University certificate
The world's largest faculty of engineering”
Description
Thanks to this Professional master’s degree, you will analyze how artificial intelligence influences pattern recognition in medical images”
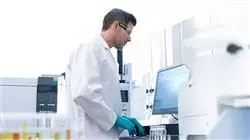
The Professional master’s degree in E-Health and Big Data aims to transmit the multiple benefits of technology in medicine. The concept of e-Health is defined by the World Health Organization (WHO) as: the cost-effective and safe use of information and communication technologies in support of health and public health related fields, including healthcare, health surveillance and health education, knowledge and research. Developments in this field make it possible, for example, to diagnose diseases thanks to hospital databases, or even to insert new 3D-printed limbs into human and animal bodies.
The imminent evolution of medicine requires highly qualified professionals who know how to respond to the needs of Industry 4.0. TECH aims to boost the careers of engineers who wish to delve into healthcare service and are interested in the simultaneous development of telemedicine. This Professional master’s degree addresses the theoretical and practical foundations of modern medicine to generate a global and deep vision of the new additions in biomedicine.
Students will not only delve into aspects of E-Health and Big Data, but will also learn about how the international healthcare system operates and is organized. Likewise, this program offers a focus on the entrepreneurship sector that positions engineering graduates as the target audience of this digital education, encouraging the creation of their own companies with the keys to business innovation.
TECH will participate in the expansion of these studies through a Professional master’s degree based on the knowledge of scientists in the field, especially those involved in artificial intelligence projects. The teaching staff will be available to students 24 hours a day and will monitor their studies. What is more, the 100% online format and the audiovisual content will facilitate student training and study
Distinguish yourself in a booming sector and join what is considered to be the technological solution of the future in medical development”
This Professional master’s degree in E-Health and Big Data contains the most complete and up-to-date program on the market. The most important features include:
- Practical cases presented by experts in Information and Communication Technology focused on the healthcare services
- The graphic, schematic, and practical contents with which they are created, provide scientific and practical information on the disciplines that are essential for professional practice
- Practical exercises where self-assessment can be used to improve learning
- Its special emphasis on innovative methodologies
- Theoretical lessons, questions to the expert, debate forums on controversial topics, and individual reflection assignments
- Content that is accessible from any fixed or portable device with an Internet connection
Join the change in modern medicine by applying artificial intelligence and the internet of things (IoT) to telemedicine”
The program’s teaching staff includes professionals from the sector who contribute their work experience to this educational program, as well as renowned specialists from leading societies and prestigious universities.
Its multimedia content, developed with the latest educational technology, will allow the professional a situated and contextual learning, that is, a simulated environment that will provide an immersive education programmed to prepare in real situations.
This program is designed around Problem-Based Learning, whereby the professional must try to solve the different professional practice situations that arise during the course. For this purpose, the student will be assisted by an innovative interactive video system created by renowned and experienced experts.
Boost your career projection thanks to bioinformatics computing and Big Data techniques"
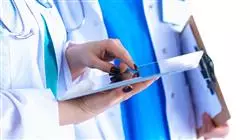
Update your biomedical knowledge thanks to new tools used in clinical health management"
Syllabus
The contents of this program have been carefully designed by a team of professionals who have contributed their knowledge based on the branches of health sciences and communication. Thanks to their contribution, the student will understand in a simple and educational way the subject matter, which transcends from clinical medicine to business innovation and entrepreneurship in e-Health. To that end, TECH applies the Relearning methodology, which offers guarantees of study by allowing for a gradual assimilation of theoretical and practical content.
