University certificate
The world's largest faculty of information technology”
Why study at TECH?
You are before a Hybrid professional master’s degree that will lead you to progress professionally in the area of Data Science and become part of the big companies in the sector"
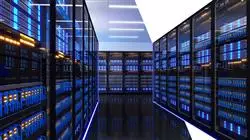
Today, in a globalized world immersed in online commerce, information and data flow at high speed and in large quantities. That is why the collection of data and its application in business strategy has been an important boost to data science. This is why companies are betting on its use to be able to draw better business strategies, expand their knowledge about the consumer, detect the risk of losses or establish more effective marketing campaigns.
In this way, Data Science comprises a combination of mathematical methods, computer science, analysis skills and optimal communication of the results obtained. A specialty, therefore, that requires qualified professionals with team management skills. In this scenario, TECH offers this Hybrid professional master’s degree, which provides students with a quality education, with a theoretical framework 100% online, complemented by an internship in a leading company in this area.
A program that will lead over 12 months to delve into the main information management systems, the life cycle of data, machine learning and web analytics. All this, through multimedia teaching resources that can be accessed, comfortably whenever you want, from any electronic device with internet connection.
Likewise, the graduate will be able to apply all the concepts acquired during the 3 weeks of the internship. A period where students will be tutored by professionals of excellent level and experience in Data Science. This scenario will not only provide the student with first-hand knowledge of the current techniques and methodologies used in this area, but will also lead to a more complete learning process in an area of constant innovation.
This institution therefore offers an excellent opportunity for students who wish to advance professionally in a booming sector. All this, through a theoretical-practical approach, which brings you closer to the most current reality of Data Science, through a unique program in the current educational landscape.
With this program you will gain an advanced knowledge of web analytics and contribute to the successful development of online businesses"
This Hybrid professional master’s degree in Corporate Technical Data Science Management contains the most complete and up-to-date program on the market. The most important features include:
- Development of more than 100 cases presented by Data Science professionals in companies
- The graphic, schematic, and practical contents with which they are created, provide scientific and practical information on the disciplines that are essential for professional practice
- Development of the activities and phases of the data protection impact assessment process
- With a special emphasis on data management regulations
- Knowledge of the most useful tools for the implementation of IaaS and PaaS models
- All of this will be complemented by theoretical lessons, questions to the expert, debate forums on controversial topics, and individual reflection assignments
- Content that is accessible from any fixed or portable device with an Internet connection
- In addition, you will be able to do an internship in one of the best Data Science centers in the world
Take an intensive 3-week internship in a prestigious center and acquire the skills you need to progress professionally with an excellent team of experts"
In this proposal for a Hybrid professional master’s degree, of a professionalizing nature and blended learning modality, the program is aimed at updating physical activity professionals who develop their functions in sports centers, and who require a high level of qualification. The contents are based on the latest scientific evidence, and oriented in a didactic way to integrate theoretical knowledge in the practice of Data Science technical management, and the theoretical-practical elements will facilitate the acquisition of knowledge and will allow the appropriate decision making in the technological field.
Thanks to multimedia content developed with the latest educational technology, professionals will enjoy a situated and contextual learning, i.e., a simulated environment that will provide immersive learning programmed to prepare professionals for real situations. This program is designed around Problem-Based Learning, whereby the physician must try to solve the different professional practice situations that arise during the course. For this purpose, the students will be assisted by an innovative interactive video system created by renowned and experienced experts.
Thanks to this program, you will master the main tools for the implementation of IaaS and PaaS models in business"
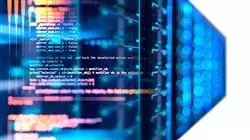
With this program you will be able to establish the communication protocols and technologies used in the Internet of Things"
Teaching Planning
The syllabus of this Hybrid professional master’s degree has been developed by a multidisciplinary teaching team, which has poured into this syllabus its extensive knowledge of Data Science. Therefore, students will be able to delve into the information management systems, the types of data and the main computer programs for their development and analysis. For this purpose, students will be provided with high quality multimedia teaching resources, available 24 hours a day. To complete this academic journey, students will have an internship in a prestigious technology company, where they will be able to deploy their technical and analytical skills in Data Science.
