture and Livestock
3.9.5. Other Sectors
3.10. Industry 4.0.
3.10.1. IoRT (Internet of Robotics Things)
3.10.2. 3D Additive Manufacturing
3.10.3. Big Data Analytics
Module 4. Graphical Representation of Data Analysis
4.1. Exploratory Analysis
4.1.1. Representation for Information Analysis
4.1.2. The Value of Graphical Representation
4.1.3. New Paradigms of Graphical Representation
4.2. Optimization for Data Science
4.2.1. Color Range and Design
4.2.2. Gestalt in Graphic Representation
4.2.3. Errors to Avoid and Advice
4.3. Basic Data Sources
4.3.1. For Quality Representation
4.3.2. For Quantity Representation
4.3.3. For Time Representation
4.4. Complex Data Sources
4.4.1. Files, Lists and Databases
4.4.2. Open Data
4.4.3. Continuous Data Generation
4.5. Types of Graphs
4.5.1. Basic Representations
4.5.2. Block Representation
4.5.3. Representation for Dispersion Analysis
4.5.4. Circular Representations
4.5.5. Bubble Representations
4.5.6. Geographical Representations
4.6. Types of Visualization
4.6.1. Comparative and Relational
4.6.2. Distribution
4.6.3. Hierarchical
4.7. Report Design with Graphic Representation
4.7.1. Application of Graphs in Marketing Reports
4.7.2. Application of Graphs in Scorecards and KPIs
4.7.3. Application of Graphs in Strategic Plans
4.7.4. Other Uses: Science, Health, Business
4.8. Graphic Narration
4.8.1. Graphic Narration
4.8.2. Evolution
4.8.3. Uses
4.9. Tools Oriented Towards Visualization
4.9.1. Advanced Tools
4.9.2. Online Software
4.9.3. Open Source
4.10. New Technologies in Data Visualization
4.10.1. Systems for Virtualization of Reality
4.10.2. Reality Enhancement and Improvement Systems
4.10.3. Intelligent Systems
Module 5. Data Science Tools
5.1. Data Science
5.1.1. Data Science
5.1.2. Advanced Tools for Data Scientists
5.2. Data, Information and Knowledge
5.2.1. Data, Information and Knowledge
5.2.2. Types of Data
5.2.3. Data Sources
5.3. From Data to Information
5.3.1. Data Analysis
5.3.2. Types of Analysis
5.3.3. Extraction of Information from a Dataset
5.4. Extraction of Information Through Visualization
5.4.1. Visualization as an Analysis Tool
5.4.2. Visualization Methods
5.4.3. Visualization of a Data Set
5.5. Data Quality
5.5.1. Quality Data
5.5.2. Data Cleaning
5.5.3. Basic Data Pre-Processing
5.6. Dataset
5.6.1. Dataset Enrichment
5.6.2. The Curse of Dimensionality
5.6.3. Modification of Our Data Set
5.7. Unbalance
5.7.1. Classes of Unbalance
5.7.2. Unbalance Mitigation Techniques
5.7.3. Balancing a Dataset
5.8. Unsupervised Models
5.8.1. Unsupervised Model
5.8.2. Methods
5.8.3. Classification with Unsupervised Models
5.9. Supervised Models
5.9.1. Supervised Model
5.9.2. Methods
5.9.3. Classification with Supervised Models
5.10. Tools and Good Practices
5.10.1. Good Practices for Data Scientists
5.10.2. The Best Model
5.10.3. Useful Tools
Module 6. Data Mining: Selection, Preprocessing and Transformation
6.1. Statistical Inference
6.1.1. Descriptive Statistics vs. Statistical Inference
6.1.2. Parametric Procedures
6.1.3. Non-Parametric Procedures
6.2. Exploratory Analysis
6.2.1. Descriptive Analysis
6.2.2. Visualization
6.2.3. Data Preparation
6.3. Data Preparation
6.3.1. Integration and Data Cleaning
6.3.2. Normalization of Data
6.3.3. Transforming Attributes
6.4. Missing Values
6.4.1. Treatment of Missing Values
6.4.2. Maximum Likelihood Imputation Methods
6.4.3. Missing Value Imputation Using Machine Learning
6.5. Noise in the Data
6.5.1. Noise Classes and Attributes
6.5.2. Noise Filtering
6.5.3. The Effect of Noise
6.6. The Curse of Dimensionality
6.6.1. Oversampling
6.6.2. Undersampling
6.6.3. Multidimensional Data Reduction
6.7. From Continuous to Discrete Attributes
6.7.1. Continuous Data vs. Discreet Data
6.7.2. Discretization Process