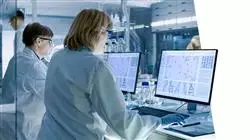
A program developed by experts in health sciences who provide quality content to transfer the appropriate knowledge”
Module 1. Molecular Medicine and Pathology Diagnosis
1.1. Molecular Medicine
1.1.1. Cellular and Molecular Biology. Cell Injury and Cell Death. Aging
1.1.2. Diseases Caused by Microorganisms and Host Defence
1.1.3. Autoimmune Diseases
1.1.4. Toxicological Diseases
1.1.5. Hypoxia Diseases
1.1.6. Diseases related to the Environment
1.1.7. Genetic Diseases and Epigenetics
1.1.8. Oncological Diseases
1.2. Circulatory System
1.2.1. Anatomy and Function
1.2.2. Myocardial Diseases and Heart Failure
1.2.3. Cardiac Rhythm Diseases
1.2.4. Valvular and Pericardial Diseases
1.2.5. Atherosclerosis, Arteriosclerosis and Arterial Hypertension
1.2.6. Peripheral Arterial and Venous Disease
1.2.7. Lymphatic Disease (Greatly Overlooked)
1.3. Respiratory Diseases
1.3.1. Anatomy and Function
1.3.2. Acute and Chronic Obstructive Pulmonary Diseases
1.3.3. Pleural and Mediastinal Diseases
1.3.4. Infectious Diseases of the Pulmonary Parenchyma and Bronchi
1.3.5. Pulmonary Circulation Diseases
1.4. Digestive System Diseases
1.4.1. Anatomy and Function
1.4.2. Digestive System, Nutrition and Hydroelectrolyte Exchange
1.4.3. Gastroesophageal Diseases
1.4.4. Gastrointestinal Infectious Diseases
1.4.5. Liver and Biliary Tract Diseases
1.4.6. Pancreatic Diseases
1.4.7. Colon Diseases
1.5. Renal and Urinary Tract Diseases
1.5.1. Anatomy and Function
1.5.2. Renal Insufficiency (Prerenal, Renal and Postrenal), How They Are Triggered
1.5.3. Obstructive Urinary Tract Diseases
1.5.4. Sphincteric Insufficiency in the Urinary Tract
1.5.5. Nephrotic Syndrome and Nephritic Syndrome
1.6. Endocrine System Diseases
1.6.1. Anatomy and Function
1.6.2. The Menstrual Cycle and Associated Conditions
1.6.3. Thyroid Disease
1.6.4. Adrenal Insufficiency
1.6.5. Disorders of Sexual Differentiation
1.6.6. Hypothalamic-Pituitary Axis, Calcium Metabolism, Vitamin D and Effects on Growth and Skeleton
1.7. Metabolism and Nutrition
1.7.1. Essential and Non-Essential Nutrients: Clarifying Definitions
1.7.2. Carbohydrate Metabolism and Alterations
1.7.3. Protein Metabolism and Alterations
1.7.4. Lipids Metabolism and Alterations
1.7.5. Iron Metabolism and Alterations
1.7.6. Disorders of Acid-Base Balance
1.7.7. Sodium and Potassium Metabolism and Alterations
1.7.8. Nutritional Diseases (Hypercaloric and Hypocaloric)
1.8. Hematologic Diseases
1.8.1. Anatomy and Function
1.8.2. Red Blood Cell Disorders
1.8.3. Diseases of White Blood Cells, Lymph Nodes and Spleen
1.8.4. Hemostasis and Bleeding Diseases
1.9. Musculoskeletal System Diseases
1.9.1. Anatomy and Function
1.9.2. Joints: Types and Function
1.9.3. Bone Regeneration
1.9.4. Normal and Pathological Skeletal System Development
1.9.5. Deformities of the Upper and Lower Limbs
1.9.6. Joint Pathology, Cartilage, and Synovial Fluid Analysis
1.9.7. Joint Diseases with Immunologic Origin
1.10. Nervous System Diseases
1.10.1. Anatomy and Function
1.10.2. Central and Peripheral Nervous System Development
1.10.3. Development of the Spine and Components
1.10.4. Cerebellum and Proprioceptive Diseases
1.10.5. Brain Disorders (Central Nervous System)
1.10.6. Spinal Cord and Cerebrospinal Fluid Diseases
1.10.7. Stenotic Diseases of the Peripheral Nervous System
1.10.8. Infectious Diseases of the Central Nervous System