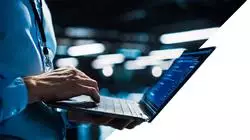
Video summaries, videos in detail, case studies... access them, whenever you want, from an electronic device with an internet connection"
Module 1. The Main Information Management Systems
1.1. ERP and CRM
1.1.1. ERP
1.1.2. CRM
1.1.3. Differences between ERP and CRM Selling Point
1.1.4. Business Success
1.2. ERP
1.2.1. ERP
1.2.2. Types of ERP
1.2.3. Development of an ERP Implementation Project
1.2.4. ERP Resource Optimiser
1.2.5. Architecture of an ERP System
1.3. Information Provided by the ERP
1.3.1. Information Provided by the ERP
1.3.2. Advantages and Disadvantages
1.3.3. The Information
1.4. ERP Systems
1.4.1. Current ERP Systems and Tools
1.4.2. Decision Making
1.4.3. Day-to-Day with ERP
1.5. CRM: The Implementation Project
1.5.1. The CRM The Implementation Project
1.5.2. The CRM as a Commercial Tool
1.5.3. Strategies for the Information System
1.6. CRM: Customer Loyalty
1.6.1. Starting Point
1.6.2. Sales or Loyalty
1.6.3. Factors for Success in our Loyalty System
1.6.4. Multi-Channel Strategies
1.6.5. Design of Loyalty Actions
1.6.6. E-Loyalty
1.7. CRM: Communication Campaigns
1.7.1. Communication Actions and Plans
1.7.2. Importance of the Informed Customer
1.7.3. Listening to the Client
1.8. CRM: Dissatisfaction Prevention
1.8.1. Customer Cancellations
1.8.2. Detecting Errors in Time
1.8.3. Improvement Processes
1.8.4. Recovery of the Dissatisfied Customer
1.9. CRM: Special Communication Actions
1.9.1. Objectives and Planning of a Company Event
1.9.2. Design and Realisation of the Event
1.9.3. Actions from the Department
1.9.4. Analysis of Results
1.10. Relational Marketing
1.10.1. Implantation. Errors
1.10.2. Methodology, Segmentation and Processes
1.10.3. Performance, According to the Department
1.10.4. CRM Tools
Module 2. Data Types and Data Life Cycle
2.1. Statistics
2.1.1. Statistics: Descriptive Statistics, Statistical Inferences
2.1.2. Population, Sample, Individual
2.1.3. Variables: Definition, Measurement Scales
2.2. Types of Data Statistics
2.2.1. According to Type
2.2.1.1. Quantitative: Continuous Data and Discrete Data
2.2.1.2. Qualitative: Binomial Data, Nominal Data and Ordinal Data
2.2.2. According to their Shape
2.2.2.1. Numeric
2.2.2.2. Text:
2.2.2.3. Logical
2.2.3. According to its Source
2.2.3.1. Primary
2.2.3.2. Secondary
2.3. Life Cycle of Data
2.3.1. Stages of the Cycle
2.3.2. Milestones of the Cycle
2.3.3. FAIR Principles
2.4. Initial Stages of the Cycle
2.4.1. Definition of Goals
2.4.2. Determination of Resource Requirements
2.4.3. Gantt Chart
2.4.4. Data Structure
2.5. Data Collection
2.5.1. Methodology of Data Collection
2.5.2. Data Collection Tools
2.5.3. Data Collection Channels
2.6. Data Cleaning
2.6.1. Phases of Data Cleansing
2.6.2. Data Quality
2.6.3. Data Manipulation (with R)
2.7. Data Analysis, Interpretation and Evaluation of Results
2.7.1. Statistical Measures
2.7.2. Relationship Indices
2.7.3. Data Mining
2.8. Data Warehouse (Datawarehouse)
2.8.1. Elements that Comprise it
2.8.2. Design
2.8.3. Aspects to Consider
2.9. Data Availability
2.9.1. Access
2.9.2. Uses
2.9.3. Security/Safety
2.10. Regulatory Aspects
2.10.1. Data Protection Law
2.10.2. Good Practices
2.10.3. Other Normative Aspects
Module 3. Number Machine Learning
3.1. Knowledge in Databases
3.1.1. Data Pre-Processing
3.1.2. Analysis
3.1.3. Interpretation and Evaluation of the Results
3.2. Machine Learning
3.2.1. Supervised and Unsupervised Learning
3.2.2. Reinforcement Learning
3.2.3. Semi-Supervised Learning: Other Learning Models
3.3. Classification
3.3.1. Decision Trees and Rule-Based Learning