6.8. The Data
6.8.1. Data Selection
6.8.2. Prospects and Selection Criteria
6.8.3. Selection Methods
6.9. Instance Selection
6.9.1. Methods for Instance Selection
6.9.2. Prototype Selection
6.9.3. Advanced Methods for Instance Selection
6.10. Data Pre-Processing in Big Data Environments
6.10.1. Big Data
6.10.2. Classical Versus Massive Pre-processing
6.10.3. Smart Data
Module 7. Predictability and Analysis of Stochastic Phenomena
7.1. Time Series
7.1.1. Time Series
7.1.2. Utility and Applicability
7.1.3. Related Case Studies
7.2. Time Series
7.2.1. Trend Seasonality of TS
7.2.2. Typical Variations
7.2.3. Waste Analysis
7.3. Typology
7.3.1. Stationary
7.3.2. Non-Stationary
7.3.3. Transformations and Settings
7.4. Time Series Schemes
7.4.1. Additive Scheme (Model)
7.4.2. Multiplicative Scheme (Model)
7.4.3. Procedures to Determine the Type of Model
7.5. Basic Forecasting Methods
7.5.1. Media
7.5.2. Naïve
7.5.3. Seasonal Naivety
7.5.4. Method Comparison
7.6. Waste Analysis
7.6.1. Autocorrelation
7.6.2. ACF of Waste
7.6.3. Correlation Test
7.7. Regression in the Context of Time Series
7.7.1. ANOVA
7.7.2. Fundamentals
7.7.3. Practical Applications
7.8. Predictive Methods of Time Series
7.8.1. ARIMA
7.8.2. Exponential Smoothing
7.9. Manipulation and Analysis of Time Series with R
7.9.1. Data Preparation
7.9.2. Identification of Patterns
7.9.3. Model Analysis
7.9.4. Prediction
7.10. Combined Graphical Analysis with R
7.10.1. Normal Situations
7.10.2. Practical Application for the Resolution of Simple Problems
7.10.3. Practical Application for the Resolution of Advanced Problems
Module 8. Design and Development of Intelligent Systems
8.1. Data Pre-Processing
8.1.1. Data Pre-Processing
8.1.2. Data Transformation
8.1.3. Data Mining
8.2. Machine Learning
8.2.1. Supervised and Unsupervised Learning
8.2.2. Reinforcement Learning
8.2.3. Other Learning Paradigms
8.3. Classification Algorithms
8.3.1. Inductive Machine Learning
8.3.2. SVM and KNN
8.3.3. Metrics and Scores for Ranking
8.4. Regression Algorithms
8.4.1. Lineal Regression, Logistical Regression and Non-Lineal Models
8.4.2. Time Series
8.4.3. Metrics and Scores for Regression
8.5. Clustering Algorithms
8.5.1. Hierarchical Clustering Techniques
8.5.2. Partitional Clustering Techniques
8.5.3. Metrics and Scores for Clustering
8.6. Association Rules Techniques
8.6.1. Methods for Rule Extraction
8.6.2. Metrics and Scores for Association Rule Algorithms
8.7. Advanced Classification Techniques. Multiclassifiers
8.7.1. Bagging Algorithms
8.7.2. Random Forests Sorter
8.7.3. Boosting for Decision Trees
8.8. Probabilistic Graphical Models
8.8.1. Probabilistic Models
8.8.2. Bayesian Networks. Properties, Representation and Parameterization
8.8.3. Other Probabilistic Graphical Models
8.9. Neural Networks
8.9.1. Machine Learning with Artificial Neural Networks
8.9.2. Feedforward Networks
8.10. Deep Learning
8.10.1. Deep Feedforward Networks
8.10.2. Convolutional Neural Networks and Sequence Models
8.10.3. Tools for Implementing Deep Neural Networks
Module 9. Architecture and Systems for Intensive Use of Data
9.1. Non-Functional Requirements. Pillars of Big Data Applications
9.1.1. Reliability
9.1.2. Adaptation
9.1.3. Maintainability
9.2. Data Models
9.2.1. Relational Model
9.2.2. Document Model
9.2.3. Graph Type Data Model
9.3. Databases. Storage Management and Data Recovery
9.3.1. H Indexes
9.3.2. Structured Log Storage
9.3.3. B Trees
9.4. Data Coding Formats
9.4.1. Language-Specific Formats
9.4.2. Standardized Formats
9.4.3. Binary Coding Formats
9.4.4. Data Stream Between Processes
9.5. Replication
9.5.1. Objectives of Replication
9.5.2. Replication Models
9.5.3. Problems with Replication
9.6. Distributed Transactions
9.6.1. Transaction
9.6.2. Protocols for Distributed Transactions