1.10.9. Cerebrovascular Disease (Stenotic and Hemorrhagic)
Module 2. Health System Management and Administration in Health Centers
2.1. Healthcare Systems
2.1.1. Healthcare Systems
2.1.2. Healthcare Systems according to the WHO
2.1.2. Healthcare Context
2.2. Healthcare Models I. Bismark Model vs. Beveridge Model
2.2.1. Bismark Model
2.2.2. Beveridge Model
2.2.3. Bismark Model vs. Beveridge Model
2.3. Healthcare Models II. Semashko, Private and Mixed Models
2.3.1. Semashko Model
2.3.2. Private Model
2.3.3. Mixed Models
2.4. The Healthcare Market
2.4.1. The Healthcare Market
2.4.2. Regulation and Limitations of the Healthcare Market
2.4.3. Payment Methods for Doctors and Hospitals
2.4.4. Clinical Engineers
2.5. Hospitals. Typology
2.5.1. Hospital Architecture
2.5.2. Types of Hospitals
2.5.3. Hospital Organization
2.6. Health Metrics
2.6.1. Mortality
2.6.2. Morbidity
2.6.3. Healthy Life Years
2.7. Health Resource Allocation Methods
2.7.1. Lineal Programming
2.7.2. Maximization Models
2.7.3. Minimization Models
2.8. Measuring Healthcare Productivity
2.8.1. Measuring Health Productivity
2.8.2. Productivity Ratios
2.8.3. Input Adjustment
2.8.4. Output Adjustment
2.9. Health Process Improvement
2.9.1. Lean Management Process
2.9.2. Work Simplification Tools
2.9.3. Troubleshooting Tools
2.10. Healthcare Project Management
2.10.1. The Role Played by Project Managers
2.10.2. Team and Project Management Tools
2.10.3. Schedule and Time Management
Module 3. Research in Health Sciences
3.1. Scientific Research I. The Scientific Method
3.1.1. Scientific Research
3.1.2. Research in Health Sciences
3.1.3. The Scientific Method
3.2. Scientific Research II. Typology
3.2.1. Basic Research
3.2.2. Clinical Research
3.2.3. Translational Research
3.3. Evidence-Based Medicine
3.3.1. Evidence-Based Medicine
3.3.2. Principles of Evidence-Based Medicine
3.3.3. Methodology of Evidence-Based Medicine
3.4. Ethics and Legislation in Scientific Research. Declaration of Helsinki
3.4.1. The Ethics Committee
3.4.2. Declaration of Helsinki
3.4.3. Ethics in Health Sciences
3.5. Scientific Research Results
3.5.1. Methods
3.5.2. Rigor and Statistical Power
3.5.3. Scientific Results Validity
3.6. Public Communication
3.6.1. Scientific Societies
3.6.2. Scientific Conferences
3.6.3. Communication Structures
3.7. Funding in Scientific Research
3.7.1. Structure in Scientific Projects
3.7.2. Public Financing
3.7.3. Private and Industrial Funding
3.8. Scientific Resources in Literature Searching. Health Sciences Databases I
3.8.1. PubMed-Medline
3.8.2. Embase
3.8.3. WOS and JCR
3.8.4. Scopus and Scimago
3.8.5. Micromedex
3.8.6. MEDES
3.8.7. IBECS
3.8.8. LILACS
3.8.9. BDENF
3.8.10. Cuidatge
3.8.11. CINAHL
3.8.12. Cuiden Plus
3.8.13. Enfispo
3.8.14. NCBI (OMIM, TOXNET) and NIH (National Cancer Institute) Databases
3.9. Scientific Resources in Literature Searching. Health Sciences Databases II
3.9.1. NARIC - Rehabdata
3.9.2. PEDro
3.9.3. ASABE: Technical Library
3.9.4. CAB Abstracts
3.9.5. Centre for Reviews and Dissemination (CRD) Databases:
3.9.6. Biomed Central BMC
3.9.7. ClinicalTrials.gov
3.9.8. Clinical Trials Register
3.9.9. DOAJ- Directory of Open Access Journals
3.9.10. PROSPERO (International Register of Systemic Review)
3.9.11. TRIP
3.9.12. LILACS
3.9.13. NIH. Medical Library
3.9.14. Medline Plus
3.9.15. OPS
3.10. Scientific Resources in Literature Searching III. Search Engines and Platforms