3.3.2. Support Vector Machines (SVM) and K-Nearest Neighbour (KNN) Algorithms
3.3.3. Metrics for Sorting Algorithms
3.4. Regression
3.4.1. Linear and Logistic Regression
3.4.2. Non-Linear Regression Models
3.4.3. Time Series Analysis
3.4.4. Metrics for Regression Algorithms
3.5. Clustering
3.5.1. Hierarchical Grouping
3.5.2. Partitional Grouping
3.5.3. Metrics for Clustering Algorithms
3.6. Association Rules
3.6.1. Measures of Interest
3.6.2. Rule Extraction Methods
3.6.3. Metrics for Association Rule Algorithms
3.7. Multiclassifiers
3.7.1. “Bootstrap Aggregation" or "Bagging"
3.7.2. "Random Forests" Algorithm
3.7.3. “Boosting” Algorithm
3.8. Probabilistic Reasoning Models
3.8.1. Probabilistic reasoning
3.8.2. Bayesian Networks or Belief Networks
3.8.3. “Hidden Markov Models”
3.9. Multilayer Perceptron
3.9.1. Neural Network:
3.9.2. Machine Learning with Neural Networks
3.9.3. Gradient Descent, Backpropagation and Activation Functions
3.9.4. Implementation of an Artificial Neural Network
3.10 Deep Learning
3.10.1. Deep Neural Networks. Introduction
3.10.2. Convolutional Networks
3.10.3. Sequence Modelling
3.10.4. Tensorflow and Pytorch
Module 4. Web Analytics
4.1. Web Analytics
4.1.1. Introduction
4.1.2. Evolution of Web Analytics
4.1.3. Analysis Process
4.2. Google Analytics
4.2.1. Google Analytics
4.2.2. Use
4.2.3. Objetivos
4.3. Hits. Interactions with the Website
4.3.1. Basic Metrics
4.3.2. KPI (Key Performance Indicators)
4.3.3. Adequate Conversion Rates
4.4. Frequent Dimensions
4.4.1. Source
4.4.2. Medium
4.4.3. Keyword
4.4.4. Campaign
4.4.5. Personalized Labelling
4.5. Setting up Google Analytics
4.5.1. Installation. Creating the Account
4.5.2. Versions of the Tool: UA/GA4
4.5.3. Tracking Label
4.5.4. Conversion Objectives
4.6. Organization of Google Analytics
4.6.1. Account
4.6.2. Property
4.6.3. View
4.7. Google Analytics Reports
4.7.1. In Real Time
4.7.2. Audience
4.7.3. Acquisition
4.7.4. Behaviour
4.7.5. Conversions
4.7.6. E-Commerce
4.8. Google Analytics Advanced Reports
4.8.1. Personalised Reports
4.8.2. Panels
4.8.3. APIs
4.9. Filters and Segments
4.9.1. Filter
4.9.2. Segment
4.9.3. Types of Segments: Predefined/Customized
4.9.4. Remarketing Lists
4.10. Digital Analytics Plan
4.10.1. Measurement
4.10.2. Implementation in the Technological Environment
4.10.3. Conclusions
Module 5. Data Management Regulations
5.1. Regulatory Framework
5.1.1. Normative Framework and Definitions
5.1.2. Controllers, Joint Controllers and Processors
5.1.3. Forthcoming Regulatory Framework for Artificial Intelligence
5.2. Principles Relating to the Processing of Personal Data
5.2.1. Lawfulness, Fairness and Transparency and Purpose Limitation
5.2.2. Data Minimisation, Accuracy and Limitation of Retention Period
5.2.3. Integrity and Confidentiality
5.2.4. Proactive Responsibility
5.3. Legitimation and Authorisation for Processing
5.3.1. Basis of Legitimacy
5.3.2. Authorisations for the Processing of Special Categories of Data
5.3.3. Data Communications
5.4. Individuals Rights
5.4.1. Transparency and Information
5.4.2. Access
5.4.3. Rectification and Deletion (Right to be Forgotten), Limitation and Portability
5.4.4. Opposition and Automated Individual Decisions
5.4.5. Limits to Rights
5.5. Risk Analysis and Management
5.5.1. Identification of Risks and Threats to the Rights and Freedoms of Individuals
5.5.2. Risk Assessment
5.5.3. Risk Management Plan
5.6. Proactive Accountability Measures
5.6.1. Identifying Techniques to Ensure and Accredit Compliance
5.6.2. Organizational measures
5.6.3. Technical Measures
5.6.4. Management of Personal Data Security Breaches