9.6.3. Serializable Transactions
9.7. Partitions
9.7.1. Forms of Partitioning
9.7.2. Secondary Index Interaction and Partitioning
9.7.3. Partition Rebalancing
9.8. Offline Data Processing
9.8.1. Batch Processing
9.8.2. Distributed File Systems
9.8.3. MapReduce
9.9. Data Processing in Real Time
9.9.1. Types of Message Brokers
9.9.2. Representation of Databases as Data Streams
9.9.3. Data Stream Processing
9.10. Practical Applications in Business
9.10.1. Consistency in Readings
9.10.2. Holistic Focus of Data
9.10.3. Scaling of a Distributed Service
Module 10. Practical Application of Data Science in Business Sectors
10.1. Health Sector
10.1.1. Implications of AI and Data Analysis in the Health Sector
10.1.2. Opportunities and Challenges
10.2. Risks and Trends in the Health Sector
10.2.1. Use in the Health Sector
10.2.2. Potential Risks Related to the Use of AI
10.3. Financial Services
10.3.1. Implications of AI and Data Analysis in Financial Services Sector
10.3.2. Use in the Financial Services
10.3.3. Potential Risks Related to the Use of AI
10.4. Retail
10.4.1. Implications of AI and Data Analysis in the Retail Sector
10.4.2. Use in Retail
10.4.3. Potential Risks Related to the Use of AI
10.5. Industry 4.0
10.5.1. Implications of AI and Data Analysis in Industry 4.0
10.5.2. Use in Industry 4.0
10.6. Risks and Trends in Industry 4.0
10.6.1. Potential Risks Related to the Use of AI
10.7. Public Administration
10.7.1. Implications of AI and Data Analysis in Public Administration
10.7.2. Use in Public Administration
10.7.3. Potential Risks Related to the Use of AI
10.8. Educational
10.8.1. Implications of AI and Data Analysis in Education
10.8.2. Potential Risks Related to the Use of AI
10.9. Forestry and Agriculture
10.9.1. Implications of AI and Data Analysis in Forestry and Agriculture
10.9.2. Use in Forestry and Agriculture
10.9.3. Potential Risks Related to the Use of AI
10.10. Human Resources
10.10.1. Implications of AI and Data Analysis in Human Resources
10.10.2. Practical Applications in the Business World
10.10.3. Potential Risks Related to the Use of AI
Module 11. Cyberintelligence and Cybersecurity
11.1. Cyberintelligence
11.1.1. Cyberintelligence
11.1.1.1. Intelligence
11.1.1.1.1. Intelligence Cycle
11.1.1.2. Cyberintelligence
11.1.1.3. Cyberintelligence and Cybersecurity
11.1.2. Intelligence Analyst
11.1.2.1. The Role of the Intelligence Analyst
11.1.2.2. The Intelligence Analyst's Biases in Evaluative Activity
11.2. Cybersecurity
11.2.1. Layers of Security
11.2.2. Identification of Cyber Threats
11.2.2.1. External Threats
11.2.2.2. Internal Threats
11.2.3. Adverse Actions
11.2.3.1. Social Engineering
11.2.3.2. Commonly Used Methods
11.3. Techniques and Tools of Intelligences
11.3.1. OSINT
11.3.2. SOCMINT
11.3.3. HUMIT
11.3.4. Linux Distributions and Tools
11.3.5. OWISAM
11.3.6. OWISAP
11.3.7. PTES
11.3.8. OSSTM
11.4. Evaluation Methodologies
11.4.1. Intelligence Analysis
11.4.2. Techniques for Organizing Acquired Information
11.4.3. Reliability and Credibility of Information Sources
11.4.4. Analysis Methodologies
11.4.5. Presentation of Intelligence Results
11.5. Audits and Documentation
11.5.1. IT Security Audit
11.5.2. Documentation and Permits for Auditing
11.5.3. Types of Audits
11.5.4. Deliverables
11.5.4.1. Technical Report
11.5.4.2. Executive Report
11.6. Anonymity in the Network
11.6.1. Use of Anonymity
11.6.2. Anonymity Techniques (Proxy, VPN)
11.6.3. TOR, Freenet and IP2 Networks
11.7. Threats and Types of Security
11.7.1. Types of Threats
11.7.2. Physical Security
11.7.3. Network Security
11.7.4. Logical Security
11.7.5. Web Application Security
11.7.6. Security on Mobile Devices
11.8. Regulations and Compliance
11.8.1. The GDPR
11.8.2. BORRAR