3.10.1. Search Engines and Multisearch Engines
3.10.1.1. Findr
3.10.1.2. Dimensions
3.10.1.3. Google Scholar
3.10.1.4. Microsoft Academic
3.10.2. WHO International Clinical Trials Registration Platform (ICTRP)
3.10.2.1. PubMed Central PMC
3.10.2.2. Open Science Collector (RECOLECTA)
3.10.2.3. Zenodo
3.10.3. Doctoral Thesis Search Engines
3.10.3.1. DART-Europe
3.10.3.2. Dialnet
3.10.3.3. OATD (Open Access Theses and Dissertations)
3.10.3.4. TDR (Doctoral Theses Online)
3.10.3.5. TESEO
3.10.4. Bibliography Managers
3.10.4.1. Endnote Online
3.10.4.2. Mendeley
3.10.4.3. Zotero
3.10.4.4. Citeulike
3.10.4.5. Refworks
3.10.5. Digital Social Networks for Researchers
3.10.5.1. Scielo
3.10.5.2. Dialnet
3.10.5.3. Free Medical Journals
3.10.5.4. DOAJ
3.10.5.5. Open Science Directory
3.10.5.6. Redalyc
3.10.5.7. Academia.edu
3.10.5.8. Mendeley
3.10.5.9. ResearchGate
3.10.6. Social Web 2.0 Resources
3.10.6.1. Delicious
3.10.6.2. SlideShare
3.10.6.3. YouTube
3.10.6.4. Twitter
3.10.6.5. Health Science Blogs
3.10.6.6. Facebook
3.10.6.7. Evernote
3.10.6.8. Dropbox
3.10.6.9. Google Drive
3.10.7. Scientific Journal Publishers and Aggregators Portals
3.10.7.1. Science Direct
3.10.7.2. Ovid
3.10.7.3. Springer
3.10.7.4. Wiley
3.10.7.5. Proquest
3.10.7.6. Ebsco
3.10.7.7. BioMed Central
Module 4. Techniques, Recognition and Intervention using Biomedical Imaging
4.1. Medical Imaging
4.1.1. Modalities in Medical Imaging
4.1.2. Objectives in Medical Imaging Systems
4.1 3. Medical Imaging Storage Systems
4.2. Radiology
4.2.1. Imaging Method
4.2.2. Radiology Interpretation
4.2.3. Clinical Applications
4.3. Computed Tomography (CT)
4.3.1. Principle of Operation
4.3.2. Image Generation and Acquisition
4.3.3. Computerized Tomography. Typology
4.3.4. Clinical Applications
4.4. Magnetic Resonance Imaging (MRI)
4.4.1. Principle of Operation
4.4.2. Image Generation and Acquisition
4.4.3. Clinical Applications
4.5. Ultrasound: Ultrasound and Doppler Sonography
4.5.1. Principle of Operation
4.5.2. Image Generation and Acquisition
4.5.3. Typology
4.5.4. Clinical Applications
4.6. Nuclear Medicine
4.6.1. Physiological Basis in Nuclear Studies. Radiopharmaceuticals and Nuclear Medicine
4.6.2. Image Generation and Acquisition
4.6.3. Types of Tests
4.6.3.1. Gammagraphy
4.6.3.2. SPECT
4.6.3.3. PET
4.6.3.4. Clinical Applications
4.7. Image-Guided Interventions
4.7.1. Interventional Radiology
4.7.2. Interventional Radiology Objectives
4.7.3. Procedures
4.7.4. Advantages and Disadvantages
4.8. Image Quality
4.8.1. Technique
4.8.2. Contrast
4.8.3. Resolution
4.8.4. Noise
4.8.5. Distortion and Artifacts
4.9. Medical Imaging Tests. Biomedicine
4.9.1. Creating 3D Images
4.9.2. Biomodels
4.9.2.1. DICOM Standard
4.9.2.2. Clinical Applications
4.10. Radiological Protection
4.10.1. European Legislation Applicable to Radiology Services
4.10.2. Safety and Action Protocols
4.10.3. Radiological Waste Management
4.10.4. Radiological Protection
4.10.5. Care and Characteristics of Rooms
Module 5. Computation in Bioinformatics
5.1. Central Tenet in Bioinformatics and Computing. Current State
5.1.1. The Ideal Application in Bioinformatics
5.1.2. Parallel Developments in Molecular Biology and Computing
5.1.3. Dogma in Biology and Information Theory
5.1.4. Information Flows
5.2. Databases for Bioinformatics Computing
5.2.1. Database
5.2.2. Data management
5.2.3. Data Life Cycle in Bioinformatics