5.6.5. The Register of Processing Activities
5.7. Data Protection Impact Assessment (DPIA)
5.7.1. Activities Requiring PCIA
5.7.2. Evaluation Methodology
5.7.3. Identification of Risks, Threats and Consultation with the Control Authority
5.8. Contractual Regulation: persons responsible, persons in charge and other subjects
5.8.1. Data Protection Contracts
5.8.2. Attribution of Responsibilities
5.8.3. Contracts between Co-Responsible Parties
5.9. International Data Transfers
5.9.1. Definition and Safeguards to be Adopted
5.9.2. Standard Contractual Clauses
5.9.3. Other Instruments to Regulate Transfers
5.10. Violations and Penalties
5.10.1. Violations and Penalties
5.10.2. Graduation Criteria for Penalties
5.10.3. The Data Protection Officer
5.10.4. Functions of the Supervisory Authorities
Module 6. Scalable and Reliable Mass Data Usage Systems
6.1. Scalability, Reliability and Maintainability
6.1.1. Scales
6.1.2. Reliability
6.1.3. Maintainability
6.2. Data Models
6.2.1. Evolution of Data Models
6.2.2. Comparison of Relational Model with Document-Based NoSQL Model
6.2.3. Network Model
6.3. Data Storage and Retrieval Engines
6.3.1. Structured Log Storage
6.3.2. Storage in Segment Tables
6.3.3. Trees B
6.4. Services, Message Passing and Data Encoding Formats
6.4.1. Data Flow in REST Services
6.4.2. Data Flow in Message Passing
6.4.3. Message Sending Formats
6.5. Replication
6.5.1. CAP Theorem
6.5.2. Consistency Models
6.5.3. Models of Replication Based on Leader and Follower Concepts
6.6. Distributed Transactions
6.6.1. Atomic Operations
6.6.2. Distributed Transactions from Different Approaches Calvin, Spanner
6.6.3. Serialisability
6.7. Partitions
6.7.1. Types of Partitions
6.7.2. Indexes in Partitions
6.7.3. Partition Rebalancing
6.8. Batch Processing
6.8.1. Batch Processing
6.8.2. MapReduce
6.8.3. Post-MapReduce Approaches
6.9. Data Stream Processing
6.9.1. Messaging Systems
6.9.2. Persistence of Data Flows
6.9.3. Uses and Operations with Data Flows
6.10. Case Uses. Twitter, Facebook, Uber
6.10.1. Twitter: The Use of Caches
6.10.2. Facebook: Non-Relational Models
6.10.3. Uber: Different Models for Different Purposes
Module 7. System Administration for Distributed Deployments
7.1. Classic Administration. The Monolithic Model
7.1.1. Classical Aplications. The Monolithic Model
7.1.2. System Requirements for Monolithic Applications
7.1.3. The Administration of Monolithic Systems
7.1.4. Automation
7.2. Distributed Applications. The Microservice
7.2.1. Distributed Computing Paradigm
7.2.2. Microservices-Based Models
7.2.3. System Requirements for Distributed Models
7.2.4. Monolithic Applications vs. Distributed Applications
7.3. Tools for Resource Exploitation
7.3.1. “Iron” Management
7.3.2. Virtualisation
7.3.3. Emulation
7.3.4. Paravirtualization
7.4. IaaS, PaaS and SaaS Models
7.4.1. LaaS Model
7.4.2. PaaS Model
7.4.3. SaaS Model
7.4.4. Design Patterns
7.5. Containerisation
7.5.1. Virtualisation with Cogroups
7.5.2. Containers
7.5.3. From Application to Container
7.5.4. Container Orchestration
7.6. Clustering
7.6.1. High Performance and High Availability
7.6.2. High Availability Models
7.6.3. Cluster as SaaS Platform
7.6.4. Cluster Securitisation
7.7. Cloud Computing
7.7.1. Clusters vs Clouds
7.7.2. Types of Clouds
7.7.3. Cloud Service Models
7.7.4. Oversubscription
7.8. Monitoring and Testing
7.8.1. Types of Monitoring
7.8.2. Visualization
7.8.3. Infrastructure Tests
7.8.4. Chaos Engineering
7.9. Study Case: Kubernetes
7.9.1. Structure
7.9.2. Administration
7.9.3. Deployment of Services
7.9.4. Development of Services for K8S
7.10. Study Case: OpenStack