11.8.3. ISO 27000 Family
11.8.4. NIST Cybersecurity Framework
11.8.5. PIC
11.8.6. ISO 27032
11.8.7. Cloud Regulations
11.8.8. SOX
11.8.9. ICP
11.9. Risk Analysis and Metrics
11.9.1. Extent of Risk
11.9.2. The Assets
11.9.3. Threats
11.9.4. Vulnerabilities
11.9.5. Risk Evaluation
11.9.6. Risk Treatment
11.10. Important Cybersecurity Agencies
11.10.1. NIST
11.10.2. ENISA
11.10.3. BORRAR
11.10.4. OEA
11.10.5. UNASUR PROSUR
Module 12. Host Security
12.1. Backup Copies
12.1.1. Backup Strategies
12.1.2. Tools for Windows
12.1.3. Tools for Linux
12.1.4. Tools for MacOS
12.2. User Antivirus
12.2.1. Types of Antivirus
12.2.2. Antivirus for Windows
12.2.3. Antivirus for Linux
12.2.4. Antivirus for MacOS
12.2.5. Antivirus for Smartphones
12.3. Intrusion Detectors - HIDS
12.3.1. Intrusion Detection Methods
12.3.2. Sagan
12.3.3. Aide
12.3.4. Rkhunter
12.4. Local Firewall
12.4.1. Firewalls for Windows
12.4.2. Firewalls for Linux
12.4.3. Firewalls for MacOS
12.5. Password Managers
12.5.1. Password
12.5.2. LastPass
12.5.3. KeePass
12.5.4. StickyPassword
12.5.5. RoboForm
12.6. Detectors for Phishing
12.6.1. Manual Detection of Phishing
12.6.2. Antiphishing Tools
12.7. Spyware
12.7.1. Avoidance Mechanisms
12.7.2. Antispyware Tools
12.8. Trackers
12.8.1. Measures to Protect the System
12.8.2. Anti-Tracking Tools
12.9. EDR- End Point Detection and Response
12.9.1. EDR System Behavior
12.9.2. Differences between EDR and Antivirus
12.9.3. The Future of EDR Systems
12.10. Control Over Software Installation
12.10.1. Repositories and Software Stores
12.10.2. Lists of Permitted or Prohibited Software
12.10.3. Update Criteria
12.10.4. Software Installation Privileges
Module 13. Network Security (Perimeter)
13.1. Threat Detection and Prevention Systems
13.1.1. General Framework for Security Incidents
13.1.2. Current Defense Systems: Defense in Depth and SOC
13.1.3. Current Network Architectures
13.1.4. Types of Tools for Incident Detection and Prevention
13.1.4.1. Network-Based Systems
13.1.4.2. Host-Based Systems
13.1.4.3. Centralized Systems
13.1.5. Instance Hosts, Container and Serverless Communication and Detection
13.2. Firewall
13.2.1. Types of Firewalls
13.2.2. Attacks and Mitigation
13.2.3. Common Firewalls in Linux Kernel
13.2.3.1. UFW
13.2.3.2. Nftables and Iptables
13.2.3.3. Firewalls
13.2.4. Detection Systems Based on System Logs
13.2.4.1. TCP Wrappers
13.2.4.2. BlockHosts and DenyHosts
13.2.4.3. Fai2ban
13.3. Intrusion Detection and Prevention Systems (IDS/IPS)
13.3.1. Attacks on IDS/IPS
13.3.2. IDS/IPS Systems
13.3.2.1. Snort
13.3.2.2. Suricata
13.4. Next Generation Firewalls (NGFW)
13.4.1. Differences between NGFW and Traditional Firewall
13.4.2. Main Capabilities
13.4.3. Commercial Solutions
13.4.4. Firewalls for Cloud Services
13.4.4.1. Virtual Private Cloud (VPC) Architecture
13.4.4.2. Cloud ACLs
13.4.4.3. Security Group
13.5. Proxy
13.5.1. Types of Proxy
13.5.2. Use of Proxy Advantages and Disadvantages
13.6. Antivirus Engines
13.6.1. General Context of Malware and IOCs
13.6.2. Antivirus Engine Problems
13.7. Email Protection Systems
13.7.1. Antispam
13.7.1.1. Black and White Lists
13.7.1.2. Bayesian Filters
13.7.2. Mail Gateway (MGW)
13.8. SIEM
13.8.1. Components and Architecture
13.8.2. Correlation Rules and Use Cases
13.8.3. Current Challenges of SIEM Systems
13.9. SOAR
13.9.1. SOAR and SIEM: Enemies or Allies
13.9.2. The Future of SOAR Systems
13.10. Other Network-Based Systems
13.10.1. WAF
13.10.2. NAC
13.10.3. HoneyPots and HoneyNets
13.10.4. CASB
Module 14. Smartphone Security
14.1. The World of Mobile Devices
14.1.1. Types of Mobile Platforms
14.1.2. IOS Devices
14.1.3. Android Devices
14.2. Mobile Security Management
14.2.1. OWASP Mobile Security Project
14.2.1.1. Top 10 Vulnerabilities