5.2.3.1. Use
5.2.3.2. Modifications
5.2.3.3. Archive
5.2.3.4. Reuse
5.2.3.5. Discarded
5.2.4. Database Technology in Bioinformatics
5.2.4.1. Architecture
5.2.4.2. Database Management
5.2.5. Interfaces for Bioinformatics Databases
5.3. Networks for Bioinformatics Computing
5.3.1. Communication Models. LAN, WAN, MAN and PAN Networks
5.3.2. Protocols and Data Transmission
5.3.3. Network Topologies
5.3.4. Datacenter Hardware for Computing
5.3.5. Security, Management and Implementation
5.4. Search Engines in Bioinformatics
5.4.1. Search Engines in Bioinformatics
5.4.2. Search Engine Processes and Technologies in Bioinformatics
5.4.3. Computational Models: Search and Approximation Algorithms
5.5. Data Display in Bioinformatics
5.5.1. Displaying Biological Sequences
5.5.2. Displaying Biological Structures
5.5.2.1. Visualization Tools
5.5.2.2. Rendering Tools
5.5.3. User Interface in Bioinformatics Applications
5.5.4. Information Architectures for Displays in Bioinformatics
5.6. Statistics for Computing
5.6.1. Statistical Concepts for Computing in Bioinformatics
5.6.2. Use Case: MARN Microarrays
5.6.3. Imperfect Data. Statistical Errors: Randomness, Approximation, Noise and Assumptions
5.6.4. Error Quantification: Precision and Sensitivity
5.6.5. Clustering and Classification
5.7. Data Mining
5.7.1. Mining and Data Computing Methods
5.7.2. Infrastructure for Data Mining and Computing
5.7.3. Pattern Discovery and Recognition
5.7.4. Machine Learning and New Tools
5.8. Genetic Pattern Matching
5.8.1. Genetic Pattern Matching
5.8.2. Computational Methods for Sequence Alignments
5.8.3. Pattern Matching Tools
5.9. Modelling and Simulation
5.9.1. Use in the Pharmaceutical Field: Drug Discovery
5.9.2. Protein Structure and Systems Biology
5.9.3. Available Tools and Future
5.10. Collaboration and Online Computing Projects
5.10.1. Grid Computing
5.10.2. Standards and Rules Uniformity, Consistency and Interoperability
5.10.3. Collaborative Computing Projects
Module 6. Biomedical Databases
6.1. Biomedical Databases
6.1.1. Biomedical Databases
6.1.2. Primary and Secondary Databases
6.1.3. Major Databases
6.2. DNA Databases
6.2.1. Genome Databases
6.2.2. Gene Databases
6.2.3. Mutations and Polymorphisms Databases
6.3. Protein Databases
6.3.1. Primary Sequence Databases
6.3.2. Secondary Sequence and Domain Databases
6.3.3. Macromolecular Structure Databases
6.4. Omics Projects Databases
6.4.1. Genomics Studies Databases
6.4.2. Transcriptomics Studies Databases
6.4.3. Proteomics Studies Databases
6.5. Genetic Diseases Databases. Personalized and Precision Medicine
6.5.1. Genetic Diseases Databases
6.5.2. Precision Medicine. The Need to Integrate Genetic Data
6.5.3. Extracting Data from OMIM
6.6. Self-Reported Patient Repositories
6.6.1. Secondary Data Use
6.6.2. Patients' Role in Deposited Data Management
6.6.3. Repositories of Self-Reported Questionnaires. Examples:
6.7. Elixir Open Databases
6.7.1. Elixir Open Databases
6.7.2. Databases Collected on the Elixir Platform
6.7.3. Criteria for Choosing between Databases
6.8. Adverse Drug Reactions (ADRs) Databases
6.8.1. Pharmacological Development Processes
6.8.2. Adverse Drug Reaction Reporting
6.8.3. Adverse Reaction Repositories at European and International Levels
6.9. Research Data Management Plans. Data to be Deposited in Public Databases
6.9.1. Data Management Plans
6.9.2. Data Custody in Research