7.10.1. Structure
7.10.2. Administration
7.10.3. Deployment
7.10.4. Development of Services for OpenStack
Module 8. Internet of Things
8.1. Internet of Things (IoT)
8.1.1. The Internet of the Future
8.1.2. Internet of Things and Industrial Internet of Things
8.1.3. The Industrial Internet Consortium
8.2. Architecture of Reference
8.2.1. The Architecture of Reference
8.2.2. Layers and Components
8.3. IoT Devices
8.3.1. Classification
8.3.2. Components
8.3.3. Sensors and Actuators
8.4. Communication Protocols
8.4.1. Classification
8.4.2. OSI Model
8.4.3. Technologies
8.5. IoT and IIoT platforms
8.5.1. The IoT Platform
8.5.2. General Purpose Cloud Platforms
8.5.3. Industrial Platforms
8.5.4. Open Code Platforms
8.6. Data Management on IoT Platforms
8.6.1. Management Mechanisms
8.6.2. Open Data
8.6.3. Exchange of Data
8.6.4. Data Visualization
8.7. IoT Security
8.7.1. Security Requirements
8.7.2. Security Areas
8.7.3. Security Strategies
8.7.4. IIoT Security
8.8. IoT Systems Application Areas
8.8.1. Intelligent Cities
8.8.2. Health and Fitness
8.8.3. Smart Home
8.8.4. Other Applications
8.9. Application of IIoT to Different Industrial Sectors
8.9.1. Fabrication
8.9.2. Transport
8.9.3. Energy
8.9.4. Agriculture and Livestock
8.9.5. Other Sectors
8.10. Integration of IIoT in the Industry 4.0 Model
8.10.1. IoRT (Internet of Robotics Things)
8.10.2. 3D Additive Manufacturing
8.10.3. Big Data Analytics
Module 9. Project Management and Agile Methodologies
9.1. Project Management
9.1.1. The Project
9.1.2. Phases of a Project
9.1.3. Project Management
9.2. PMI Methodology for Project Management
9.2.1. PMI (Project Management Institute)
9.2.2. PMBOK
9.2.3. Difference between Project, Programme and Project Portfolio
9.2.4. Evolution of Organisations Working with Projects
9.2.5. Process Assets in Organisations
9.3. PMI Methodology for Project Management: Process
9.3.1. Groups of Processes
9.3.2. Knowledge Areas
9.3.3. Process Matrix
9.4. Agile Methodologies for Project Management
9.4.1. VUCA Context (Volatility, Uncertainty, Complexity and Ambiguity)
9.4.2. Agile Values
9.4.3. Principles of the Agile Manifesto
9.5. Agile SCRUM Framework for Project Management
9.5.1. Scrum
9.5.2. The Pillars of the Scrum Methodology
9.5.3. The Values in Scrum
9.6. Agile SCRUM Framework for Project Management. Process
9.6.1. The Scrum Process
9.6.2. Typified Roles in a Scrum Process
9.6.3. The Ceremonies of Scrum
9.7. Agile SCRUM Framework for Project Management. Artefacts
9.7.1. Artefacts in the Scrum Process
9.7.2. The Scrum Team
9.7.3. Metrics for Evaluating the Performance of a Scrum Team
9.8. Agile KANBAN Framework for Project Management. Kanban Method
9.8.1. Kanban
9.8.2. Benefits of Kanban
9.8.3. Kanban Method Components
9.9. Agile KANBAN Framework for Project Management. Kanban Method Practices
9.9.1. The Values of Kanban
9.9.2. Principles of the Kanban Method
9.9.3. General Practices of the Kanban Method
9.9.4. Metrics for Kanban Performance Evaluation
9.10. Comparison: PMI, SCRUM y KANBAN
9.10.1. PMI – SCRUM
9.10.2. PMI – KANBAN
9.10.3. SCRUM – KANBAN
Module 10. Communication, Leadership and Team Management
10.1. Organisational Development in Business
10.1.1. Climate, Culture and Organisational Development in the Company
10.1.2. Human Capital Management
10.2. Direction Models Decision Making
10.2.1. Paradigm Shift in Management Models
10.2.2. Management Process of the Technology Company
10.2.3. Decision-Making. Planning Instruments
10.3. Leadership. Delegation and Empowerment
10.3.1. Leadership
10.3.2. Delegation and Empowerment
10.3.3. Performance Evaluation