14.2.2. Communications, Networks and Connection Modes
14.3. Mobile Devices in Business Environments
14.3.1. Risk
14.3.2. Security Policies
14.3.3. Device Monitoring
14.3.4. Mobile Device Management (MDM)
14.4. User Privacy and Data Security
14.4.1. Statements of Information
14.4.2. Data Protection and Confidentiality
14.4.2.1. Licenses
14.4.2.2. Encryption
14.4.3. Secure Data Storage
14.4.3.1. Secure Storage on iOS
14.4.3.2. Secure Storage on Android
14.4.4. Best Practices in Application Development
14.5. Vulnerabilities and Attack Vectors
14.5.1. Vulnerabilities
14.5.2. Attack Vectors
14.5.2.1. Malware
14.5.2.2. Data Exfiltration
14.5.2.3. Data Manipulation
14.6. Main Threats
14.6.1. Unforced User
14.6.2. Malware
14.6.2.1. Types of Malware
14.6.3. Social Engineering
14.6.4. Data Leakage
14.6.5. Information Theft
14.6.6. Unsecured Wi-Fi Networks
14.6.7. Outdated Software
14.6.8. Malicious Applications
14.6.9. Insecure Passwords
14.6.10 Weak or No Security Configuration
14.6.11. Physical Access
14.6.12. Loss or Theft of the Device
14.6.13. Identity Theft (Integrity)
14.6.14. Weak or Broken Cryptography
14.6.15. Denial of Service (DoS)
14.7. Main Attacks
14.7.1. Phishing Attacks
14.7.2. Attacks Related to Communication Modes
14.7.3. Smishing Attacks
14.7.4. Cryptojacking Attacks
14.7.5. Man in The Middle
14.8. Hacking
14.8.1. Rooting and Jailbreaking
14.8.2. Anatomy of a Mobile Attack
14.8.2.1. Threat Propagation
14.8.2.2. Malware Installation on the Device
14.8.2.3. Persistence
14.8.2.4. Payload Execution and Information Extraction
14.8.3. Hacking on iOS Devices: Mechanisms and Tools
14.8.4. Hacking Android Devices: Mechanisms and Tools
14.9. Penetration Testing
14.9.1. iOS PenTesting
14.9.2. Android PenTesting
14.9.3. Tools
14.10. Safety and Security
14.10.1. Security Configuration
14.10.1.1. On iOS Devices
14.10.1.2. On Android Devices
14.10.2. Safety Measures
14.10.3. Protection Tools
Module 15. IoT Security
15.1. Devices
15.1.1. Types of Devices
15.1.2. Standardized Architectures
15.1.2.1. ONEM2M
15.1.2.2. IoTWF
15.1.3. Application Protocols
15.1.4. Connectivity Technologies
15.2. IoT Devices. Areas of Application
15.2.1. SmartHome
15.2.2. SmartCity
15.2.3. Transportation
15.2.4. Wearables
15.2.5. Health Sector
15.2.6. IioT
15.3. Communication Protocols
15.3.1. MQTT
15.3.2. LWM2M
15.3.3. OMA-DM
15.3.4. TR-069
15.4. SmartHome
15.4.1. Home Automation
15.4.2. Networks
15.4.3. Household Appliances
15.4.4. Surveillance and Security
15.5. SmartCity
15.5.1. Lighting
15.5.2. Meteorology
15.5.3. Security
15.6. Transportation
15.6.1. Localization
15.6.2. Making Payments and Obtaining Services
15.6.3. Connectivity
15.7. Wearables
15.7.1. Smart Clothing
15.7.2. Smart Jewelry
15.7.3. Smart Watches
15.8. Health Sector
15.8.1. Exercise/Heart Rate Monitoring
15.8.2. Monitoring of Patients and Elderly People
15.8.3. Implantable
15.8.4. Surgical Robots
15.9. Connectivity
15.9.1. Wi-Fi/Gateway
15.9.2. Bluetooth
15.9.3. Built-In Connectivity
15.10. Securitization
15.10.1. Dedicated Networks
15.10.2. Password Managers
15.10.3. Use of Encrypted Protocols
15.10.4. Tips for Use
Module 16. Ethical Hacking
16.1. Work Environment
16.1.1. Linux Distributions
16.1.1.1. Kali Linux - Offensive Security
16.1.1.2. Parrot OS
16.1.1.3. Ubuntu
16.1.2. Virtualization Systems
16.1.3. Sandbox
16.1.4. Deployment of Laboratories
16.2. Methods
16.2.1. OSSTM
16.2.2. OWASP
16.2.3. NIST
16.2.4. PTES
16.2.5. ISSAF
16.3. Footprinting
16.3.1. Open-Source Intelligence (OSINT)
16.3.2. Search for Data Breaches and Vulnerabilities
16.3.3. Use of Passive Tools
16.4. Network Scanning
16.4.1. Scanning Tools
16.4.1.1. Nmap
16.4.1.2. Hping3
16.4.1.3. Other Scanning Tools
16.4.2. Scanning Techniques
16.4.3. Firewall and IDS Avoidance Techniques
16.4.4. Banner Grabbing