6.9.3. Data Entry in Public Databases
6.10. Clinical Databases. Problems with Secondary Use of Health Data
6.10.1. Medical Record Repositories
6.10.2. Data Encryption
Module 7. Big Data in Medicine: Massive Medical Data Processing
7.1. Big Data in Biomedical Research
7.1.1. Data Generation in Biomedicine
7.1.2. High-Throughput Technology
7.1.3. Uses of High-Throughput Data. Hypotheses in the Age of Big Data
7.2. Data Pre-Processing in Big Data
7.2.1. Data Pre-Processing
7.2.2. Methods and Approaches
7.2.3. Problems with Data Pre-Processing in Big Data
7.3. Structural Genomics
7.3.1. Sequencing the Human Genome
7.3.2. Sequencing vs. Chips
7.3.3. Variant Discovery
7.4. Functional Genomics
7.4.1. Functional Notation
7.4.2. Mutation Risk Predictors
7.4.3. Association Studies in Genomics
7.5. Transcriptomics
7.5.1. Techniques to Obtain Massive Data in Transcriptomics: RNA-seq
7.5.2. Data Normalization in Transcriptomics
7.5.3. Differential Expression Studies
7.6. Interactomics and Epigenomics
7.6.1. The Role of Chromatin in Gene Expression
7.6.2. High-Throughput Studies in Interactomics
7.6.3. High-Throughput Studies in Epigenetics
7.7. Proteomics
7.7.1. Analysis of Mass Spectrometry Data
7.7.2. Post-Translational Modifications Study
7.7.3. Quantitative Proteomics
7.8. Enrichment and Clustering Techniques
7.8.1. Contextualizing Results
7.8.2. Clustering Algorithms in Omics Techniques
7.8.3. Repositories for Enrichment: Gene Ontology and KEGG
7.9. Applying Big Data to Public Health
7.9.1. Discovery of New Biomarkers and Therapeutic Targets
7.9.2. Risk Predictors
7.9.3. Personalized Medicine
7.10. Big Data Applied to Medicine
7.10.1. Potential for Diagnostic and Preventive Assistance
7.10.2. Use of Machine Learning Algorithms in Public Health
7.10.3. The Problem of Privacy
Module 8. Applications of Artificial Intelligence and the Internet of Things (IoT) in Telemedicine
8.1. E-Health Platforms. Personalizing Healthcare Services
8.1.1. E-Health Platform
8.1.2. Resources for E-Health Platforms
8.1.3. Digital Europe Program. Digital Europe-4-Health and Horizon Europe
8.2. Artificial Intelligence in Healthcare I: New Solutions in Computer Applications
8.2.1. Remote Analysis of Results
8.2.2. Chatbox
8.2.3. Prevention and Real-Time Monitoring
8.2.4. Preventive and Personalized Medicine in Oncology
8.3. Artificial Intelligence in Healthcare II: Monitoring and Ethical Challenges
8.3.1. Monitoring Patients with Reduced Mobility
8.3.2. Cardiac Monitoring, Diabetes, Asthma
8.3.3. Health and Wellness Apps
8.3.3.1. Heart Rate Monitors
8.3.3.2. Blood Pressure Bracelets
8.3.4. Ethical Use of AI in the Medical Field. Data Protection
8.4. Artificial Intelligence Algorithms for Image Processing
8.4.1. Artificial Intelligence Algorithms for Image Handling
8.4.2. Image Diagnosis and Monitoring in Telemedicine
8.4.2.1. Melanoma Diagnosis
8.4.3. Limitations and Challenges in Image Processing in Telemedicine
8.5. Application Acceleration using Graphics Processing Units (GPU) in Medicine
8.5.1. Program Parallelization
8.5.2. GPU Operations
8.5.3. Application Acceleration using GPU in Medicine
8.6. Natural Language Processing (NLP) in Telemedicine
8.6.1. Text Processing in the Medical Field. Methodology
8.6.2. Natural Language Processing in Therapy and Medical Records
8.6.3. Limitations and Challenges in Natural Language Processing in Telemedicine