10.4. Leadership. Knowledge and Talent Management
10.4.1. Talent Management in the Company
10.4.2. Engagement Management in the Company
10.4.3. Improving Communication in the Company
10.5. Coaching Applied to Business
10.5.1. Executive Coaching
10.5.2. Team Coaching
10.6. Mentoring Applied to Business
10.6.1. Mentor Profile
10.6.2. The 4 Processes of a Mentoring Programme
10.6.3. Tools and Techniques in a Mentoring Process
10.6.4. Benefits of Mentoring in the Business Environment
10.7. Team Management I. Interpersonal Relations
10.7.1. Interpersonal Relationships
10.7.1.1. Relational Styles: Focuses
10.7.1.2. Effective Meetings and Agreements in Difficult Situations
10.8. Team Management II. The Conflicts
10.8.1. The Conflicts
10.8.2. Preventing, Addressing and Resolving Conflict
10.8.2.1. Strategies to Prevent Conflict
10.8.2.2. Conflict Management. Basic Principles
10.8.2.3. Conflict Resolution Strategies
10.8.3. Stress and Work Motivation
10.9. Team Management III. Negotiation
10.9.1. Negotiation at the Managerial Level in Technology Companies
10.9.2. Styles of Negotiation
10.9.3. Negotiation Phases
10.9.3.1. Barriers to Overcome in Negotiations
10.10. Team Management IV. Negotiation Techniques
10.10.1. Negotiation Techniques and Strategies
10.10.1.1. Strategies and Main Types of Negotiation
10.10.1.2. Negotiation Tactics and Practical Issues
10.10.2. The Figure of the Negotiating Subject
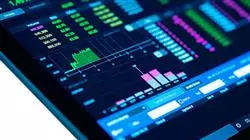
Make the most of this opportunity to surround yourself with expert professionals and learn from their work methodology"
Hybrid Professional Master's Degree in Corporate Technical Data Science Management
In an increasingly digitized world, data analysis has become a key factor for the success of companies. At TECH Global University, we present you our Hybrid Professional Master's Degree in Corporate Technical Data Science Management, a unique opportunity to acquire the necessary skills and lead the digital transformation in your organization. Our master's degree combines the best of two modalities: 100% online classes and 100% face-to-face practices. This will allow you to access a complete academic program from the comfort of your home, but you will also have the opportunity to do internships in specialized companies, applying your knowledge in real environments and enriching your experience. In this Master, you will learn the most advanced techniques of Data Science and its application in strategic decision making in the company. You will acquire skills in the use of programming tools and languages, data analysis, machine learning and information visualization. In addition, our hands-on approach will give you the opportunity to work on real projects and develop innovative solutions to current business challenges.
Study 100% online theory and 100% face-to-face your internship
At the end of the Master, you will be prepared to take on leadership roles in the area of Data Science, leading teams and contributing to the success of your organization. You will be able to identify business opportunities based on data analysis, make informed decisions and implement efficient strategies for the growth and competitiveness of your company. Do not miss the opportunity to become an expert in Data Science Technical Management in the company. Enroll in our Hybrid Professional Master's Degree in Corporate Technical Data Science Management at TECH Global University and take a step forward in your professional career - become the leader who drives digital transformation in your organization and achieve success in the business world!