University certificate
The world's largest faculty of information technology”
Why study at TECH?
Combine Deep Learning with Computer Vision thanks to this Postgraduate diploma, which offers you all the latest developments in this booming technology"
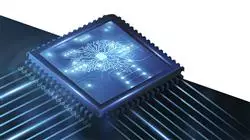
Artificial intelligence has revolutionized the technological landscape. Its principles are applied in many areas and are of great importance in fields such as healthcare, which takes advantage of this technology to improve diagnostic processes and treatments. Deep Learning is an essential area in this whole process, since it is what determines how the machine learning work will be carried out.
Therefore, by combining the potential of Deep Learning with another discipline such as computer vision, spectacular results can be obtained in all types of sectors. By combining these two specialties, a complete and in-depth visual data collection and reading is produced, perfecting the performance of complex technological tasks. This Postgraduate diploma, therefore, offers the computer scientists the possibility of accessing the latest innovations in this area, so that they can incorporate new knowledge about neural networks and their activation functions, convolutional neural networks and object detection, among others, into their work.
All of this is based on a 100% online teaching methodology that allows professionals to choose how, when and where to study, since it adapts to their personal circumstances. In addition, the computer scientist who completes this program will have access to the best multimedia content in the form of case studies, videos, master classes and multimedia summaries, among many other resources. In addition, the most experienced faculty will guide the entire process, ensuring that the professional receives the most up-to-date and practical knowledge.
Develop powerful computer vision tools from Deep Learning with this innovative and specialized educational program"
This Postgraduate diploma in Deep Learning Applied to Computer Vision contains the most complete and up-to-date educational program on the market. The most important features include:
- The development of case studies presented by experts in Deep Learning, computer science and computer vision
- The graphic, schematic, and practical contents with which they are created, provide scientific and practical information on the disciplines that are essential for professional practice
- Practical exercises where self assessment can be used to improve learning
- Its special emphasis on innovative methodologies
- Theoretical lessons, questions to the expert, debate forums on controversial topics, and individual reflection assignments
- Content that is accessible from any fixed or portable device with an Internet connection
You know that artificial intelligence is the present and the future. Do not miss this opportunity to learn about the latest advances in Deep Learning Applied to Computer Vision"
The program’s teaching staff includes professionals from the sector who contribute their work experience to this educational program, as well as renowned specialists from leading societies and prestigious universities.
The multimedia content, developed with the latest educational technology, will provide the professional with situated and contextual learning, i.e., a simulated environment that will provide immersive education programmed to learn in real situations.
This program is designed around Problem-Based Learning, whereby the professional must try to solve the different professional practice situations that arise during the academic year. For this purpose, the student will be assisted by an innovative interactive video system created by renowned and experienced experts.
This is the program you were looking for. Enroll now and progress professionally in the technology sector"
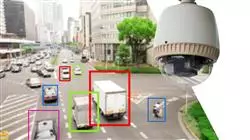
The best IT and technology companies are focusing all their efforts in these areas. Don't get left behind"
Syllabus
The contents of this Postgraduate diploma in Deep Learning Applied to Computer Vision have been carefully designed by leading specialists in artificial intelligence. For that reason, this knowledge is the newest and in-depth, and the computer scientist will have the opportunity to delve into the latest innovations in issues such as neural network evaluation metrics, types of CNN layers, learning with regularization or datasets, among many others.
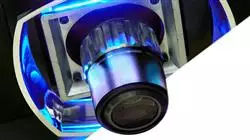
These contents will make you a great specialist in Deep Learning and computer vision"