8.7. The Internet of Things (IoT) in Telemedicine. Applications
8.7.1. Monitoring Vital Signs. Wearables
8.7.1.1. Blood Pressure, Temperature, and Heart Rate
8.7.2. The IoT and Cloud Technology
8.7.2.1. Data Transmission to the Cloud
8.7.3. Self-Service Terminals
8.8. IT in Patient Monitoring and Care
8.8.1. IoT Applications for Emergency Detection
8.8.2. The Internet of Things in Patient Rehabilitation
8.8.3. Artificial Intelligence Support in Victim Recognition and Rescue
8.9. Nanorobots. Typology
8.9.1. Nanotechnology
8.9.2. Types of Nanorobots
8.9.2.1. Assemblers. Applications
8.9.2.2. Self-Replicators. Applications
8.10. Artificial Intelligence in COVID-19 Control
8.10.1. COVID-19 and Telemedicine
8.10.2. Management and Communication of Breakthroughs and Outbreaks
8.10.3. Outbreak Prediction in Artificial Intelligence
Module 9. Telemedicine and Medical, Surgical and Biomechanical Devices
9.1. Telemedicine and Telehealth
9.1.1. Telemedicine as a Telehealth Service
9.1.2. Telemedicine
9.1.2.1. Telemedicine Objectives
9.1.2.2. Benefits and Limitations of Telemedicine
9.1.3. Digital Health. Technologies
9.2. Telemedicine Systems
9.2.1. Components in Telemedicine Systems
9.2.1.1. Personal
9.2.1.2. Technology
9.2.2. Information and Communication Technologies (ICT) in the Health Sector
9.2.2.1. T-Health
9.2.2.2. M-Health
9.2.2.3. M-Health
9.2.2.4. P-Health
9.2.3. Telemedicine Systems Assessment
9.3. Technology Infrastructure in Telemedicine
9.3.1. Public Switched Telephone Network (PSTN)
9.3.2. Satellite Networks
9.3.3. Integrated Services Digital Network (ISDN)
9.3.4. Wireless Technology
9.3.4.1. WAP. Wireless Application Protocol
9.3.4.2. Bluetooth
9.3.5. Microwave Connections
9.3.6. Asynchronous Transfer Mode (ATM)
9.4. Types of Telemedicine. Uses in Healthcare
9.4.1. Remote Patient Monitoring
9.4.2. Storage and Shipping Technologies
9.4.3. Interactive Telemedicine
9.5. Telemedicine: General Applications
9.5.1. Telecare
9.5.2. Telemonitoring
9.5.3. Telediagnostics
9.5.4. Tele-Education
9.5.5. Telemanagement
9.6. Telemedicine: Clinical Applications
9.6.1. Teleradiology
9.6.2. Teledermatology
9.6.3. Teleoncology
9.6.4. Telepsychiatry
9.6.5. Home Care (Telehomecare)
9.7. Smart Technologies and Care
9.7.1. Integrating Smart Homes
9.7.2. Digital Health to Improve Treatment
9.7.3. Telehealth Clothing Technology. “Smart Clothes”
9.8. Ethical and Legal Aspects of Telemedicine
9.8.1. Ethical Foundations
9.8.2. Common Regulatory Frameworks
9.8.4. ISO Standards
9.9. Telemedicine and Diagnostic, Surgical and Biomechanical Devices
9.9.1. Diagnostic Devices
9.9.2. Surgical Devices
9.9.2. Biomechanical Devices
9.10. Telemedicine and Medical Devices
9.10.1. Medical Devices
9.10.1.1. Mobile Medical Devices
9.10.1.2. Telemedicine Carts
9.10.1.3. Telemedicine Kiosks
9.10.1.4. Digital Cameras
9.10.1.5. Telemedicine Kit
9.10.1.6. Telemedicine Software
Module 10. Business Innovation and Entrepreneurship in E-Health
10.1. Entrepreneurship and Innovation
10.1.1. Innovation
10.1.2. Entrepreneurship
10.1.3. Startups
10.2. Entrepreneurship in E-Health
10.2.1. Innovative E-Health Market
10.2.2. Verticals in E-Health: M-Health
10.2.3. Tele-Health
10.3. Business Models I: First Stages in Entrepreneurship
10.3.1. Types of Business Models
10.3.1.1. Marketplaces
10.3.1.2. Digital Platforms
10.3.1.3. SaaS
10.3.2. Critical Elements in the Initial Phase. The Business Idea