16.4.5. Network Diagrams
16.5. Enumeration
16.5.1. SMTP Enumeration
16.5.2. DNS Enumeration
16.5.3. NetBIOS and Samba Enumeration
16.5.4. LDAP Enumeration
16.5.5. SNMP Enumeration
16.5.6. Other Enumeration Techniques
16.6. Vulnerability Analysis
16.6.1. Vulnerability Scanning Solutions
16.6.1.1. Qualys
16.6.1.2. Nessus
16.6.1.3. CFI LanGuard
16.6.2. Vulnerability Scoring Systems
16.6.2.1. CVSS
16.6.2.2. CVE
16.6.2.3. NVD
16.7. Attacks on Wireless Networks
16.7.1. Methodology of Hacking in Wireless Networks
16.7.1.1. Wi-Fi Discovery
16.7.1.2. Traffic Analysis
16.7.1.3. Aircrack Attacks
16.7.1.3.1. WEP Attacks
16.7.1.3.2. WPA/WPA2 Attacks
16.7.1.4. Evil Twin Attacks
16.7.1.5. Attacks on WPS
16.7.1.6. Jamming
16.7.2. Tools for Wireless Security
16.8. Hacking of Web Servers
16.8.1. Cross Site Scripting
16.8.2. CSRF
16.8.3. Session Hijacking
16.8.4. SQLinjection
16.9. Exploiting Vulnerabilities
16.9.1. Use of Known Exploits
16.9.2. Use of Metasploit
16.9.3. Use of Malware
16.9.3.1. Definition and Scope
16.9.3.2. Malware Generation
16.9.3.3. Bypass of Antivirus Solutions
16.10. Persistence
16.10.1. Rootkits Installation
16.10.2. Use of Ncat
16.10.3. Use of Programmed Tasks for Backdoors
16.10.4. User Creation
16.10.5. HIDS Detection
Module 17. Reverse Engineering
17.1. Compilers
17.1.1. Types of Codes
17.1.2. Phases of a Compiler
17.1.3. Table of Symbols
17.1.4. Error Manager
17.1.5. GCC Compiler
17.2. Types of Analysis in Compilers
17.2.1. Lexical Analysis
17.2.1.1. Terminology
17.2.1.2. Lexical Components
17.2.1.3. LEX Lexical Analyzer
17.2.2. Parsing
17.2.2.1. Context-Free Grammars
17.2.2.2. Types of Parsing
17.2.2.2.1. Top-Down Analysis
17.2.2.2.2. Bottom-Up Analysis
17.2.2.3. Syntactic Trees and Derivations
17.2.2.4. Types of Parsers
17.2.2.4.1. LR (Left To Right) Analyzers
17.2.2.4.2. LALR Analyzers
17.2.3. Semantic Analysis
17.2.3.1. Attribute Grammars
17.2.3.2. S-Attributed
17.2.3.3. L-Attributed
17.3. Data Structures in Assembler
17.3.1. Variables
17.3.2. Arrays
17.3.3. Pointers
17.3.4. Structures
17.3.5. Objects
17.4. Assembler Code Structures
17.4.1. Selection Structures
17.4.1.1. If, Else If, Else
17.4.1.2. Switch
17.4.2. Iteration Structures
17.4.2.1. For
17.4.2.2. While
17.4.2.3. Use of Break
17.4.3. Functions
17.5. X86 Architecture Hardware
17.5.1. x86 Processor Architecture
17.5.2. x86 Data Structures
17.5.3. x86 Code Structures
17.6. ARM Architecture Hardware
17.6.1. ARM Processor Architecture
17.6.2. ARM Data Structures
17.6.3. ARM Code Structures
17.7. Static Code Analysis
17.7.1. Disassemblers
17.7.2. IDA
17.7.3. Code Rebuilders
17.8. Dynamic Code Analysis
17.8.1. Behavioral Analysis
17.8.1.1. Communications
17.8.1.2. Monitoring
17.8.2. Linux Code Debuggers
17.8.3. Windows Code Debuggers
17.9. Sandbox
17.9.1. Sandbox Architecture
17.9.2. Sandbox Evasion
17.9.3. Detection Techniques
17.9.4. Avoidance Techniques
17.9.5. Countermeasures
17.9.6. Sandbox in Linux
17.9.7. Sandbox in Windows
17.9.8. Sandbox in MacOS
17.9.9. Sandbox in Android
17.10. Malware Analysis
17.10.1. Malware Analysis Methods
17.10.2. Malware Obfuscation Techniques
17.10.2.1. Executable Obfuscation
17.10.2.2. Restriction of Execution Environments
17.10.3. Malware Analysis Tools
Module 18. Secure Development
18.1. Secure Development
18.1.1. Quality, Functionality and Safety
18.1.2. Confidentiality, Integrity and Availability
18.1.3. Software Development Life Cycle
18.2. Requirements Phase
18.2.1. Authentication Control
18.2.2. Role and Privilege Control
18.2.3. Risk-Oriented Requirements
18.2.4. Privilege Approval
18.3. Analysis and Design Phases
18.3.1. Component Access and System Administration
18.3.2. Audit Trails
18.3.3. Session Management
18.3.4. Historical Data
18.3.5. Proper Error Handling