Module 1. Deep Learning
1.1. Artificial Intelligence
1.1.1. Machine Learning
1.1.2. Deep Learning
1.1.3. The Explosion of Deep Learning Why Now?
1.2. Neural Networks
1.2.1. The Neural Network
1.2.2. Uses of Neural Networks
1.2.3. Linear Regression and Perceptron
1.2.4. Forward Propagation
1.2.5. Backpropagation
1.2.6. Feature Vectors
1.3. Loss Functions
1.3.1. Loss Functions
1.3.2. Types of Loss Functions
1.3.3. Choice of Loss Functions
1.4. Activation Functions
1.4.1. Activation Function
1.4.2. Linear Functions
1.4.3. Non-Linear Functions
1.4.4. Output vs. Hidden Layer Activation Functions
1.5. Regularization and Normalization
1.5.1. Regularization and Normalization
1.5.2. Overfitting and Data Augmentation
1.5.3. Regularization Methods: L1, L2 and Dropout
1.5.4. Normalization Methods: Batch, Weight, Layer
1.6. Optimization
1.6.1. Gradient Descent
1.6.2. Stochastic Gradient Descent
1.6.3. Mini Batch Gradient Descent
1.6.4. Momentum
1.6.5. Adam
1.7. Hyperparameter Tuning and Weights
1.7.1. Hyperparameters
1.7.2. Batch Size vs. Learning Rate vs. Step Decay
1.7.3. Weights
1.8. Evaluation Metrics of a Neural Network
1.8.1. Accuracy
1.8.2. Dice Coefficient
1.8.3. Sensitivity Vs. Specificity/Recall Vs. Precision
1.8.4. ROC Curve (AUC)
1.8.5. F1-Score
1.8.6. Matrix Confusion
1.8.7. Cross-Validation
1.9. Frameworks and Hardware
1.9.1. Tensor Flow
1.9.2. Pytorch
1.9.3. Caffe
1.9.4. Keras
1.9.5. Hardware for the Learning Phase
1.10. Creation of a Neural Network-Training and Validation.
1.10.1. Dataset
1.10.2. Network Construction
1.10.3. Training
1.10.4. Visualization of Results
Module 2. Convolutional Neural Networks and Image Classification
2.1. Convolutional Neural Networks
2.1.1. Introduction
2.1.2. Convolution
2.1.3. CNN Building Blocks
2.2. Types of CNN Layers
2.2.1. Convolutional
2.2.2. Activation
2.2.3. Batch Normalization
2.2.4. Polling
2.2.5. Fully Connected
2.3. Metrics
2.3.1. Matrix Confusion
2.3.2. Accuracy
2.3.3. Precision
2.3.4. Recall
2.3.5. F1 Score
2.3.6. ROC Curve
2.3.7. AUC
2.4. Architecture
2.4.1. AlexNet
2.4.2. VGG
2.4.3. Resnet
2.4.4. GoogleLeNet
2.5. Image Classification
2.5.1. Introduction
2.5.2. Analysis of Data
2.5.3. Data Preparation
2.5.4. Model Training
2.5.5. Model Validation
2.6. Practical Considerations for CNN Training
2.6.1. Optimizer Selection
2.6.2. Learning Rate Scheduler
2.6.3. Check Training from Pipeline
2.6.4. Training with Regularization
2.7. Best Practices in Deep Learning
2.7.1. Transfer Learning
2.7.2. Fine Tuning
2.7.3. Data Augmentation
2.8. Statistical Data Evaluation
2.8.1. Number of Datasets
2.8.2. Number of Labels
2.8.3. Number of Images
2.8.4. Data Balancing
2.9. Deployment
2.9.1. Model Saving
2.9.2. Onnx
2.9.3. Inference
2.10. Case Study: Image Classification
2.10.1. Data Analysis and Preparation
2.10.2. Testing the Training Pipeline
2.10.3. Model Training
2.10.4. Model Validation
Module 3. Object Detection
3.1. Object Detection and Tracking
3.1.1. Object Detection
3.1.2. Use Cases
3.1.3. Object Tracking
3.1.4. Case Uses
3.1.5. Occlusions, Rigid and Non-Rigid Poses
3.2. Evaluation Metrics
3.2.1. IOU - Intersection Over Union
3.2.2. Confidence Score
3.2.3. Recall
3.2.4. Precision
3.2.5. Recall–Precision Curve
3.2.6. Mean Average Precision (mAP)
3.3. Traditional Methods
3.3.1. Sliding Window
3.3.2. Viola Detector
3.3.3. HOG
3.3.4. Non-Maximal Suppression (NMS)
3.4. Datasets
3.4.1. Pascal VC
3.4.2. MS Coco
3.4.3. ImageNet (2014)
3.4.4. MOTA Challenge
3.5. Two Shot Object Detector
3.5.1. R-CNN
3.5.2. Fast R-CNN
3.5.3. Faster R-CNN
3.5.4. Mask R-CNN
3.6. Single Shot Object Detector
3.6.1. SSD
3.6.2. YOLO
3.6.3. RetinaNet
3.6.4. CenterNet
3.6.5. EfficientDet
3.7. Backbones
3.7.1. VGG
3.7.2. ResNet
3.7.3. Mobilenet
3.7.4. Shufflenet
3.7.5. Darknet
3.8. Object Tracking
3.8.1. Classical Approaches
3.8.2. Particulate Filters
3.8.3. Kalman
3.8.4. Sort Tracker
3.8.5. Deep Sort
3.9. Deployment
3.9.1. Computing Platform
3.9.2. Choice of Backbone
3.9.3. Choice of Framework
3.9.4. Model Optimization
3.9.5. Model Versioning
3.10. Study: Detection and Monitoring People
3.10.1. Detection of People
3.10.2. Monitoring of People
3.10.3. Re-Identification
3.10.4. Counting People in Crowds
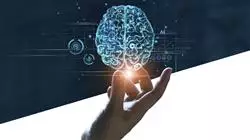
Don't wait any longer and access to the most specialized contents in these powerful branches of artificial intelligence"
Postgraduate Diploma in Deep Learning Applied to Computer Vision
.
Dive into the exciting world of deep learning applied to computer vision and become an expert in image analysis and visual recognition with TECH Global University's Postgraduate Diploma in Deep Learning Applied to Computer Vision academic program. Discover how artificial intelligence can revolutionize the way we perceive and understand the visual world around us. Our online classes give you the opportunity to acquire cutting-edge knowledge from anywhere and at any time. At our institution we value your time and convenience, that's why we have designed our program in an accessible and flexible way. With our online classes, you can learn at your own pace, without geographical restrictions or schedule limitations. The Postgraduate Diploma in Deep Learning Applied to Computer Vision program focuses on providing you with the necessary knowledge to apply deep learning techniques in the field of computer vision. You will learn to develop and train deep neural networks for the classification, detection and segmentation of objects in images. In addition, you will become familiar with state-of-the-art tools and frameworks used in the industry, which will allow you to tackle real problems with efficient solutions.
Learn all the tools in Deep Learning only at TECH
.
At the faculty of computer science we have a team of experts in artificial intelligence and computer vision that will guide you in your learning process. Through hands-on projects and interactive exercises, you will apply your knowledge in real cases and develop practical skills to solve complex challenges in the field of computer vision. Don't miss the opportunity to become a leader in the field of deep learning applied to computer vision. Join TECH Global University's Postgraduate Diploma in Deep Learning Applied to Computer Vision program and gain the skills you need to excel in the field of artificial intelligence and computer vision. Start your path to mastery in this exciting technology discipline today!