10.3.3. Common Mistakes in the First Stages of Entrepreneurship
10.4. Business Models II: Business Model Canvas
10.4.1. Canvas Business Model
10.4.2. Value proposition
10.4.3. Key Activities and Resources
10.4.4. Customer Segments
10.4.5. Customer Relationships
10.4.6. Distribution Channels
10.4.7. Partnerships
10.4.7.1. Cost Structure and Revenue Streams
10.5. Business Models III: Lean Startup Methodology
10.5.1. Create
10.5.2. Validate
10.5.3. Measure
10.5.4. Decide
10.6. Business Models IV: External, Strategic and Regulatory Analysis
10.6.1. Red Ocean and Blue Ocean Strategies
10.6.2. Value Curves
10.6.3. Applicable E-Health Regulations
10.7. Successful E-Health Models I: Knowing Before Innovating
10.7.1. Analysis of Successful E-Health Companies
10.7.2. Analysis of Company X
10.7.3. Analysis of Company Y
10.7.4. Analysis of Company Z
10.8. Successful E-Health Models II: Listening before Innovating
10.8.1. Practical Interview: E-Health Startup CEO
10.8.2. Practical Interview: "Sector X" Startup CEO
10.8.3. Practical Interview: "Startup X" Technical Management
10.9. Entrepreneurial Environment and Funding
10.9.1. Entrepreneur Ecosystems in the Health Sector
10.9.2. Financing
10.9.3. Funding
10.10. Practical Tools in Entrepreneurship and Innovation
10.10.1. Open-Source Intelligence (OSINT)
10.10.2. Analysis
10.10.3. No-Code Tools in Entrepreneurship
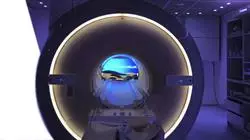
Make the most of this opportunity to learn about the latest advances in this subject to apply it to your daily practice"
Professional Master's Degree in E-Health and Big Data
E-healthy refers to the use of information and communication technologies (ICT) to improve the efficiency, effectiveness and quality of health services. This includes a set of applications and services that use technologies such as telemedicine, telehealth, remote patient monitoring and electronic medical record management. E-health also involves patients, allowing them remote access to health information and services, facilitating the management of their own pathologies.
On the other hand, big data refers to the set of massive data that is generated in different fields, and which is often too large and complex to be processed by traditional means. In healthcare, big data involves the collection, storage and analysis of large amounts of information about patients' medical records, medical test results and health-related behaviors.
At tech we have developed an intensive program in which we will train you on E-health and big data focused on educating students in the use of information technology, communication and the analysis of large amounts of data in the field of health. E-health focuses on the use of ICT to improve the efficiency and quality of healthcare services, while big data involves the analysis of large amounts of data to identify patterns and trends in healthcare. Both technologies have the potential to significantly improve healthcare and facilitate access to healthcare information and services.
Learn how to use monitoring and telemedicine technologies in healthcare, improving patient care. Know the principles of privacy and computer security that should be applied in the management of health data. Develop skills in the analysis and management of large amounts of data to improve the quality of patient care. Learn techniques and strategies for informed clinical decision making in the medical setting.