18.3.6. Separation of Functions
18.4. Implementation and Coding Phase
18.4.1. Ensuring the Development Environment
18.4.2. Preparation of Technical Documentation
18.4.3. Secure Codification
18.4.4. Communications Security
18.5. Good Secure Coding Practices
18.5.1. Input Data Validation
18.5.2. Coding of Output Data
18.5.3. Programming Style
18.5.4. Change Log Management
18.5.5. Cryptographic Practices
18.5.6. Error and Log Management
18.5.7. File Management
18.5.8. Memory Management
18.5.9. Standardization and Reuse of Security Functions
18.6. Server Preparation and Hardening
18.6.1. Management of Users, Groups and Roles on the Server
18.6.2. Software Installation
18.6.3. Server Hardening
18.6.4. Robust Configuration of the Application Environment
18.7. Preparing Databases and Hardening
18.7.1. DB Engine Optimization
18.7.2. Create Your Own User for the Application
18.7.3. Assigning the Required Privileges to the User
18.7.4. Hardening of the Databases
18.8. Testing Phase
18.8.1. Quality Control in Security Controls
18.8.2. Phased Code Inspection
18.8.3. Checking Configuration Management
18.8.4. Black Box Testing
18.9. Preparing the Transition to Production
18.9.1. Perform Change Control
18.9.2. Carry out Production Changeover Procedure
18.9.3. Perform Rollback Procedure
18.9.4. Pre-Production Testing
18.10. Maintenance Phase
18.10.1. Risk-Based Assurance
18.10.2. White Box Security Maintenance Testing
18.10.3. Black Box Safety Maintenance Tests
Module 19. Forensic Analysis
19.1. Data Acquisition and Duplication
19.1.1. Volatile Data Acquisition
19.1.1.1. System Information
19.1.1.2. Network Information
19.1.1.3. Volatility Order
19.1.2. Static Data Acquisition
19.1.2.1. Creating a Duplicate Image
19.1.2.2. Preparation of a Chain of Custody Document
19.1.3. Methods for Validation of Acquired Data
19.1.3.1. Methods for Linux
19.1.3.2. Methods for Windows
19.2. Evaluation and Defeat of Antiforensic Techniques
19.2.1. Objectives of Antiforensic Techniques
19.2.2. Data Deletion
19.2.2.1. Deletion of Data and Files
19.2.2.2. File Recovery
19.2.2.3. Recovery of Deleted Partitions
19.2.3. Password Protection
19.2.4. Steganography
19.2.5. Secure Device Wiping
19.2.6. Encryption
19.3. Forensic Analysis of the Operating System
19.3.1. Windows Forensics
19.3.2. Linux Forensics
19.3.3. Mac Forensics
19.4. Network Forensic Analysis
19.4.1. Logs Analysis
19.4.2. Data Correlation
19.4.3. Network Research
19.4.4. Steps to Follow in Network Forensic Analysis
19.5. Web Forensics
19.5.1. Investigation of Web Attacks
19.5.2. Attack Detection
19.5.3. IP Address Location
19.6. Forensic Database Analysis
19.6.1. Forensic Analysis in MSSQL
19.6.2. MySQL Forensic Analysis
19.6.3. PostgreSQL Forensic Analysis
19.6.4. Forensic Analysis in MongoDB
19.7. Cloud Forensics
19.7.1. Types of Crimes in the Cloud
19.7.1.1. Cloud as a Subject
19.7.1.2. Cloud as an Object
19.7.1.3. Cloud as a Tool
19.7.2. Challenges of Cloud Forensics
19.7.3. Research on Cloud Storage Services
19.7.4. Cloud Forensic Analysis Tools
19.8. Investigation of Email Crimes
19.8.1. Mailing Systems
19.8.1.1. Mail Clients
19.8.1.2. Mail Server
19.8.1.3. SMTP Server
19.8.1.4. POP3 Server
19.8.1.5. IMAP4 Server
19.8.2. Mailing Crimes
19.8.3. Mail Message
19.8.3.1. Standard Headers
19.8.3.2. Extended Headers
19.8.4. Steps for the Investigation of These Crimes
19.8.5. E-Mail Forensic Tools
19.9. Mobile Forensic Analysis
19.9.1. Cellular Networks
19.9.1.1. Types of Networks
19.9.1.2. CDR Contents
19.9.2. Subscriber Identity Module (SIM)
19.9.3. Logical Acquisition
19.9.4. Physical Acquisition
19.9.5. File System Acquisition
19.10. Forensic Report Writing and Presentation
19.10.1. Important Features of a Forensic Report
19.10.2. Classification and Types of Reports
19.10.3. Guide to Writing a Report
19.10.4. Presentation of the Report
19.10.4.1. Prior Preparation for Testifying
19.10.4.2. Deposition
19.10.4.3. Dealing with the Media
Module 20. Current and Future Challenges in Information Security
20.1. Blockchain Technology
20.1.1. Scope of Application
20.1.2. Confidentiality Guarantee
20.1.3. Non-Repudiation Guarantee
20.2. Digital Money
20.2.1. Bitcoins
20.2.2. Cryptocurrencies
20.2.3. Cryptocurrency Mining
20.2.4. Pyramid Schemes
20.2.5. Other Potential Crimes and Problems
20.3. Deepfake
20.3.1. Media Impact
20.3.2. Dangers to Society
20.3.3. Detection Mechanisms
20.4. The Future of Artificial Intelligence
20.4.1. Artificial Intelligence and Cognitive Computing
20.4.2. Uses to Simplify Customer Service
20.5. Digital Privacy
20.5.1. Value of Data in the Network
20.5.2. Use of Data in the Network
20.5.3. Privacy and Digital Identity Management
20.6. Cyberconflicts, Cybercriminals and Cyberattacks
20.6.1. The Impact of Cybersecurity on International Conflicts
20.6.2. Consequences of Cyber-attacks on the General Population.
20.6.3. Types of Cybercriminals. Protective Measures
20.7. Telework
20.7.1. Remote Work Revolution during and post COVID-19
20.7.2. Access Bottlenecks
20.7.3. Variation of the Attacking Surface
20.7.4. Workers' Needs
20.8. Emerging Wireless Technologies
20.8.1. WPA3
20.8.2. 5G
20.8.3. Millimeter Waves
20.8.4. Trend in Get Smart instead of Get More
20.9. Future Addressing in Networks
20.9.1. Current Problems with IP Addressing
20.9.2. IPv6
20.9.3. IPv4+
20.9.4. Advantages of IPv4+ Over IPv4
20.9.5. Advantages of IPv6 Over IPv4
20.10. The Challenge of Raising Awareness of Early and Continuing Education in the Population
20.10.1. Current Government Strategies
20.10.2. Resistance of the Population to Learning
20.10.3. Training Plans to be Adopted by Companies
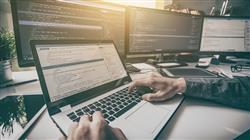
You will learn through real-world cases designed in simulated learning environments that reflect current challenges in data management and cybersecurity”
Advanced Master’s Degree in Secure Information Management
Digitalization processes are becoming increasingly common in the different areas in which we develop. However, although these technologies offer multiple benefits for carrying out a large number of work and leisure activities, they can also represent a risk factor for the integrity of users. Faced with a complex scenario in which we are increasingly exposed due to the massive amounts of data that are transferred daily, from conversations through social networks, to sensitive documents hosted on banking or business websites, it is necessary to have professionals who have specialized knowledge in data protection in digital media. For this reason, at TECH Global University we have designed the Advanced Master's Degree in Secure Information Management, a program that will prepare you to collect, process and analyze all types of information, having as a principle of all professional performance, security in data management.
Specialize in secure information management
If you want to stand out as one of the best specialists in a highly competitive sector, this program is perfect for you. The curriculum, presented in a 100% online format, brings together in a comprehensive way the updating, deepening and systematization of the most important aspects on data management and protection, which will allow you to compile the current regulations on cybersecurity, develop appropriate usage policies, evaluate threat detection systems, and identify and develop strategies for risk prevention and resolution. At the largest School of Computer Science you will strengthen your skills and boost your career growth as a cyber intelligence and cybersecurity expert.